DVSAI: Diverse View-Shared Anchors Based Incomplete Multi-View Clustering
AAAI 2024(2024)
摘要
In numerous real-world applications, it is quite common that sample information is partially available for some views due to machine breakdown or sensor failure, causing the problem of incomplete multi-view clustering (IMVC). While several IMVC approaches using view-shared anchors have successfully achieved pleasing performance improvement, (1) they generally construct anchors with only one dimension, which could deteriorate the multi-view diversity, bringing about serious information loss; (2) the constructed anchors are typically with a single size, which could not sufficiently characterize the distribution of the whole samples, leading to limited clustering performance. For generating view-shared anchors with multi-dimension and multi-size for IMVC, we design a novel framework called Diverse View-Shared Anchors based Incomplete multi-view clustering (DVSAI). Concretely, we associate each partial view with several potential spaces.
In each space, we enable anchors to communicate among views and generate the view-shared anchors with space-specific dimension and size. Consequently, spaces with various scales make the generated view-shared anchors enjoy diverse dimensions and sizes. Subsequently, we devise an integration scheme with linear computational and memory expenditures to integrate the outputted multi-scale unified anchor graphs such that running spectral algorithm generates the spectral embedding. Afterwards, we theoretically demonstrate that DVSAI owns linear time and space costs, thus well-suited for tackling large-size datasets. Finally, comprehensive experiments confirm the effectiveness and advantages of DVSAI.
更多查看译文
关键词
ML: Clustering,ML: Multi-instance/Multi-view Learning
AI 理解论文
溯源树
样例
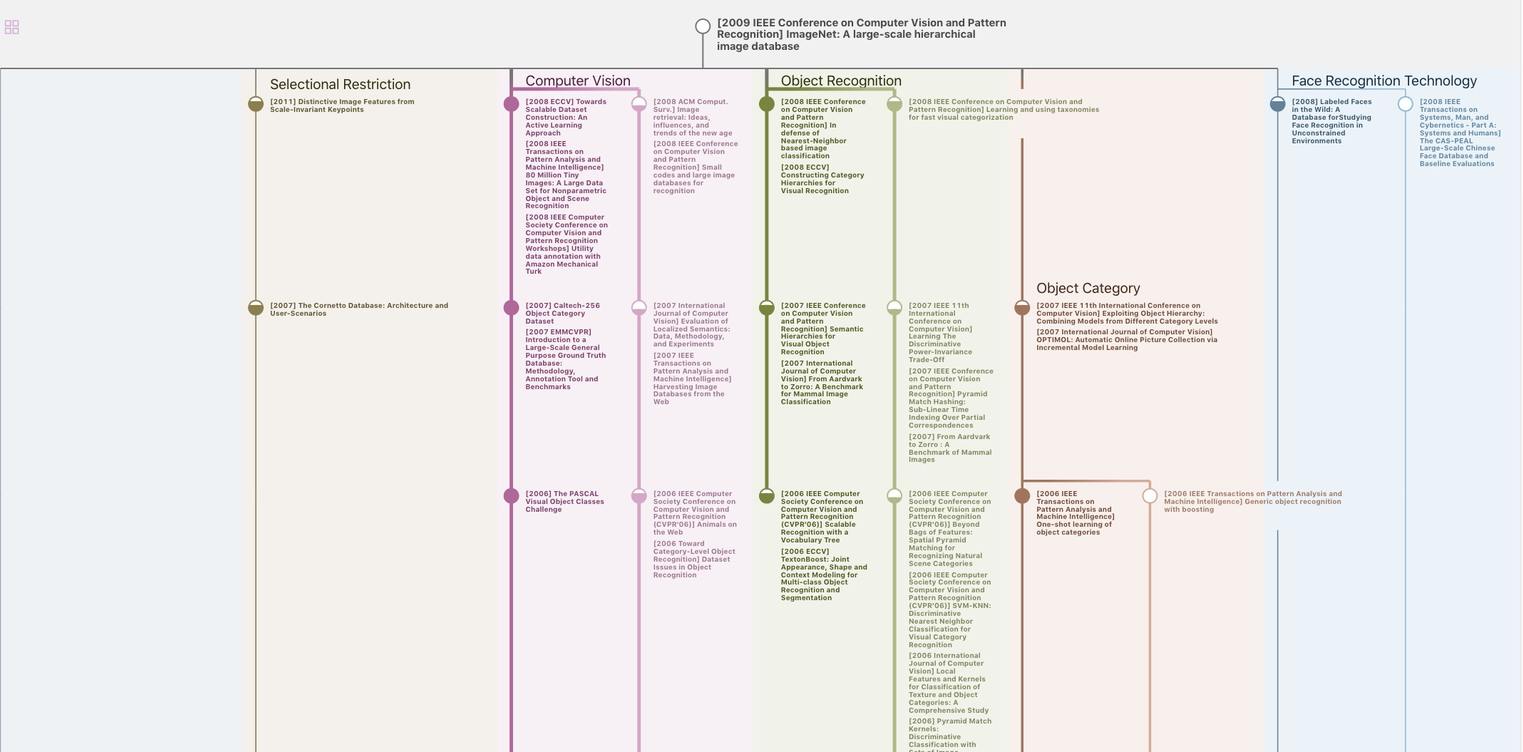
生成溯源树,研究论文发展脉络
Chat Paper
正在生成论文摘要