MindMap: Constructing Evidence Chains for Multi-Step Reasoning in Large Language Models
AAAI 2024(2024)
摘要
Large language models (LLMs) have demonstrated remarkable performance in various natural language processing tasks. However, they still face significant challenges in automated reasoning, particularly in scenarios involving multi-step reasoning. In this paper, we focus on the logical reasoning problem. The main task is to answer a question based on a set of available facts and rules. A lot of work has focused on guiding LLMs to think logically by generating reasoning paths, ignoring the structure among available facts. In this paper, we propose a simple approach MindMap by introducing evidence chains for supporting reasoning. An evidence chain refers to a set of facts that involve the same subject. In this way, we can organize related facts together to avoid missing important information. MindMap can be integrated with existing reasoning framework, such as Chain-of-Thought (CoT) and Selection-Inference (SI), by letting the model select relevant evidence chains instead of independent facts. The experimental results on the bAbI and ProofWriterOWA datasets demonstrate the effectiveness of MindMap.It can significantly improve CoT and SI, especially in multi-step reasoning tasks.
更多查看译文
关键词
NLP: Sentence-level Semantics, Textual Inference, etc.,NLP: (Large) Language Models
AI 理解论文
溯源树
样例
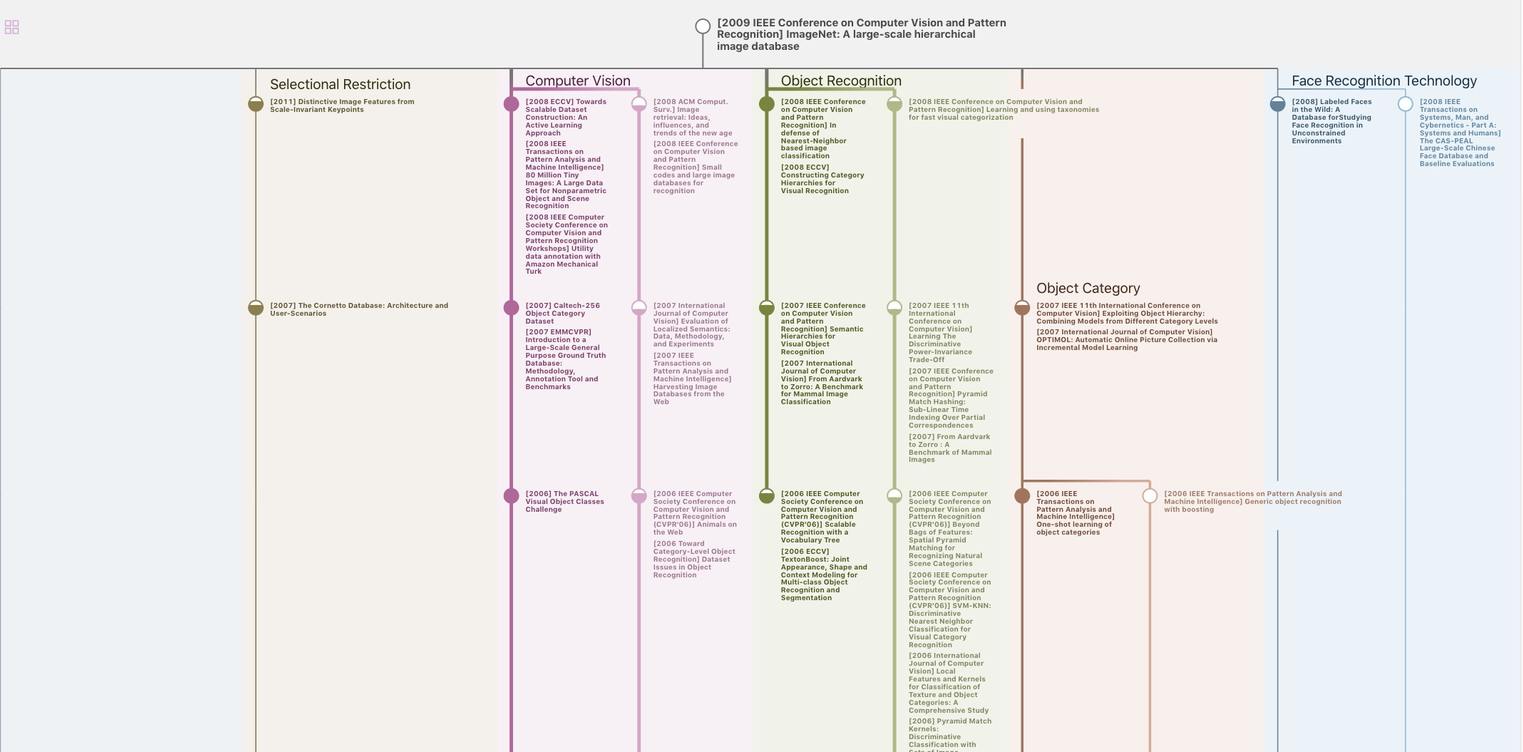
生成溯源树,研究论文发展脉络
Chat Paper
正在生成论文摘要