Deep Learning Based Localization Detection Technique for False Data Injection Attacks
2024 IEEE 4th International Conference on Power, Electronics and Computer Applications (ICPECA)(2024)
摘要
False Data Injection Attacks (FDIA) significantly threaten the state estimation of cyber-physical systems (CPS) in the power grid, jeopardizing the secure operation of the electricity system. Currently, most FDIA detection methods are designed for direct current systems, and traditional DC FDIA detection methods cannot effectively detect alternating current FDIA with satisfactory performance. The existing AC FDIA detection methods typically employ time-series measurement data for direct detection, leading to suboptimal detection accuracy. Moreover, these methods focus on detecting the presence of attacks, failing to locate the positions affected by the attacks accurately. To address these issues, this study introduces a deep learning detection model based on Short-Time Fourier Transform (STFT) for the localization of stealthy FDIA in AC systems. Initially, low-quality data affected by FDIA is removed using a BDD detector. Then, the filtered measurement data is transformed from the time domain to the time-frequency domain using STFT. Finally, the results of the STFT are input into a dual-channel convolutional neural network (DCNN) to extract time-frequency domain features for training and learning, aiming to locate the position of the FDIA attack. Experiments conducted in the IEEE 14 and IEEE 118 bus systems demonstrate that the detection method proposed in this article achieves an average AUC value that is respectively 0.0465, 0.0812 and 0.0255 higher than those of the STFT+BPNN, STFT+LSTM, and STFT+Bi-LSTM algorithms. Furthermore, under various noise intensities and attack strengths, it maintains accuracy, recall, precision, F1 score, and RACC at levels above 0.9036, 0.9425, 0.9588, 0.9506, and 0.899, respectively.
更多查看译文
关键词
AC systems,FDIA,STFT,DCNN,Locate
AI 理解论文
溯源树
样例
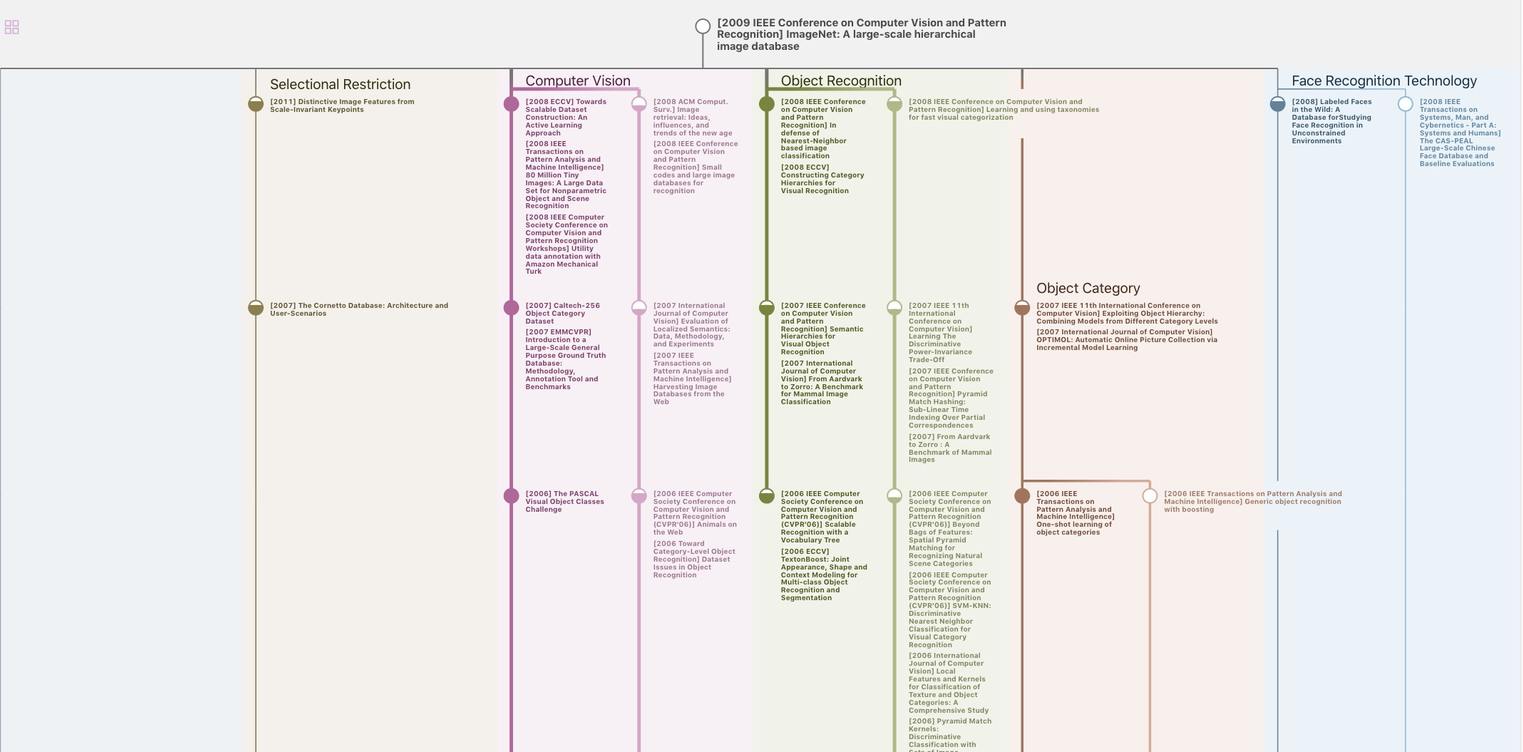
生成溯源树,研究论文发展脉络
Chat Paper
正在生成论文摘要