NTDformer: A Multi-Scale Forecasting Model for Non-Stationary Multilevel Time Series
2023 5th International Conference on Smart Power & Internet Energy Systems (SPIES)(2023)
摘要
The gradual achievement of the dual carbon goal has led to an increasing focus on precise carbon management as a significant area of concern. The dynamic electricity carbon factor plays a crucial role in establishing the link between electricity generation and carbon emissions. However, the inherent unpredictability associated with renewable energy sources poses a challenge to accurately forecasting the future carbon factor based solely on time series data analysis. This paper introduces NTDformer, a model that utilizes the transformer architecture to incorporate temporal patterns and highlight the relationships among multiple variables. In the NTDformer framework, the multivariate time series (MTS) input undergoes an embedding process, resulting in a two-dimensional vector. Subsequently, a Temporal and Dimension Dependence Block (TDBlock) is introduced to effectively capture both temporal and dimensional information. The Hierarchical Encoder-Decoder architecture is adopted to acquire different levels of information, leading to enhanced prediction capabilities. Experimental results from three real datasets show that NTDformer demonstrates superior effectiveness in predicting electricity carbon factors compared to other methods.
更多查看译文
关键词
electricity carbon factor,time series
AI 理解论文
溯源树
样例
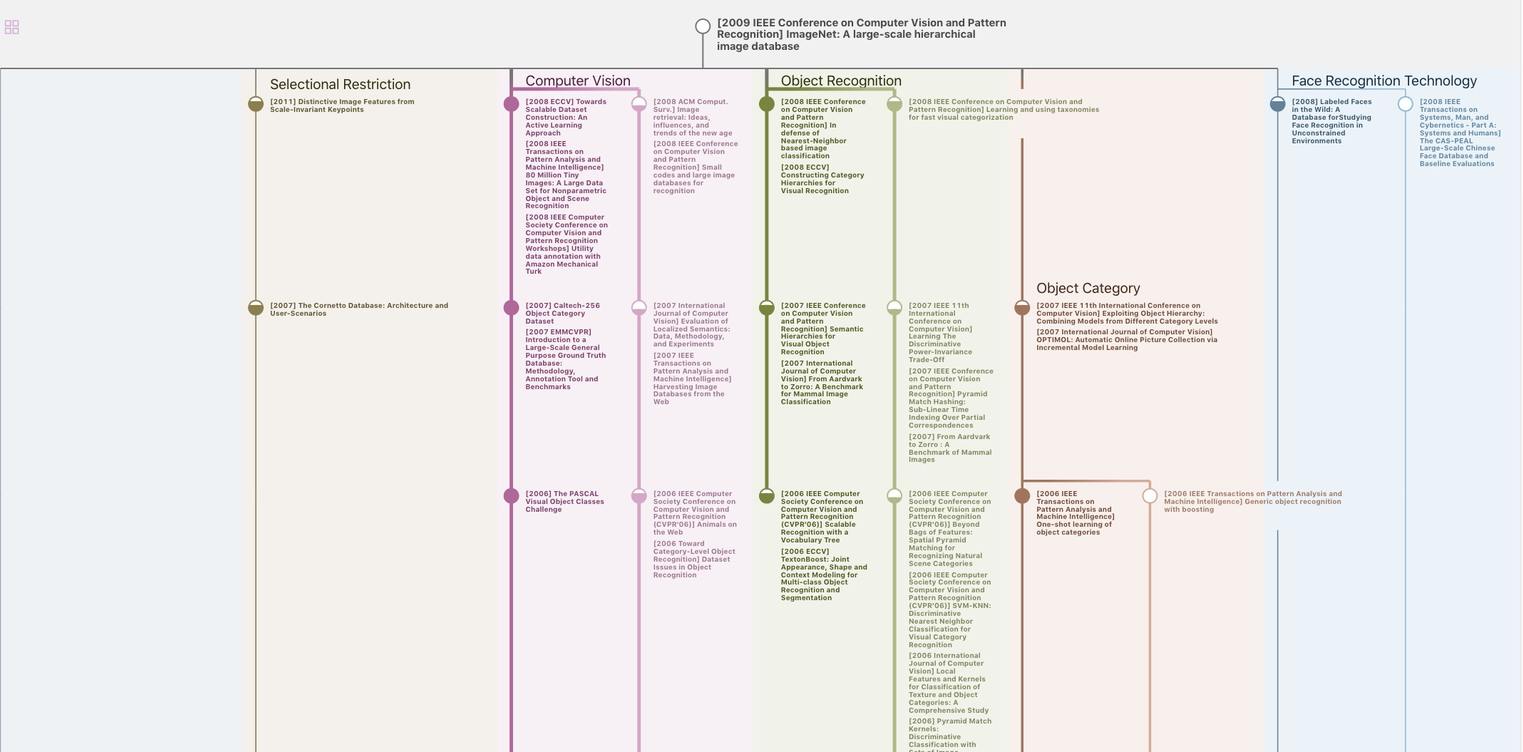
生成溯源树,研究论文发展脉络
Chat Paper
正在生成论文摘要