When Lyapunov Drift Based Queue Scheduling Meets Adversarial Bandit Learning
IEEE-ACM TRANSACTIONS ON NETWORKING(2024)
摘要
In this paper, we study scheduling of a queueing system with zero knowledge of instantaneous network conditions. We consider a one-hop single-server queueing system consisting of $K$ queues, each with time-varying and non-stationary arrival and service rates. Our scheduling approach builds on an innovative combination of adversarial bandit learning and Lyapunov drift minimization, without knowledge of the instantaneous network state (the arrival and service rates) of each queue. We then present two novel algorithms SoftMW (SoftMaxWeight) and SSMW (Sliding-window SoftMaxWeight), both capable of stabilizing systems that can be stabilized by some (possibly unknown) sequence of randomized policies whose time-variation satisfies a mild condition. We further generalize our results to the setting where arrivals and departures only have bounded moments instead of being deterministically bounded and propose SoftMW+ and SSMW+ that are capable of stabilizing the system. As a building block of our new algorithms, we also extend the classical EXP3.S algorithm for multi-armed bandits to handle unboundedly large feedback signals, which can be of independent interest.
更多查看译文
关键词
Queueing analysis,Dynamic scheduling,Time-varying systems,Knowledge engineering,Stability analysis,Scheduling algorithms,Vectors,Scheduling,queueing,bandit learning,Lyapunov analysis
AI 理解论文
溯源树
样例
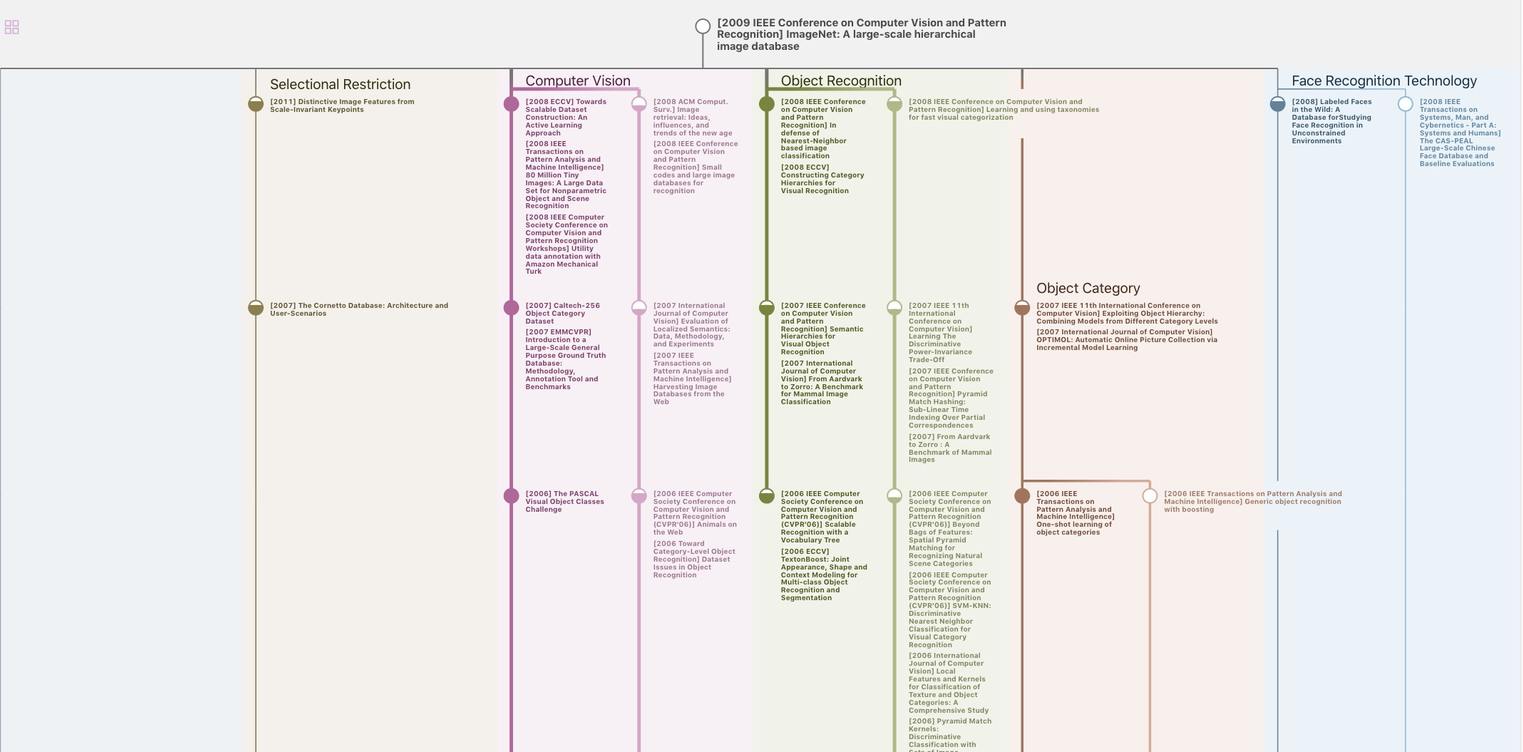
生成溯源树,研究论文发展脉络
Chat Paper
正在生成论文摘要