Enrichment on steps, not genes, improves inference of differentially expressed pathways
PLOS COMPUTATIONAL BIOLOGY(2024)
摘要
Enrichment analysis is frequently used in combination with differential expression data to investigate potential commonalities amongst lists of genes and generate hypotheses for further experiments. However, current enrichment analysis approaches on pathways ignore the functional relationships between genes in a pathway, particularly OR logic that occurs when a set of proteins can each individually perform the same step in a pathway. As a result, these approaches miss pathways with large or multiple sets because of an inflation of pathway size (when measured as the total gene count) relative to the number of steps. We address this problem by enriching on step-enabling entities in pathways. We treat sets of protein-coding genes as single entities, and we also weight sets to account for the number of genes in them using the multivariate Fisher's noncentral hypergeometric distribution. We then show three examples of pathways that are recovered with this method and find that the results have significant proportions of pathways not found in gene list enrichment analysis. Genome-scale experiments typically identify sets of genes which are primarily analyzed by enrichment analysis to identify relevant pathways that may be perturbed. Curated pathway models have rich structure that we believe can be exploited to get better results. Some pathway steps are enabled by sets of interchangeable genes which inflate the gene count of their respective pathways relative to the number of steps. We improve sensitivity towards these pathways in enrichment analysis by performing enrichment on steps. We then use this approach to identify pathways that would otherwise be missed in medically relevant datasets to gain new insights.
更多查看译文
AI 理解论文
溯源树
样例
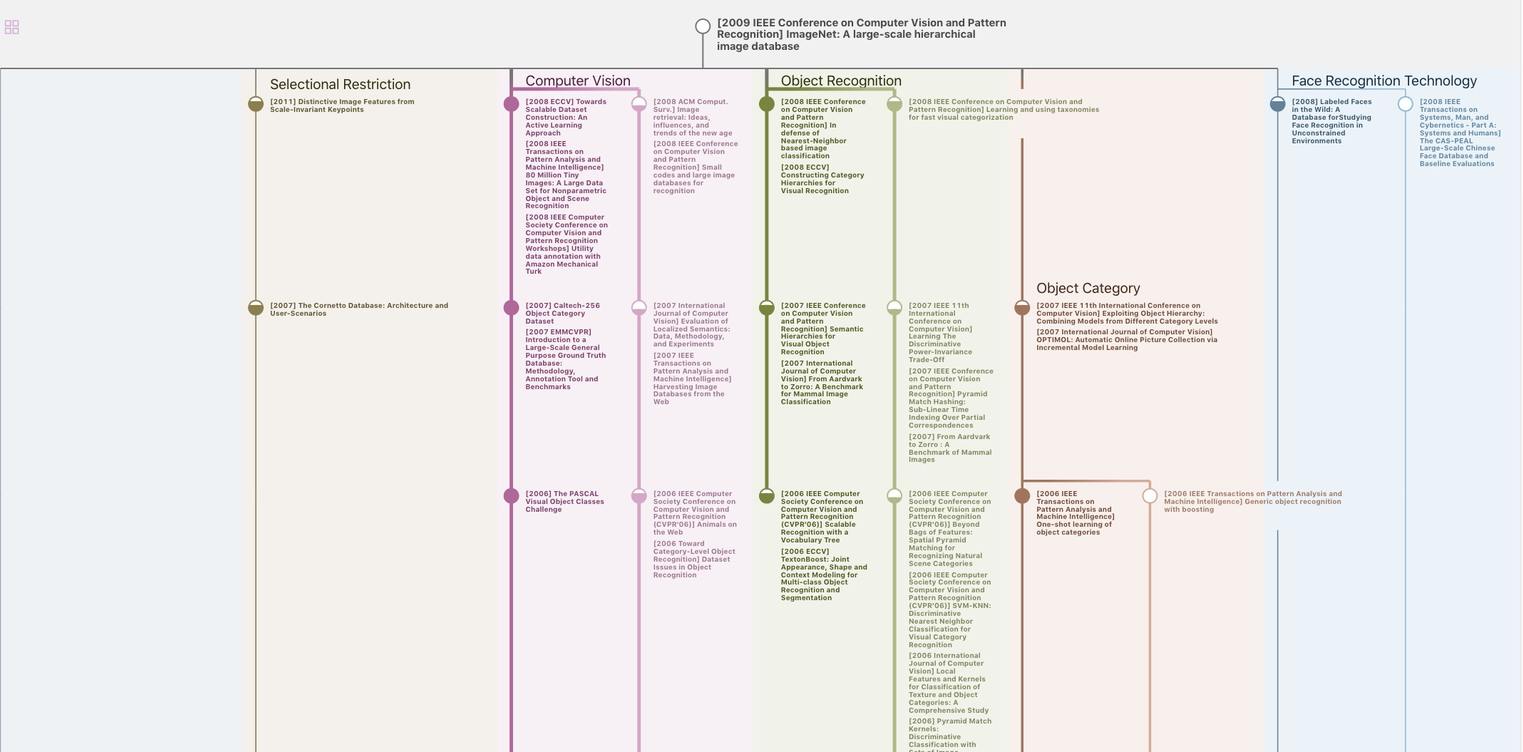
生成溯源树,研究论文发展脉络
Chat Paper
正在生成论文摘要