Outlier detection and removal in multifractal analysis of electrophysiological brain signals
crossref(2024)
摘要
Abstract The analysis of the scale invariant properties of brain activity has received growing attention in the last 15 years, especially in the context of the brain criticality hypothesis.Multifractal analysis (MFA) extends the ability of usual tools in brain criticality research (e.g. Detrended Fluctuation Analysis) to characterize scale invariance, by investigating the scaling of higher order statistical moments. However MFA is very sensitive to the presence of outliers in the data, for instance in the form of impulsive noise.In this work, we propose a novel algorithm for outlier detection and removal, which relies on the wavelet p-leader MFA formalism. The proposed approach consists in temporally segmenting the time-scale representation of the data (time series), and then tagging and removing the outlier segments from the final MFA estimation process. We first compare on on synthetic noisy mono- and multifractral time series the performances of our method with those of existing techniques such as the wavelet $p$-leader MFA formalism and a naive robust cumulant based approach. The proposed method is then evaluated on a single noisy resting-state Magnetoencephalography (MEG) recording of a healthy volunteer. Overall we show that in the presence of noise impulses, this method removes the existing bias with standard MFA tools (wavelet-leader based analysis) on both synthetic and neurophysiological data, with consistent findings across the two brain hemispheres in MEG.
更多查看译文
AI 理解论文
溯源树
样例
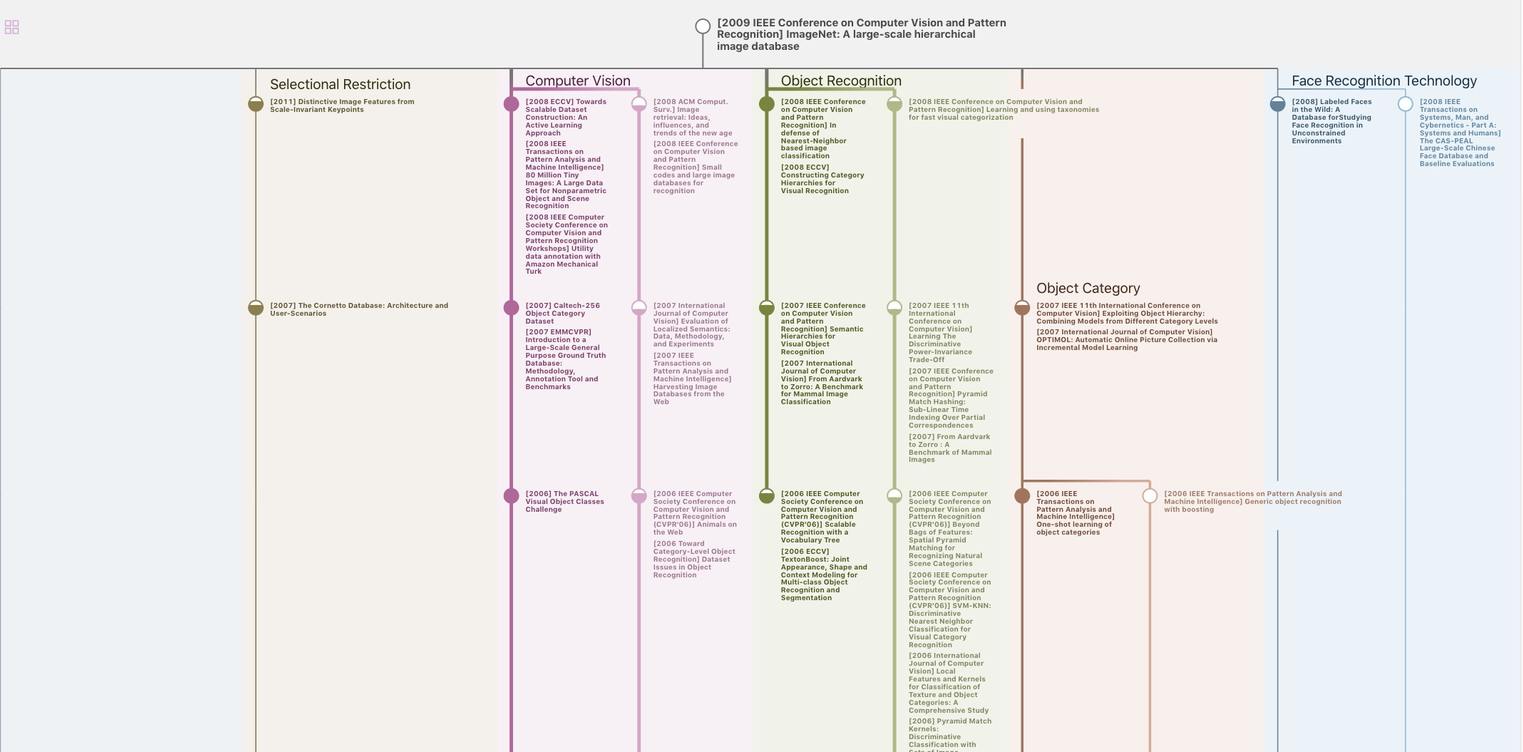
生成溯源树,研究论文发展脉络
Chat Paper
正在生成论文摘要