Towards Dynamic Spatial-Temporal Graph Learning: A Decoupled Perspective
AAAI 2024(2024)
摘要
With the progress of urban transportation systems, a significant amount of high-quality traffic data is continuously collected through streaming manners, which has propelled the prosperity of the field of spatial-temporal graph prediction. In this paper, rather than solely focusing on designing powerful models for static graphs, we shift our focus to spatial-temporal graph prediction in the dynamic scenario, which involves a continuously expanding and evolving underlying graph. To address inherent challenges, a decoupled learning framework (DLF) is proposed in this paper, which consists of a spatial-temporal graph learning network (DSTG) with a specialized decoupling training strategy. Incorporating inductive biases of time-series structures, DSTG can interpret time dependencies into latent trend and seasonal terms. To enable prompt adaptation to the evolving distribution of the dynamic graph, our decoupling training strategy is devised to iteratively update these two types of patterns. Specifically, for learning seasonal patterns, we conduct thorough training for the model using a long time series (e.g., three months of data). To enhance the learning ability of the model, we also introduce the masked auto-encoding mechanism. During this period, we frequently update trend patterns to expand new information from dynamic graphs. Considering both effectiveness and efficiency, we develop a subnet sampling strategy to select a few representative nodes for fine-tuning the weights of the model. These sampled nodes cover unseen patterns and previously learned patterns. Experiments on dynamic spatial-temporal graph datasets further demonstrate the competitive performance, superior efficiency, and strong scalability of the proposed framework.
更多查看译文
关键词
DMKM: Mining of Spatial, Temporal or Spatio-Temporal Data,DMKM: Data Stream Mining,DMKM: Graph Mining, Social Network Analysis & Community
AI 理解论文
溯源树
样例
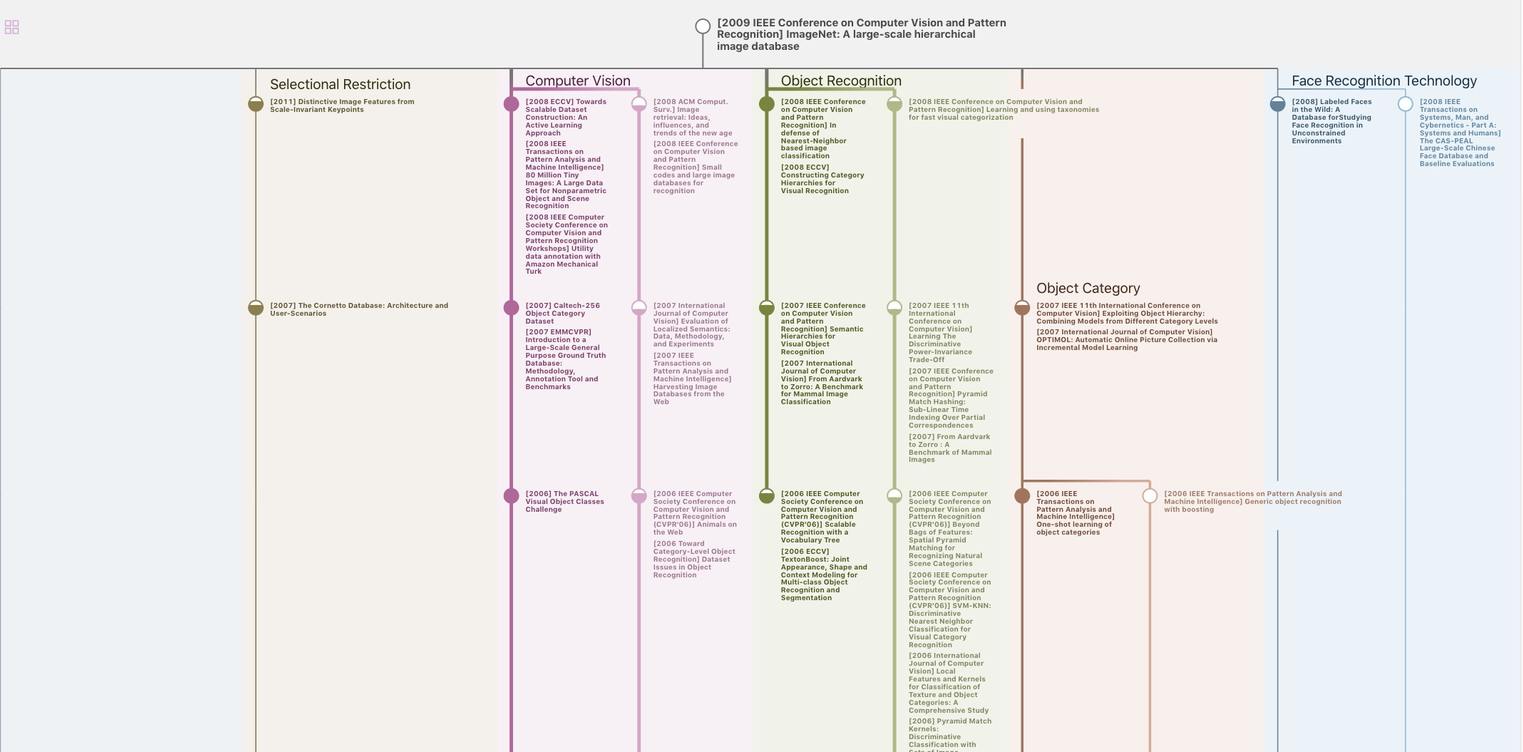
生成溯源树,研究论文发展脉络
Chat Paper
正在生成论文摘要