Complex Crack Segmentation and Quantitative Evaluation of Engineering Materials Based on Deep Learning Methods
IEEE ACCESS(2024)
摘要
Recognizing and quantifying microcracks on surfaces are crucial for early detection of structural damage, as they can lead to more complex issues in engineering structures. In this study, a dataset reflecting varying surface cracks in various engineering materials from the 2018 Ecuador earthquake was constructed. Furthermore, we proposed deep learning-based methods for recognizing and quantifying complex surface cracks. The methods utilized an enhanced U-Net semantic segmentation model, along with noise reduction and topological parameter extraction post-processing algorithms. The intersection-over-union recognition capacity for simple extended, complex crisscrossed, and microcracks was significantly improved, reaching as high as 91.71%, 89.56%, and 100% compared to the original U-Net. The results showed that the enhanced U-Net architecture had a steady training process and high accuracy in segmenting various types of cracks, especially microcracks, which were difficult to detect through manual observation or conventional imaging techniques. Therefore, the proposed model is suitable for pre-alarming monitoring of multiscale damage. A noise reduction post-processing algorithm was introduced to enhance the segmenting capability by extracting detailed characteristics of prediction samples. Statistical analysis of false-positive samples showed that 90% of misrecognitions were eliminated, compared to the original situations. The study also developed an approach for determining the topological parameters of complicated patterns on hierarchical cracks in the refined masks at the pixel level. The average relative errors of area, perimeter, length, compactness, average width, and centroid coordinate for crack samples in ground-truth annotations and refined masks are below 10.75%, 1.89%, 1.51%, 6.20%, 9.75%, and 0.72%, respectively. Finally, a graphical user interface integrating all algorithms was explored and applied for damage assessment of structural components under various environmental disturbances.
更多查看译文
关键词
Complex surface cracks,enhanced U-Net,geometrical quantification,noise reduction,semantic segmentation,structural damage recognition
AI 理解论文
溯源树
样例
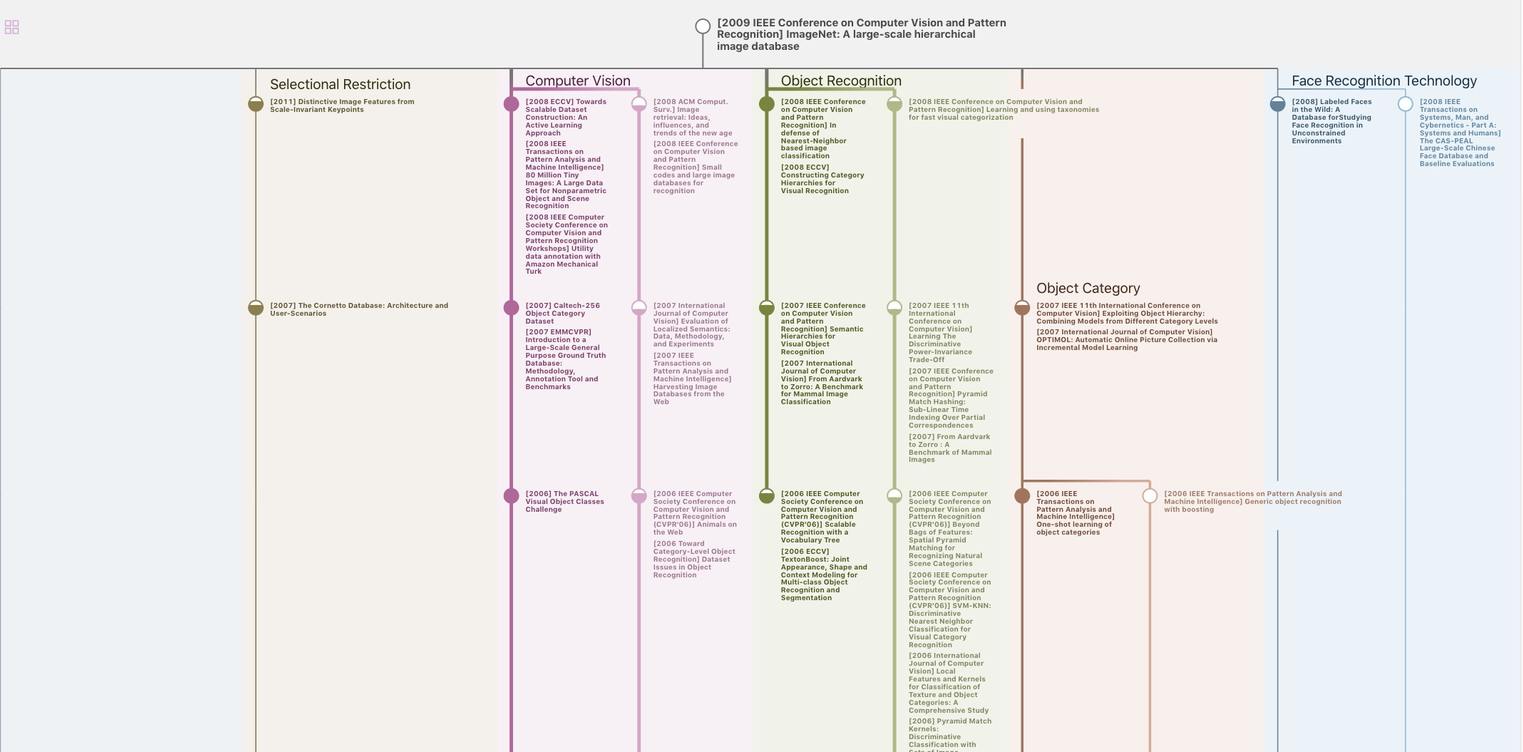
生成溯源树,研究论文发展脉络
Chat Paper
正在生成论文摘要