Application of graph neural networks to forecast urban flood events: the case study of the 2013 flood of the Bow River, Calgary, Canada
INTERNATIONAL JOURNAL OF RIVER BASIN MANAGEMENT(2024)
摘要
Mitigating the harmful effects of flooding is essential in the current climate change and urban growth scenario, where these natural phenomena are expected to occur more often. Addressing this problem, we present a novel graph-based forecasting model for predicting urban flooding and showcase its application in the Bow River in the City of Calgary, Alberta, Canada. The proposed model is based on graph theory and deep learning paradigms and was used to forecast flooding occurrences up to 24 h ahead. Our new approach developed the SAGE algorithm, a hybrid learning and planning approach that accurately predicted floodings for various analyzed forecasting horizons, increasing the forecasting performance by up to 44% compared to the persistence model. The SAGE model also returned superior or competitive results compared to other models in the literature. The superiority of the SAGE model was highlighted for longer forecast horizons of 24 h ahead, where it reached a maximum improvement of 79%, suggesting its superiority in capturing spatiotemporal information in the dataset. These results indicate that the SAGE approach is a cutting-edge tool for flooding forecasting, and it could be used to develop early flood warning systems to reduce potential flooding impacts.
更多查看译文
关键词
Flooding,Bow River,forecasting,machine learning,graph neural networks,deep learning
AI 理解论文
溯源树
样例
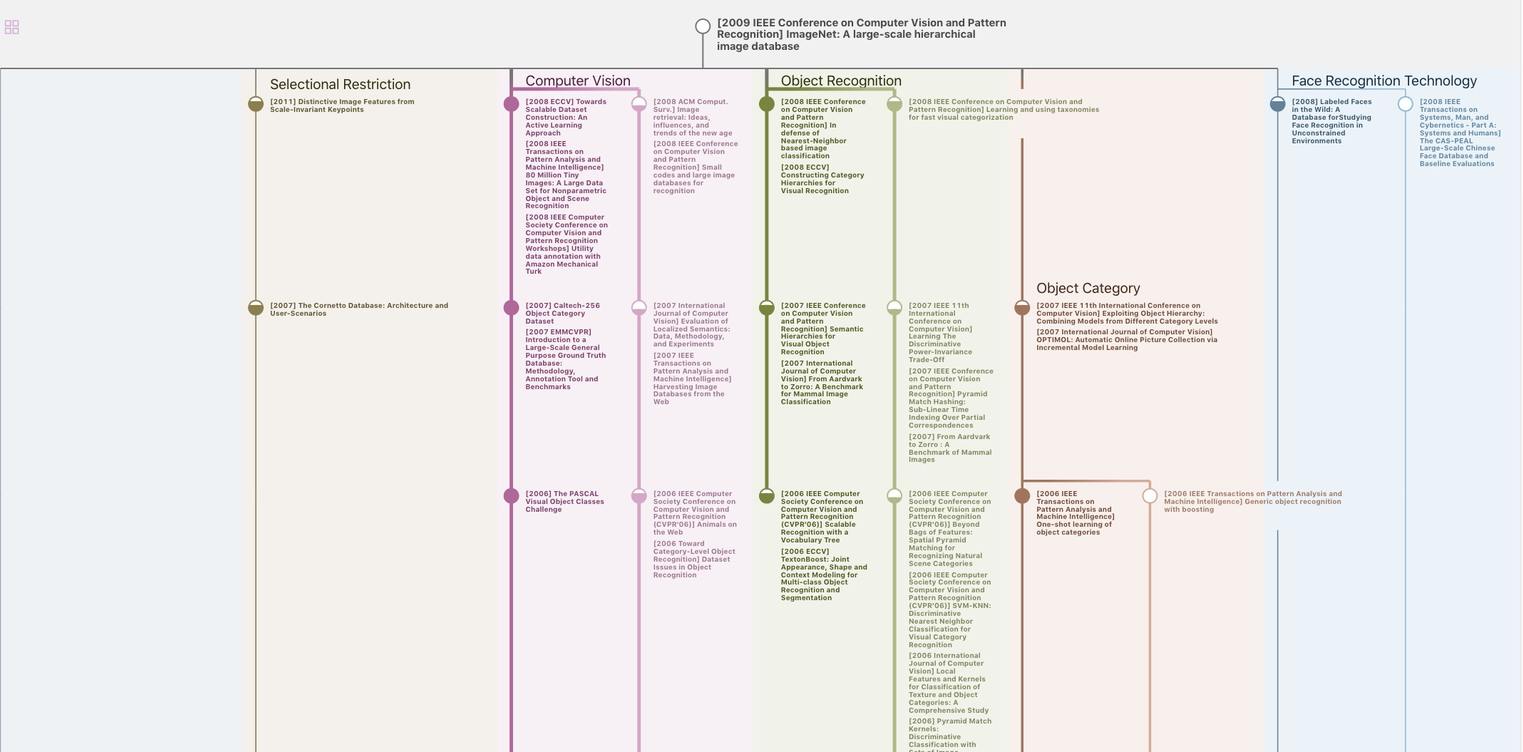
生成溯源树,研究论文发展脉络
Chat Paper
正在生成论文摘要