Generative Calibration of Inaccurate Annotation for Label Distribution Learning
AAAI 2024(2024)
摘要
Label distribution learning (LDL) is an effective learning paradigm for handling label ambiguity. When applying LDL, it typically requires datasets annotated with label distributions. However, obtaining supervised data for LDL is a challenging task. Due to the randomness of label annotation, the annotator can produce inaccurate annotation results for the instance, affecting the accuracy and generalization ability of the LDL model. To address this problem, we propose a generative approach to calibrate the inaccurate annotation for LDL using variational inference techniques. Specifically, we assume that instances with similar features share latent similar label distributions. The feature vectors and label distributions are generated by Gaussian mixture and Dirichlet mixture, respectively. The relationship between them is established through a shared categorical variable, which effectively utilizes the label distribution of instances with similar features, and achieves a more accurate label distribution through the generative approach. Furthermore, we use a confusion matrix to model the factors that contribute to the inaccuracy during the annotation process, which captures the relationship between label distributions and inaccurate label distributions. Finally, the label distribution is used to calibrate the available information in the noisy dataset to obtain the ground-truth label distribution.
更多查看译文
关键词
ML: Multi-class/Multi-label Learning & Extreme Classification
AI 理解论文
溯源树
样例
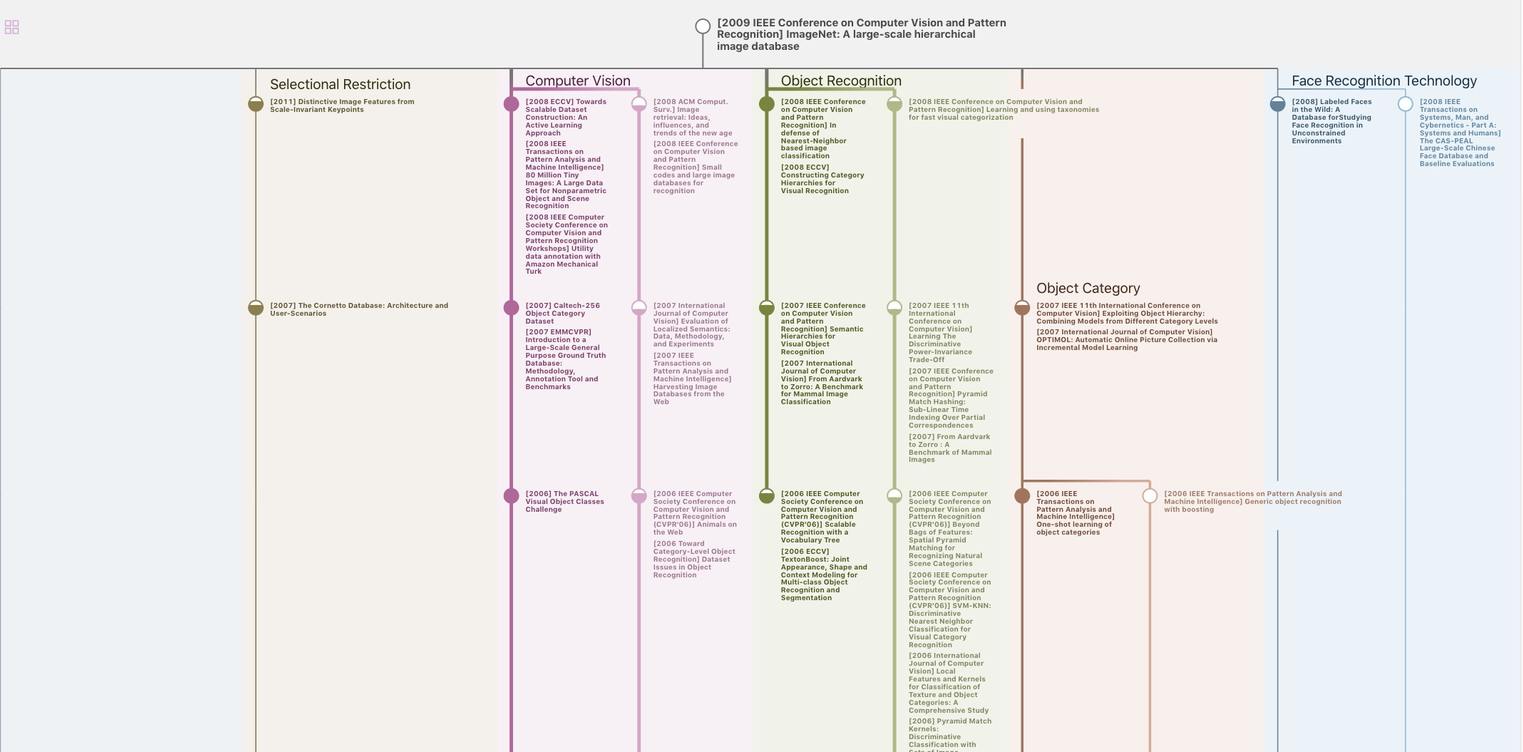
生成溯源树,研究论文发展脉络
Chat Paper
正在生成论文摘要