Graph Representation Learning in the ITS: Car-Following Informed Spatiotemporal Network for Vehicle Trajectory Predictions
IEEE Transactions on Intelligent Vehicles(2024)
摘要
Multimodal synchronization has become the research highlight of the ITS, where complex driving scenarios, various types of vehicles and diverse data sources are crucial constituents. As real-time microscopic traffic characteristics can be vividly represented by graph data, we strive to achieve accurate trajectory predictions via graph-structured series for the stability and the efficiency of transportation. Although the existing data-driven algorithms have achieved fabulous accuracy in various simulation tasks, there are limitations in the distribution and the number of vehicles under investigation. With car-following patterns applied as physical information, we derive the adjacency matrix and design graph filters to explore the spatial dependence between vehicles via the graph-represented multi-lane traffic. The multi-head attention layer is attached to the spatiotemporal convolutional network as an extension. The rationality and the superiority of our model are validated on two calibrated datasets. Through error comparisons, we discuss the role of changeable hyper-parameters to deduce the optimal model for one-step and multi-step predictions. Novel ideas are shared in this paper to simplify the complexity of trajectory prediction in the synchronized transportation system.
更多查看译文
关键词
Trajectory prediction,Graph representation,Spatiotemporal convolutional network,Multi-head attention
AI 理解论文
溯源树
样例
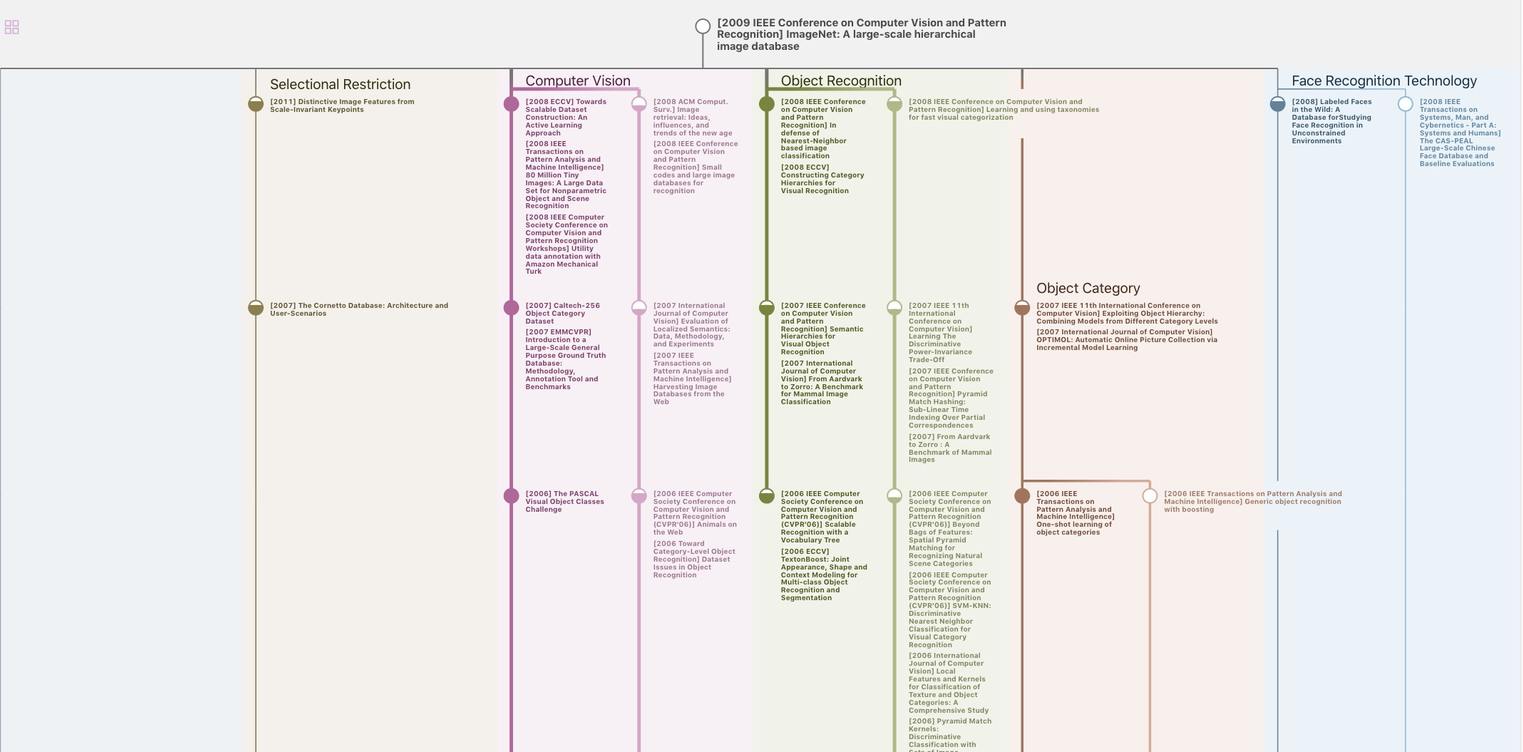
生成溯源树,研究论文发展脉络
Chat Paper
正在生成论文摘要