Super-Tsetlin: Superconducting Tsetlin Machines
IEEE TRANSACTIONS ON APPLIED SUPERCONDUCTIVITY(2024)
摘要
The recently proposed Tsetlin machine (TM) is a low-complexity and versatile machine learning architecture that learns a collection of propositional clauses to describe or classify data. Each clause is constructed from a set of Tsetlin Automata (TAs), which are used to update the model during learning. TMs have been widely used, including image analysis, dimension reduction and intrusion detection, and recommendation systems. TMs provide interpretable results outperforming state-of-the-art machine learning approaches on various tasks. Existing hardware implementations of TMs are mainly based on Field Programmable Gate Arrays (FPGAs) and CMOS accelerator integrated circuit (IC) modules. These hardware solutions show high power efficiencies and pattern recognition accuracies compared to traditional machine learning algorithms. In this work, we explore the use of superconducting rapid single-flux quantum (RSFQ) technology to implement TMs, which would benefit from the ultra-low power consumption and high processing speed of superconducting technology. We designed circuits for TAs, propositional clauses, and the learning algorithm based on RSFQ circuits. To demonstrate the hardware's functionality in simulations, we train the system to learn the noisy xor problem. We have also modeled larger TMs with more complex applications such as image analysis. We estimate that the dynamic power dissipation is less than 0.5 mW for a TM with eight clauses and four TAs per clause, and processing speeds up to 10 GHz using MIT-LL SFQ5ee process with a critical current density of 100 mu A/ mu m( 2) . These results show RSFQ as a potential candidate for implementing Tsetlin machine based massively parallel architectures.
更多查看译文
关键词
Machine learning,Training data,rapid single-flux quantum (RSFQ),Tsetlin machines (TMs)
AI 理解论文
溯源树
样例
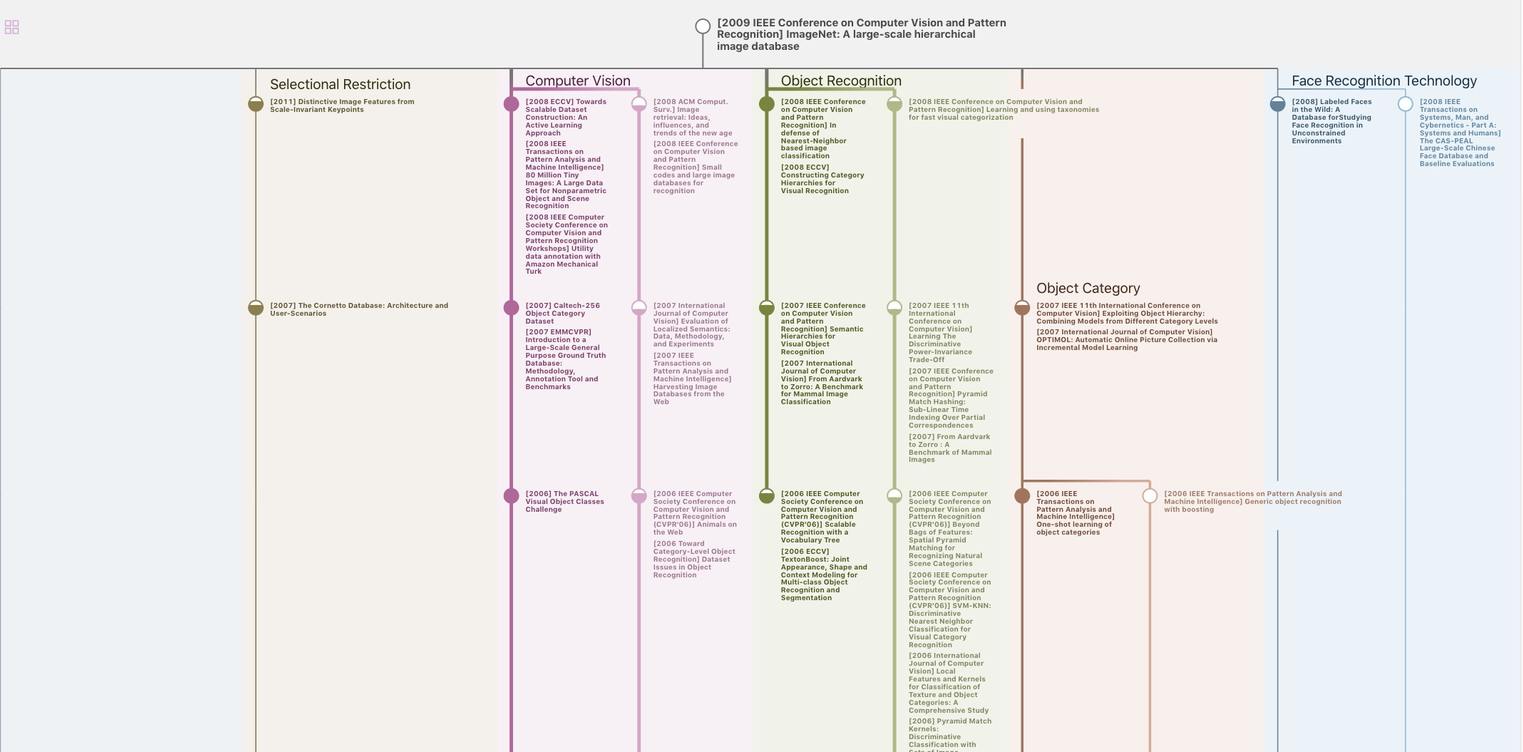
生成溯源树,研究论文发展脉络
Chat Paper
正在生成论文摘要