AI/ML Model Training in O-RAN: Assessing Datasets for Hoarding and Choosing Strategies
2023 IEEE International Conference on Advanced Networks and Telecommunications Systems (ANTS)(2023)
摘要
The advent of Open Radio Access Network (O-RAN) is transforming the traditional cellular networks into flexible, interoperable, and innovative through open standard interfaces (i.e., O1, A1, E2) and RAN Intelligent Controllers (RICs) — Near-real time and Non-real time (Near- and Non-RT RIC). These RICs leverage AI/ML models for intelligent decisions, interacting with RAN components — centralized units (CUs), distributed units (DUs), and radio units (RUs). Choosing the appropriate data for AI/ML model training in O-RAN is critical, as training based on the nature of the data, whether homogeneous or heterogeneous, can significantly improve model accuracy and efficient resource utilization. This paper introduces an approach that determines the dataset homogeneity by employing the Kolmogorov-Smirnov test (KS Test) and also considers evaluating both real-time and synthetic datasets.
更多查看译文
关键词
Open RAN,AI/ML Model,Beyond fifth-generation (B5G) Networks,Choosing,Hoarding
AI 理解论文
溯源树
样例
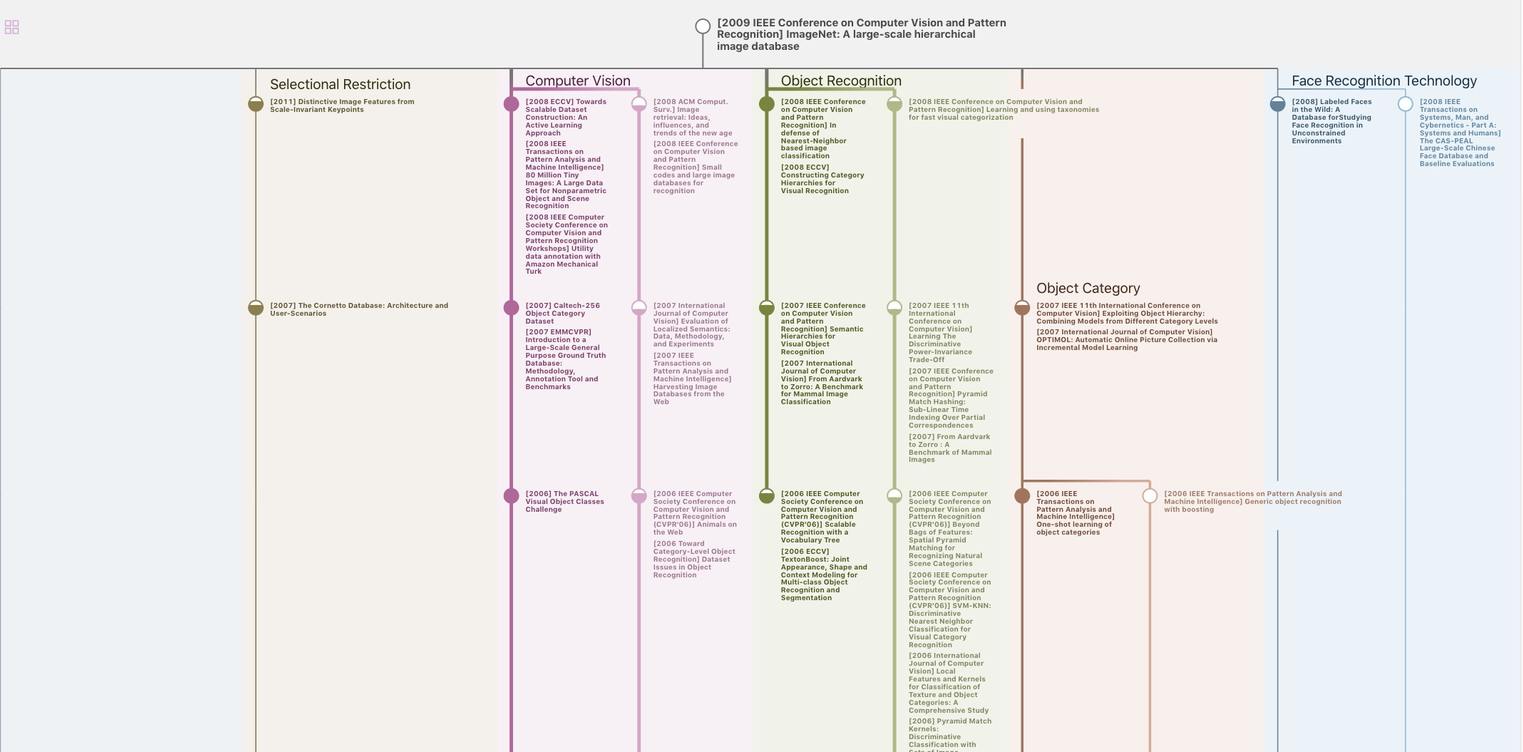
生成溯源树,研究论文发展脉络
Chat Paper
正在生成论文摘要