AGS: Affordable and Generalizable Substitute Training for Transferable Adversarial Attack
AAAI 2024(2024)
摘要
In practical black-box attack scenarios, most of the existing transfer-based attacks employ pretrained models (e.g. ResNet50) as the substitute models. Unfortunately, these substitute models are not always appropriate for transfer-based attacks. Firstly, these models are usually trained on a largescale annotated dataset, which is extremely expensive and time-consuming to construct. Secondly, the primary goal of these models is to perform a specific task, such as image classification, which is not developed for adversarial attacks. To tackle the above issues, i.e., high cost and over-fitting on taskspecific models, we propose an Affordable and Generalizable Substitute (AGS) training framework tailored for transferbased adversarial attack. Specifically, we train the substitute model from scratch by our proposed adversary-centric constrastive learning. This proposed learning mechanism introduces another sample with slight adversarial perturbations as an additional positive view of the input image, and then encourages the adversarial view and two benign views to interact comprehensively with each other. To further boost the generalizability of the substitute model, we propose adversarial invariant learning to maintain the representations of the adversarial example invariants under augmentations with various strengths. Our AGS model can be trained solely with unlabeled and out-of domain data and avoid overfitting to any task-specific models, because of its inherently self-supervised nature. Extensive experiments demonstrate that our AGS achieves comparable or superior performance compared to substitute models pretrained on the complete ImageNet training set, when executing attacks across a diverse range of target models, including ViTs, robustly trained models, object detection and segmentation models. Our source codes are available at https://github.com/lwmming/AGS.
更多查看译文
关键词
CV: Adversarial Attacks & Robustness,ML: Adversarial Learning & Robustness
AI 理解论文
溯源树
样例
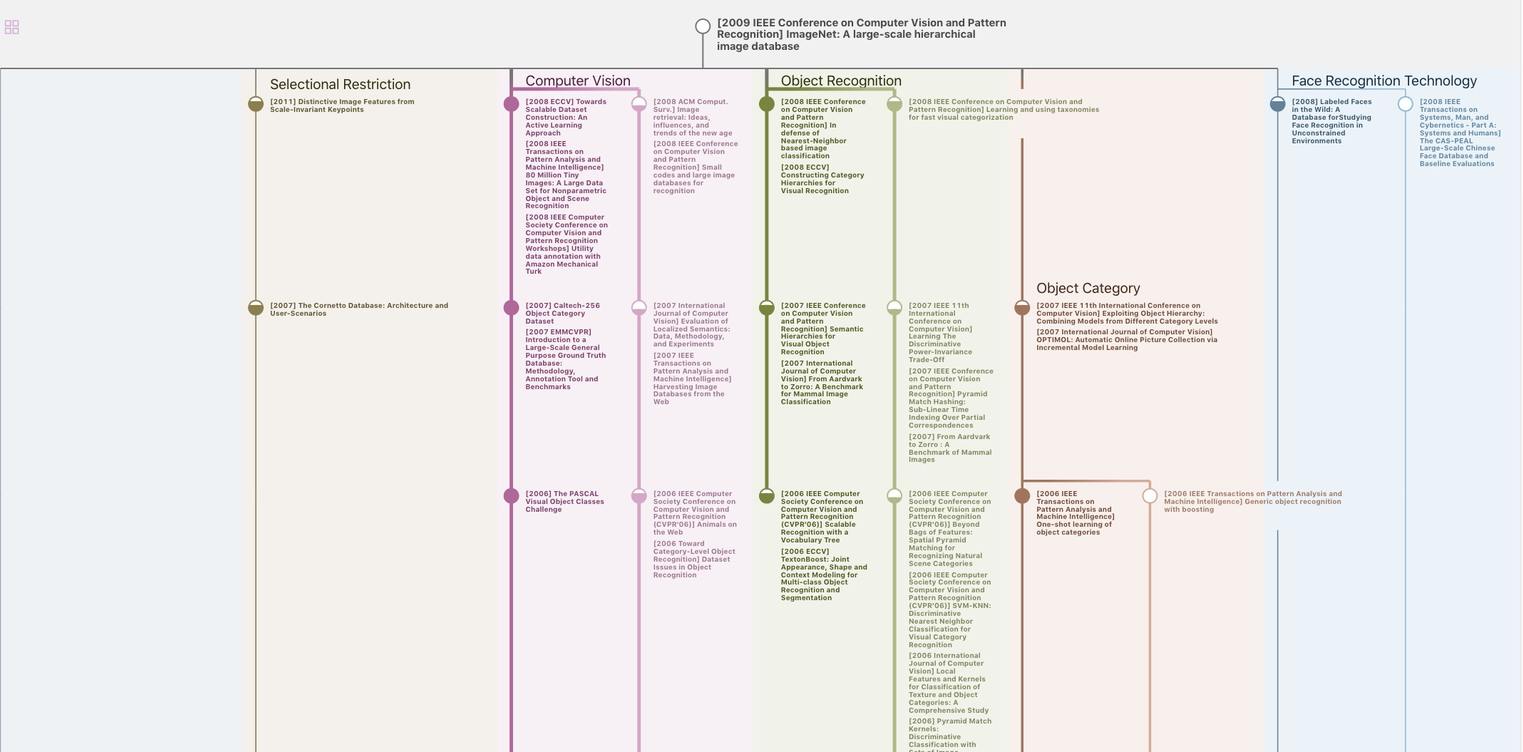
生成溯源树,研究论文发展脉络
Chat Paper
正在生成论文摘要