HLMD: Detecting Lateral Movement Using Heterogeneous Graph Model.
IEEE International Conference on Smart City(2023)
摘要
Lateral movement has been one of the most popular attacks in these years, which allows attackers to retain access secretly without being detected. Recent studies show that graph-based methods are effective for detecting this covert attack. However, existing models have two main limitations. First, their graph structures have poor types of nodes or edges that cannot represent the relation comprehensively. Second, these methods rely on a large number of labels, which is unsuitable in practice. To address these problems, we propose HLMD, a novel semi-supervised method based on a heterogeneous user-entity interaction graph, and use a graph embedding method to represent the feature of each node. HLMD fully characterizes various types of relationships between users and entities from network structure and historical interaction behaviors. For lateral movement detection, we use an RGCN (Relational Graph Convolution Network)-based semi-supervised anomaly detection model, which employs an unsupervised and supervised unified learning strategy to predict the abnormal nodes in a few labels. Our HLMD method performs better than the other baseline approaches on the LANL dataset with a high AUC of 0.92, a lower FPR of 1.5%, and an F1-score of 80.47%.
更多查看译文
关键词
Lateral movement,semi-supervised,anomaly detection,graph neural network
AI 理解论文
溯源树
样例
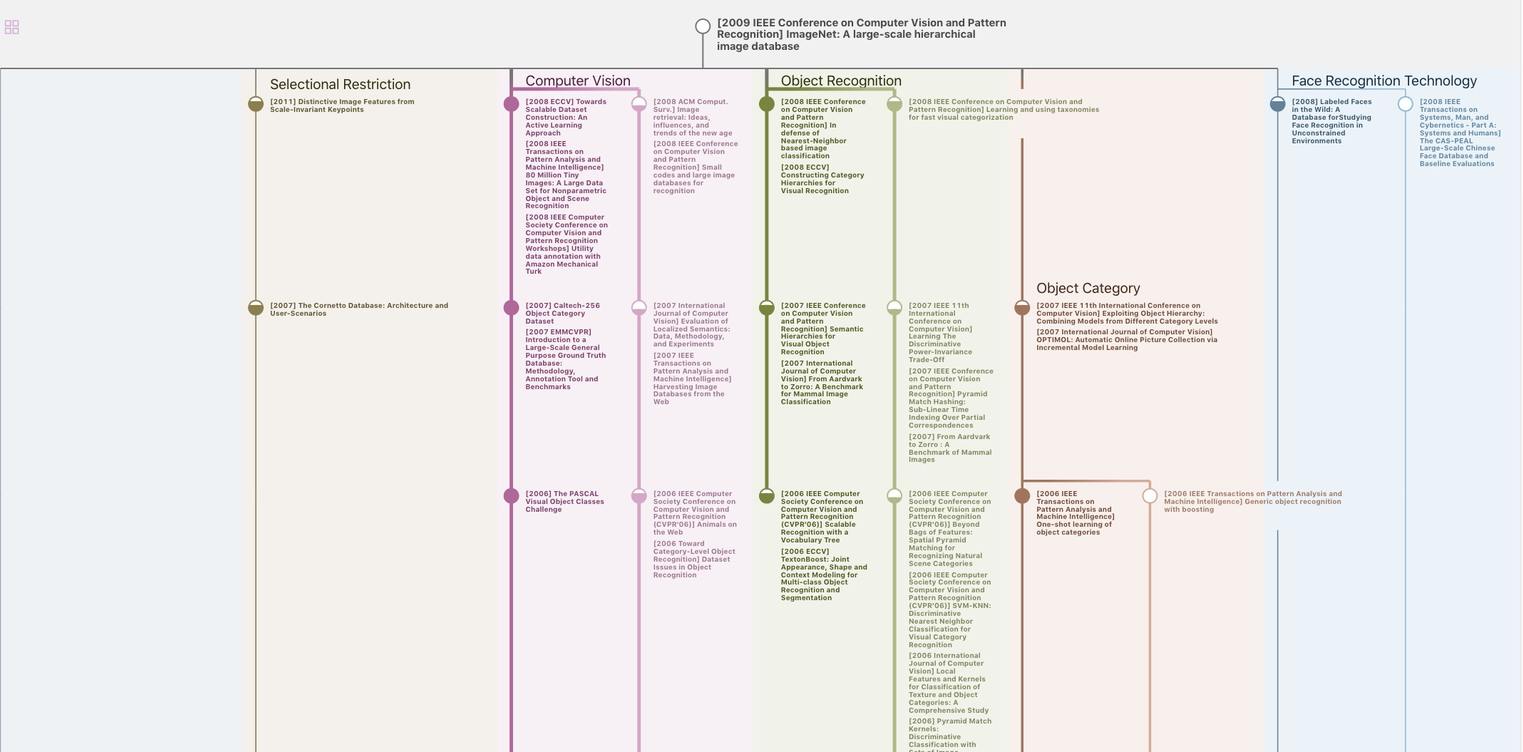
生成溯源树,研究论文发展脉络
Chat Paper
正在生成论文摘要