Cross-modality Feature Fusion for Night Pedestrian Detection
Frontiers in physics(2024)
摘要
Night pedestrian detection with visible image only suffers from the dilemma of high miss rate due to poor illumination conditions. Cross-modality fusion can ameliorate this dilemma by providing complementary information to each other through infrared and visible images. In this paper, we propose a cross-modal fusion framework based on YOLOv5, which is aimed at addressing the challenges of night pedestrian detection under low-light conditions. The framework employs a dual-stream architecture that processes visible images and infrared images separately. Through the Cross-Modal Feature Rectification Module (CMFRM), visible and infrared features are finely tuned on a granular level, leveraging their spatial correlations to focus on complementary information and substantially reduce uncertainty and noise from different modalities. Additionally, we have introduced a two-stage Feature Fusion Module (FFM), with the first stage introducing a cross-attention mechanism for cross-modal global reasoning, and the second stage using a mixed channel embedding to produce enhanced feature outputs. Moreover, our method involves multi-dimensional interaction, not only correcting feature maps in terms of channel and spatial dimensions but also applying cross-attention at the sequence processing level, which is critical for the effective generalization of cross-modal feature combinations. In summary, our research significantly enhances the accuracy and robustness of nighttime pedestrian detection, offering new perspectives and technical pathways for visual information processing in low-light environments.
更多查看译文
关键词
pedestrian detection,YOLOv5,vision transformer,CNNs,feature fusion
AI 理解论文
溯源树
样例
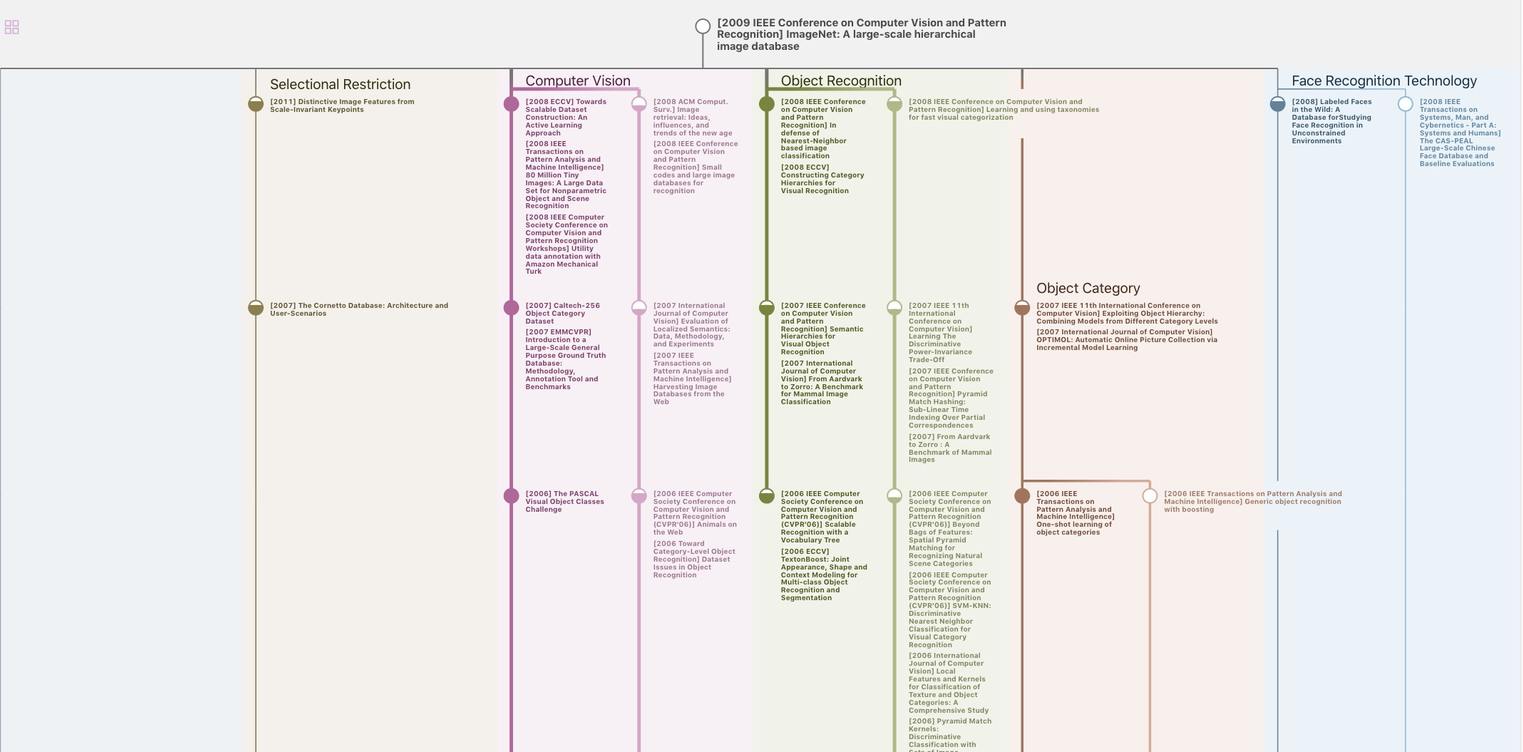
生成溯源树,研究论文发展脉络
Chat Paper
正在生成论文摘要