ECHO-GL: Earnings Calls-Driven Heterogeneous Graph Learning for Stock Movement Prediction
AAAI 2024(2024)
摘要
Stock movement prediction serves an important role in quantitative trading. Despite advances in existing models that enhance stock movement prediction by incorporating stock relations, these prediction models face two limitations, i.e., constructing either insufficient or static stock relations, which fail to effectively capture the complex dynamic stock relations because such complex dynamic stock relations are influenced by various factors in the ever-changing financial market. To tackle the above limitations, we propose a novel stock movement prediction model ECHO-GL based on stock relations derived from earnings calls. ECHO-GL not only constructs comprehensive stock relations by exploiting the rich semantic information in the earnings calls but also captures the movement signals between related stocks based on multimodal and heterogeneous graph learning. Moreover, ECHO-GL customizes learnable stock stochastic processes based on the post earnings announcement drift (PEAD) phenomenon to generate the temporal stock price trajectory, which can be easily plugged into any investment strategy with different time horizons to meet investment demands. Extensive experiments on two financial datasets demonstrate the effectiveness of ECHO-GL on stock price movement prediction tasks together with high prediction accuracy and trading profitability.
更多查看译文
关键词
ML: Applications,ML: Graph-based Machine Learning
AI 理解论文
溯源树
样例
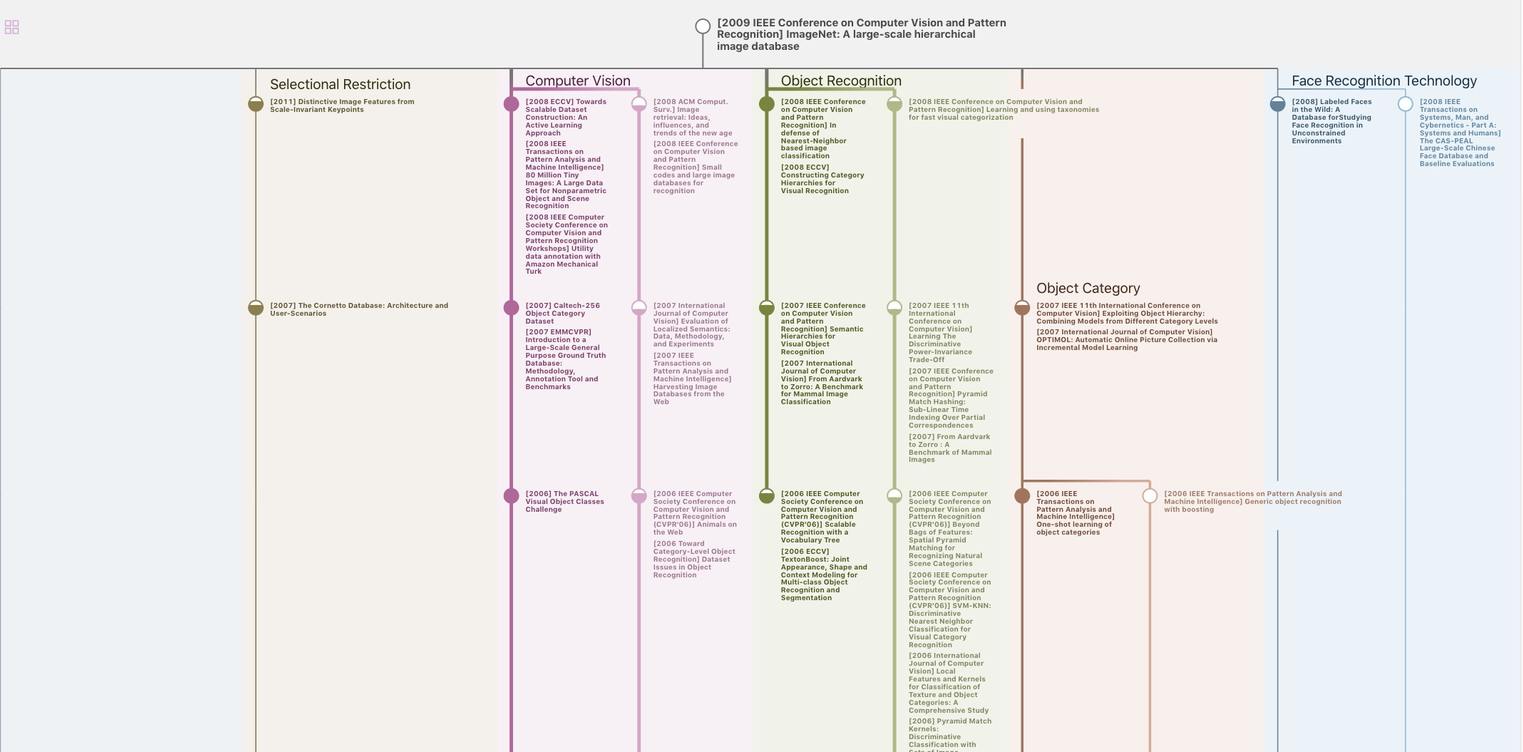
生成溯源树,研究论文发展脉络
Chat Paper
正在生成论文摘要