End-to-End RGB-D Image Compression via Exploiting Channel-Modality Redundancy
AAAI 2024(2024)
摘要
As a kind of 3D data, RGB-D images have been extensively used in object tracking, 3D reconstruction, remote sensing mapping, and other tasks. In the realm of computer vision, the significance of RGB-D images is progressively growing. However, the existing learning-based image compression methods usually process RGB images and depth images separately, which cannot entirely exploit the redundant information between the modalities, limiting the further improvement of the Rate-Distortion performance. With the goal of overcoming the defect, in this paper, we propose a learning-based dual-branch RGB-D image compression framework. Compared with traditional RGB domain compression scheme, a YUV domain compression scheme is presented for spatial redundancy removal. In addition, Intra-Modality Attention (IMA) and Cross-Modality Attention (CMA) are introduced for modal redundancy removal. For the sake of benefiting from cross-modal prior information, Context Prediction Module (CPM) and Context Fusion Module (CFM) are raised in the conditional entropy model which makes the context probability prediction more accurate. The experimental results demonstrate our method outperforms existing image compression methods in two RGB-D image datasets. Compared with BPG, our proposed framework can achieve up to 15% bit rate saving for RGB images.
更多查看译文
关键词
CV: Low Level & Physics-based Vision,CV: Multi-modal Vision
AI 理解论文
溯源树
样例
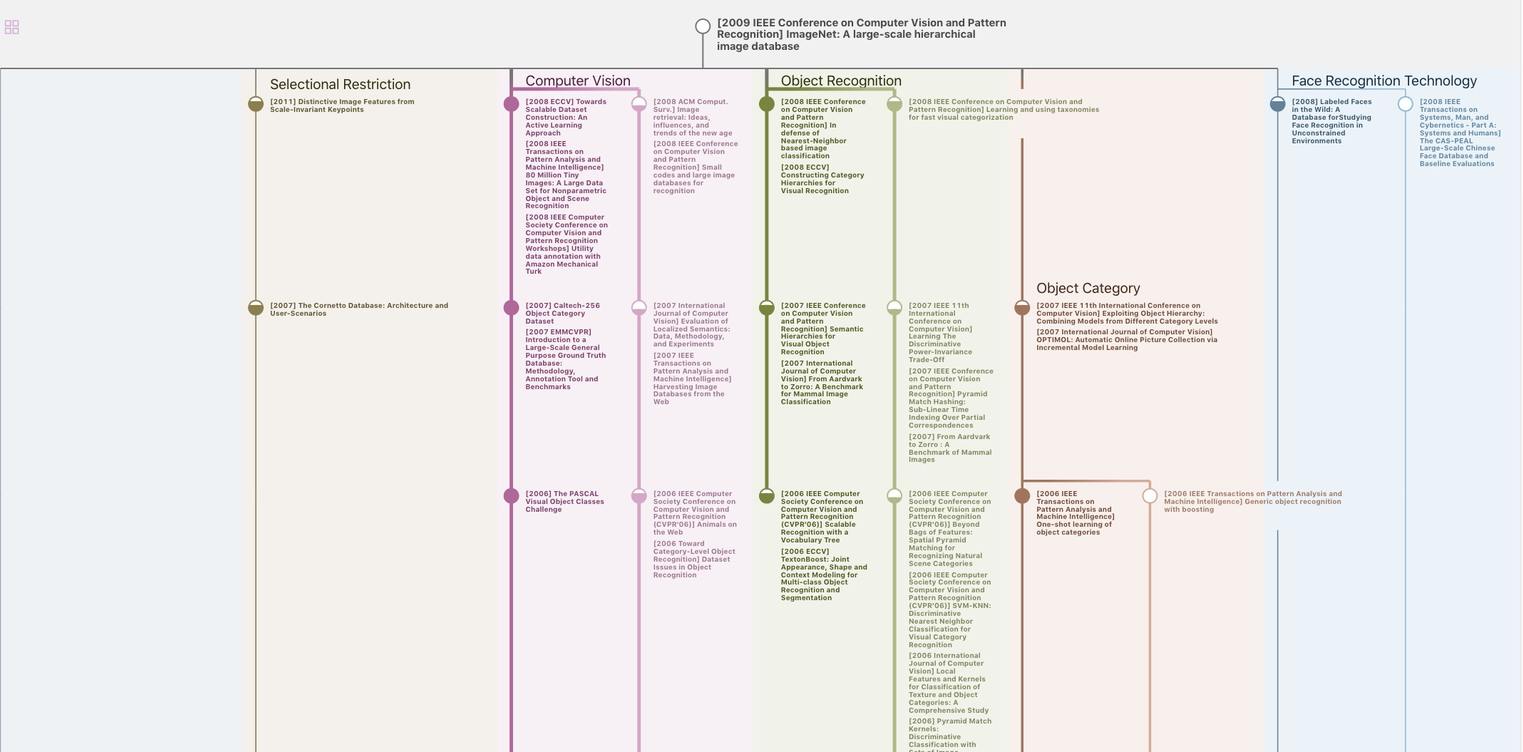
生成溯源树,研究论文发展脉络
Chat Paper
正在生成论文摘要