Machine Learning Analysis of Seismograms Reveals a Continuous Plumbing System Evolution Beneath the Klyuchevskoy Volcano in Kamchatka, Russia
JOURNAL OF GEOPHYSICAL RESEARCH-SOLID EARTH(2024)
摘要
Volcanoes produce a variety of seismic signals and, therefore, continuous seismograms provide crucial information for monitoring the state of a volcano. According to their source mechanism and signal properties, seismo-volcanic signals can be categorized into distinct classes, which works particularly well for short transients. Applying classification approaches to long-duration continuous signals containing volcanic tremors, characterized by varying signal characteristics, proves challenging due to the complex nature of these signals. That makes it difficult to attribute them to a single volcanic process and questions the feasibility of classification. In the present study, we consider the whole seismic time series as valuable information about the plumbing system (the combination of plumbing structure and activity distribution). The considered data are year-long seismograms recorded at individual stations near the Klyuchevskoy Volcanic Group (Kamchatka, Russia). With a scattering network and a Uniform Manifold Approximation and Projection (UMAP), we transform the continuous data into a two-dimensional representation (a seismogram atlas), which helps us to identify sudden and continuous changes in the signal properties. We observe an ever-changing seismic wavefield that we relate to a continuously evolving plumbing system. Through additional data, we can relate signal variations to various state changes of the volcano including transitions from deep to shallow activity, deep reactivation, weak signals during quiet times, and eruptive activity. The atlases serve as a visual tool for analyzing extensive seismic time series, allowing us to associate specific atlas areas, indicative of similar signal characteristics, with distinct volcanic activities and variations in the volcanic plumbing system. Volcanoes generate diverse seismic signals, and analyzing continuous seismograms can offer valuable insights into a volcano's behavior. However, classifying and cataloging these signals is challenging, particularly for long-period signals such as volcanic tremors with varying signal characteristics. In this study, we utilize seismic time series data from the Klyuchevskoy Volcanic Group in Russia, employing machine learning and signal processing methods to transform and represent the time series data. The resulting "seismogram atlas" helps identify changes in signal properties, revealing a dynamic seismic wavefield linked to the evolving plumbing system of the volcano. This approach provides a comprehensive understanding of the volcanic behavior in time by connecting signal variations to different volcanic states, such as shifts in activity depth, reactivation, and eruptive periods. These findings highlight the unique characteristics of continuous seismograms near the volcano, suggesting that there is valuable information in the complete seismic time series that conventional methods and event catalogs may miss. With machine learning, we analyze 1 year long seismic time series at individual stations at Klyuchevskoy volcano Continuous evolution of the signal characteristics over time reflects dynamic changes occurring in the volcano plumbing system Different episodes of volcanic activity are well distinguished on UMAP-based seismogram atlases
更多查看译文
关键词
volcano seismology,machine learning,time series representation
AI 理解论文
溯源树
样例
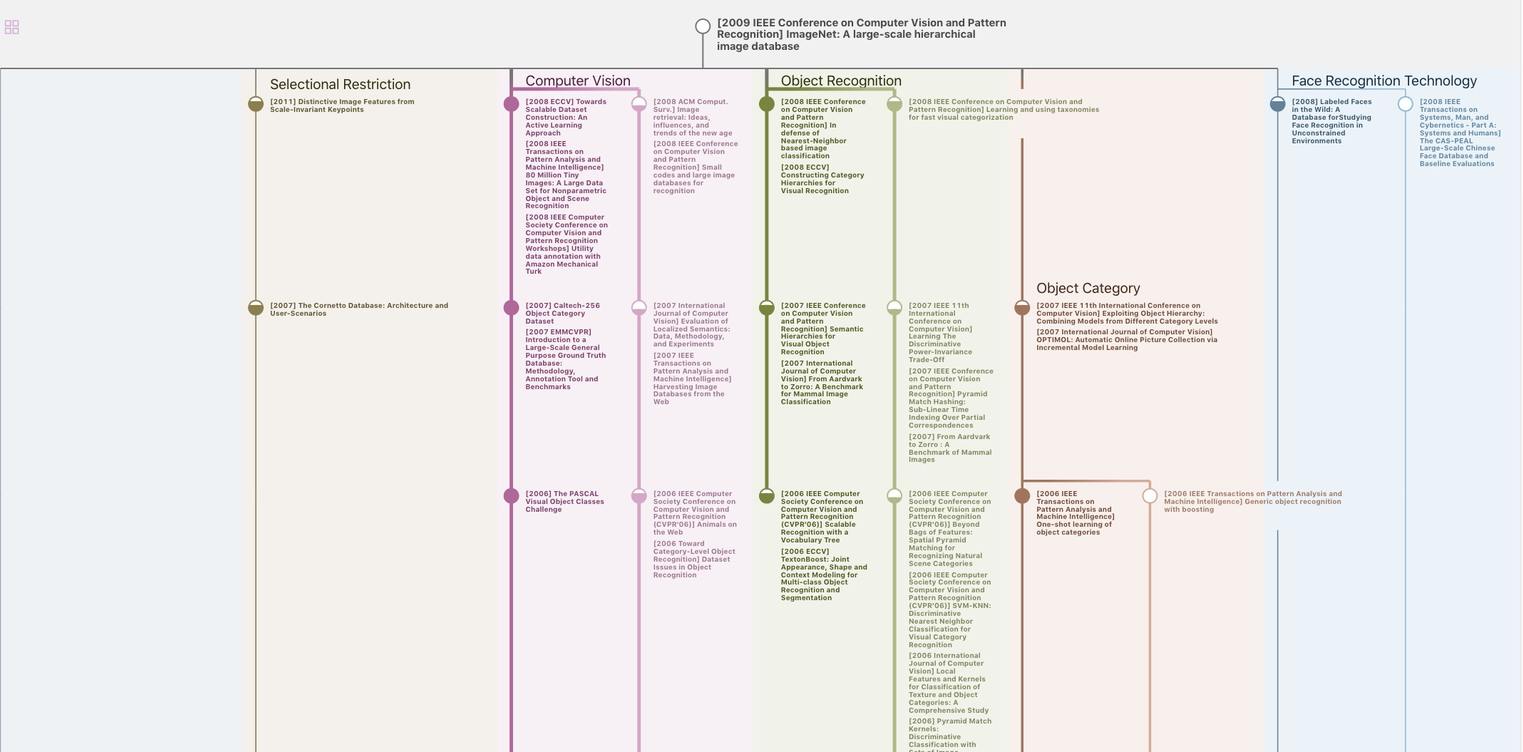
生成溯源树,研究论文发展脉络
Chat Paper
正在生成论文摘要