Better power by design: A quasirandomization strategy to boost power in repeated-measures experiments
crossref(2024)
摘要
Low power is a longstanding problem for laboratory studies in the cognitive sciences. We suggest that researchers using within-participant repeated measures designs can increase power by tapping into an unexpected source: variation in measurement over time. Such variation is widespread and reflects learning, fatigue, or fluctuations in attention. Given such variation, the current field-wide standard of randomizing the presentation order of conditions without constraint is subobtimal for power. We introduce a simple quasirandomization algorithm—bucket-constrained randomization (BCR)—that increases power by ensuring a more uniform distribution of conditions over time. We explored the performance of BCR across three scenarios of time-dependent error: exponential decay, Gaussian random walk, and pink noise. Monte Carlo simulations suggest that BCR can potentially boost power between about 4% and 30% while simultaneously keeping false positive rates close to the nominal level when time-dependent variation is absent.
更多查看译文
AI 理解论文
溯源树
样例
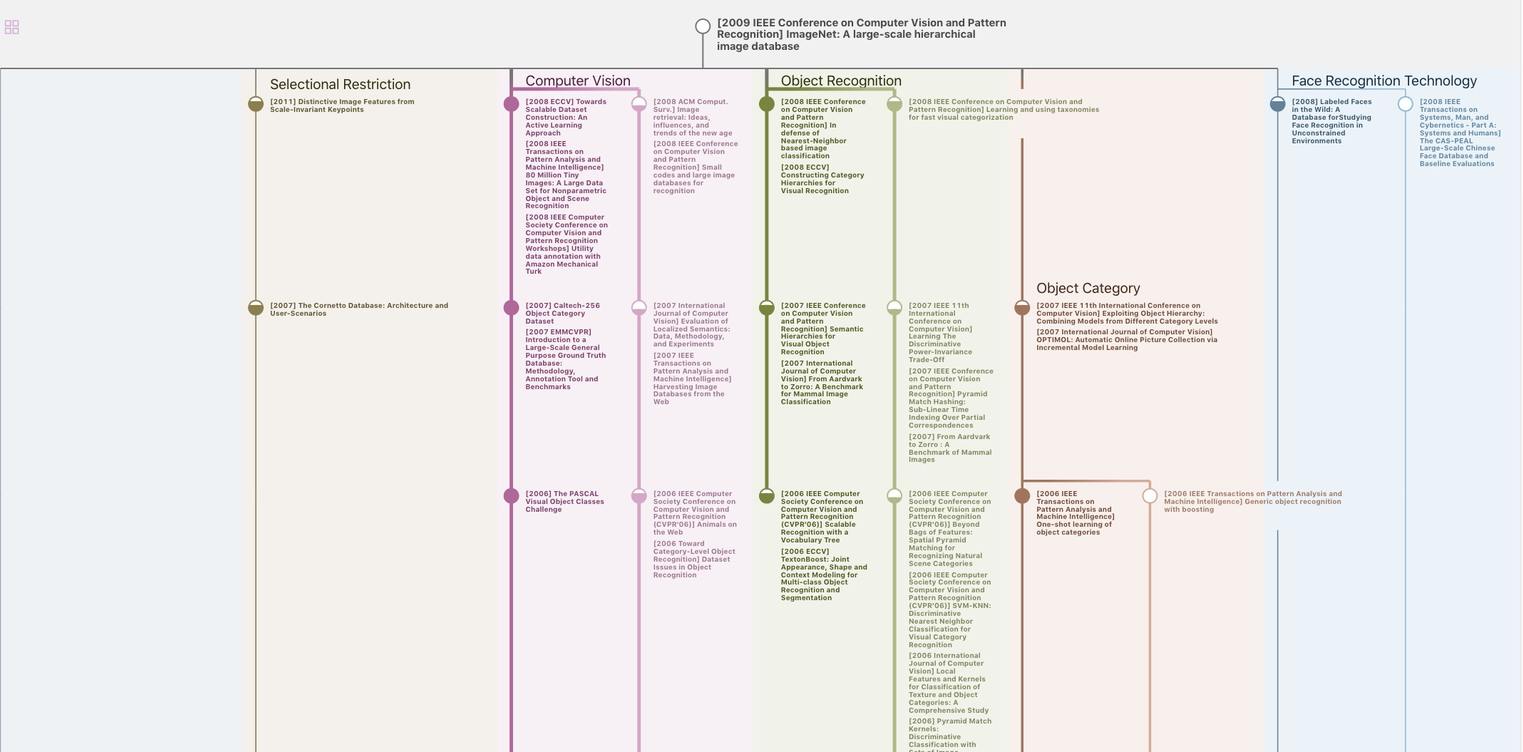
生成溯源树,研究论文发展脉络
Chat Paper
正在生成论文摘要