Enhancing Cybersecurity in Industrial Control System with Autonomous Defense Using Normalized Proximal Policy Optimization Model.
International Conference on Parallel and Distributed Systems(2023)
摘要
Industrial control networks are frequent targets of cyber attacks, calling for autonomous defense strategies to combat threats effectively and promptly. We propose to leverage Reinforcement Learning (RL) to train defenders how to select the most appropriate actions in response to attackers’ behavior. We model the defender and attacker as agents in RL environments and define action space and reward function. We focus on evaluating value-based and policy gradient-based RL algorithms and propose enhancements to Proximal Policy Optimization (PPO) method through normalization. We then leverage CybORG, a simulation tool to study how the RL algorithms perform to achieve autonomous defense. Our extensive experiments reveal that the proposed normalized PPO outperforms other models in terms of stability, robustness, and average rewards. The results also demonstrate the effectiveness of the defender in protecting the operational server upon changing the attacker’s position. Additionally, increasing the number of attackers had a significant impact on policy-based algorithms, particularly PPO, with decreased reward values highlighting the increased vulnerability of the network.
更多查看译文
关键词
Cybersecurity,Reinforcement Learning,Autonomous Defence
AI 理解论文
溯源树
样例
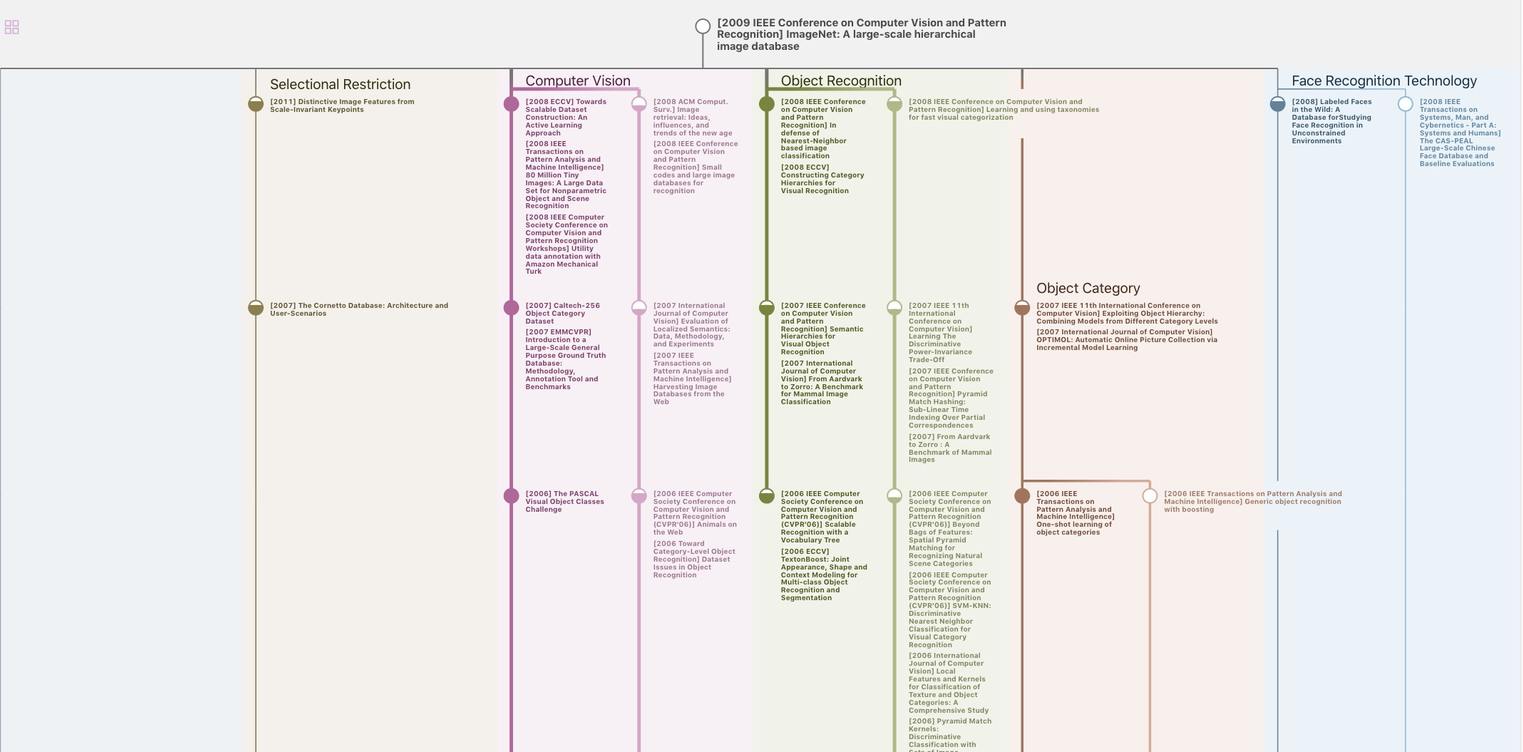
生成溯源树,研究论文发展脉络
Chat Paper
正在生成论文摘要