PD-CR: Patch-Based Diffusion Using Constrained Refinement for Image Restoration
IEEE SIGNAL PROCESSING LETTERS(2024)
摘要
Diffusion models, which are state-of-the-art generative models, have been widely applied to image restoration tasks. However, most image restoration methods based on diffusion models require a large amount of computational memory, making it difficult to use them with high-resolution images. Although patch-based diffusion models have emerged to address this problem, these models are limited in effectively mitigating boundary artifacts and producing results close to the ground truth. In this letter, we propose Patch-based Diffusion using Constrained Refinement (PD-CR) that refines the noise estimated by patch-based diffusion models to produce a restored image while keeping the luminance of the input degraded image. Leveraging patch-based diffusion models, the proposed method can handle a high-resolution image as input with minimal memory requirements. Our experiments on various image restoration tasks, such as image denoising and raindrop removal, demonstrate that the proposed method is better than or on par with the state-of-the-art methods.
更多查看译文
关键词
Diffusion models,image denoising,image restoration,raindrop removal
AI 理解论文
溯源树
样例
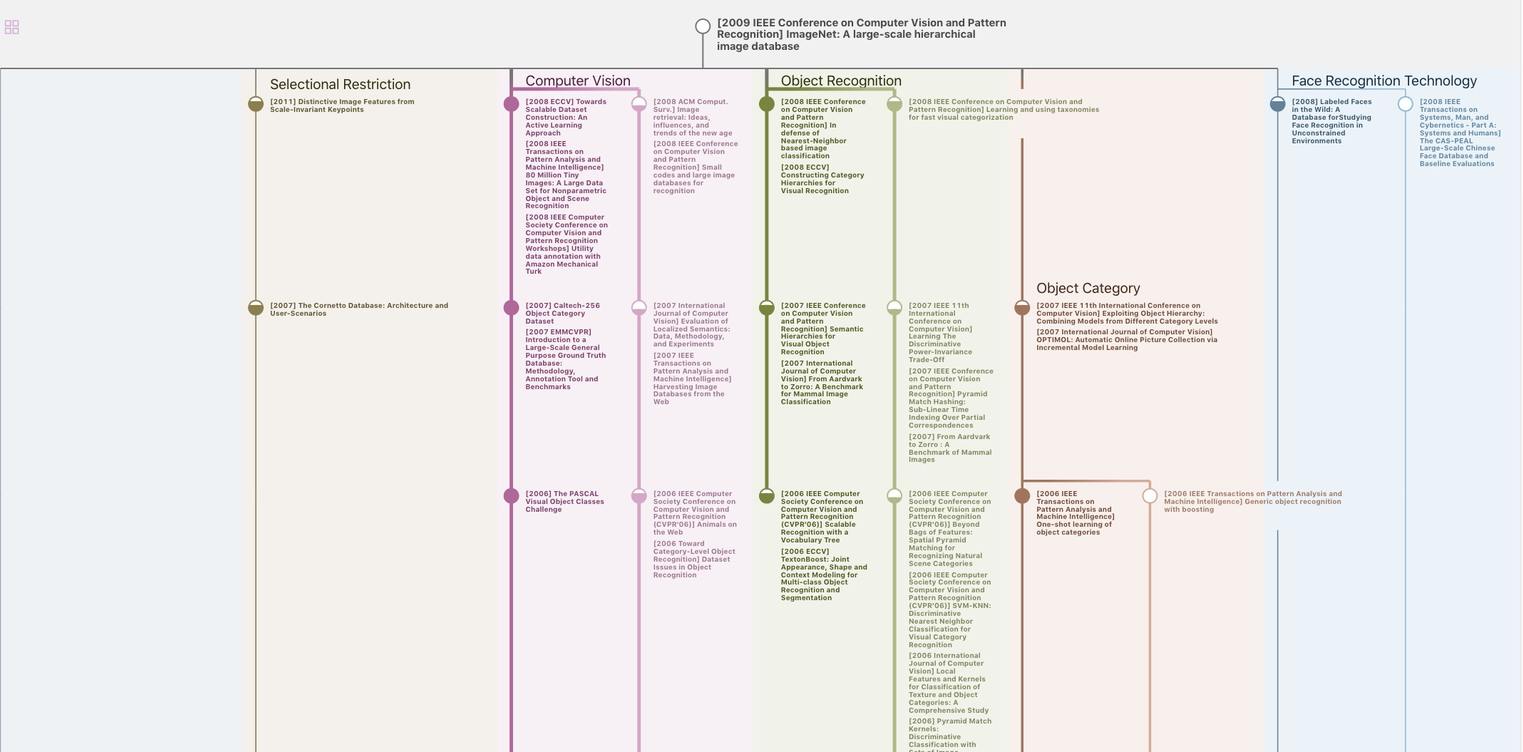
生成溯源树,研究论文发展脉络
Chat Paper
正在生成论文摘要