Sparse Bayesian Deep Learning for Cross Domain Medical Image Reconstruction
AAAI 2024(2024)
摘要
Cross domain medical image reconstruction aims to address the issue that deep learning models trained solely on one source dataset might not generalize effectively to unseen target datasets from different hospitals. Some recent methods achieve satisfactory reconstruction performance, but often at the expense of extensive parameters and time consumption. To strike a balance between cross domain image reconstruction quality and model computational efficiency, we propose a lightweight sparse Bayesian deep learning method. Notably, we apply a fixed-form variational Bayes (FFVB) approach to quantify pixel-wise uncertainty priors derived from degradation distribution of the source domain. Furthermore, by integrating the uncertainty prior into the posterior sampled through stochastic gradient Langevin dynamics (SGLD), we develop a training strategy that dynamically generates and optimizes the prior distribution on the network weights for each unseen domain. This strategy enhances generalizability and ensures robust reconstruction performance. When evaluated on medical image reconstruction tasks, our proposed approach demonstrates impressive performance across various previously unseen domains.
更多查看译文
关键词
CV: Medical and Biological Imaging,ML: Bayesian Learning,ML: Transfer, Domain Adaptation, Multi-Task Learning
AI 理解论文
溯源树
样例
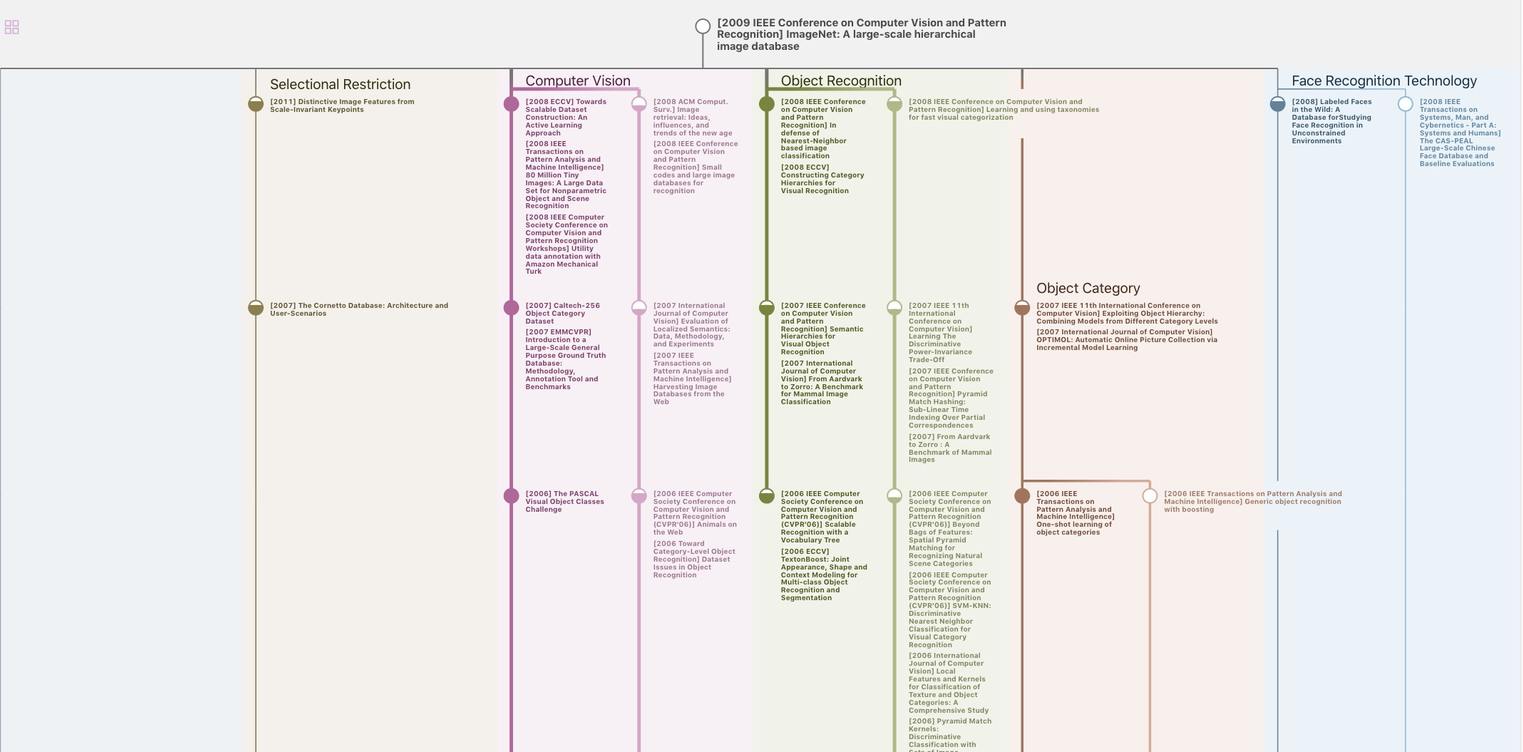
生成溯源树,研究论文发展脉络
Chat Paper
正在生成论文摘要