Attractive Solutions for Hilfer Fractional Neutral Stochastic Integro-Differential Equations with Almost Sectorial Operators
AIMS MATHEMATICS(2024)
关键词
Hilfer fractional derivative,stochastic evolution equations,neutral systems,infinite interval,attractivity
AI 理解论文
溯源树
样例
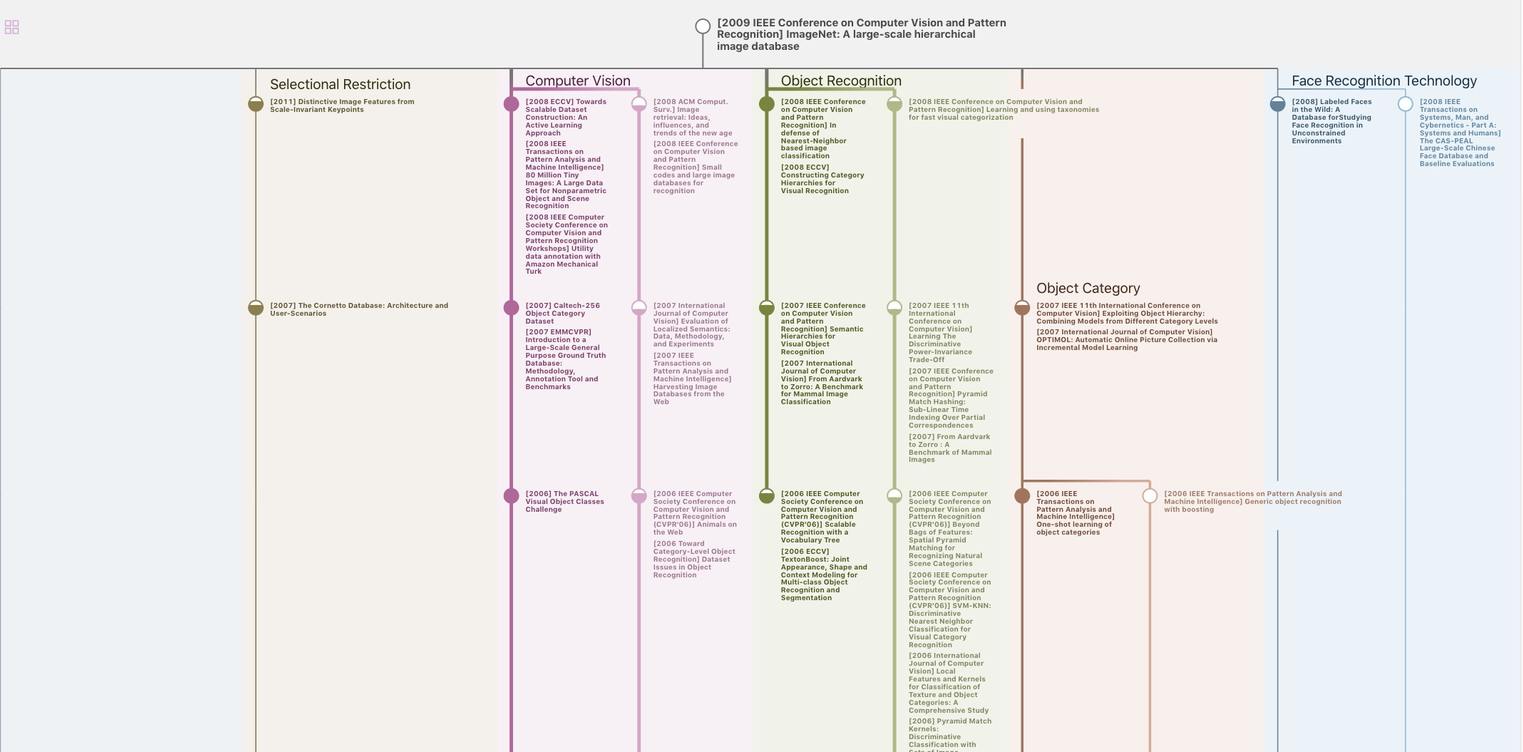
生成溯源树,研究论文发展脉络
Chat Paper
正在生成论文摘要