Adaptive magnification network for precise tumor analysis in histopathological images
Computers in Human Behavior(2024)
摘要
The variable magnification levels in histopathology images make it difficult to accurately categorize tumor regions in breast cancer histology. In this study, a novel architecture for accurate image interpretation MagNet is presented. With specific modules like Separable Dilation Convolution (SDC), Separable Dilation Skip Block (SDSB), and Point-wise Reformation Block (PRB), MagNet uses a Parallel U-Net (PU-Net) infrastructure. SDC in the PU-Net encoder ensures downsampled generalized feature representations by capturing characteristic attributes at varying magnifications. Using feature upsampling, attribute mapping merging, and PRB for precise feature capture, the decoder improves reconstruction. While PRB combines data from several decoder levels, SDSB creates vital links between the encoder and decoder layers. MagNet requires less processing of histopathology images and improves multi-magnification feature maps. MagNet performs exceptionally well, constantly outperforming rivals in terms of accuracy (0.98), F1 score (0.97), sensitivity (0.96), and specificity (0.97). The effectiveness of MagNet and its revolutionary potential in cancer diagnostics are shown by these quantitative data.
更多查看译文
关键词
Breast cancer histopathology,Magnification invariance,Stain normalization,Multi-level features,Cancer diagnostics,Histopathological images
AI 理解论文
溯源树
样例
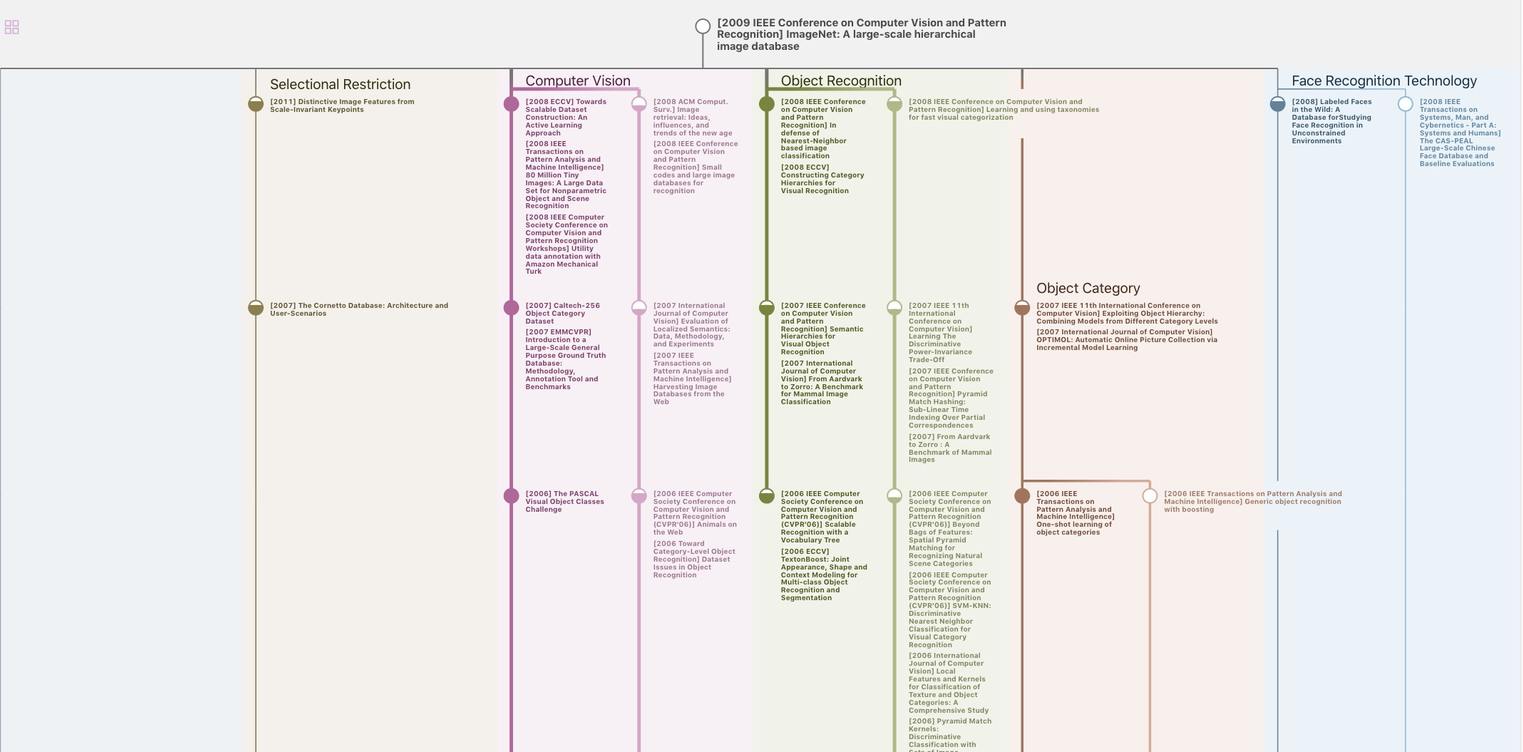
生成溯源树,研究论文发展脉络
Chat Paper
正在生成论文摘要