Polyp Generalization via Diversifying Style at Feature-Level Space
APPLIED SCIENCES-BASEL(2024)
摘要
In polyp segmentation, the latest notable topic revolves around polyp generalization, which aims to develop deep learning-based models capable of learning from single or multiple source domains and applying this knowledge to unseen datasets. A significant challenge in real-world clinical settings is the suboptimal performance of generalized models due to domain shift. Convolutional neural networks (CNNs) are often biased towards low-level features, such as style features, impacting generalization. Despite attempts to mitigate this bias using data augmentation techniques, learning model-agnostic and class-specific feature representations remains complex. Previous methods have employed image-level transformations with styles to supplement training data diversity. However, these approaches face limitations in ensuring style diversity due to restricted style sources, limiting the utilization of the potential style space. To address this, we propose a straightforward yet effective style conversion and generation module integrated into the UNet model. This module transfers diverse yet plausible style features to the original training data at the feature-level space, ensuring that generated styles align closely with the original data. Our method demonstrates superior performance in single-domain generalization tasks across five datasets compared to prior methods.
更多查看译文
关键词
polyp generalization,polyp segmentation,domain generalization,image segmentation
AI 理解论文
溯源树
样例
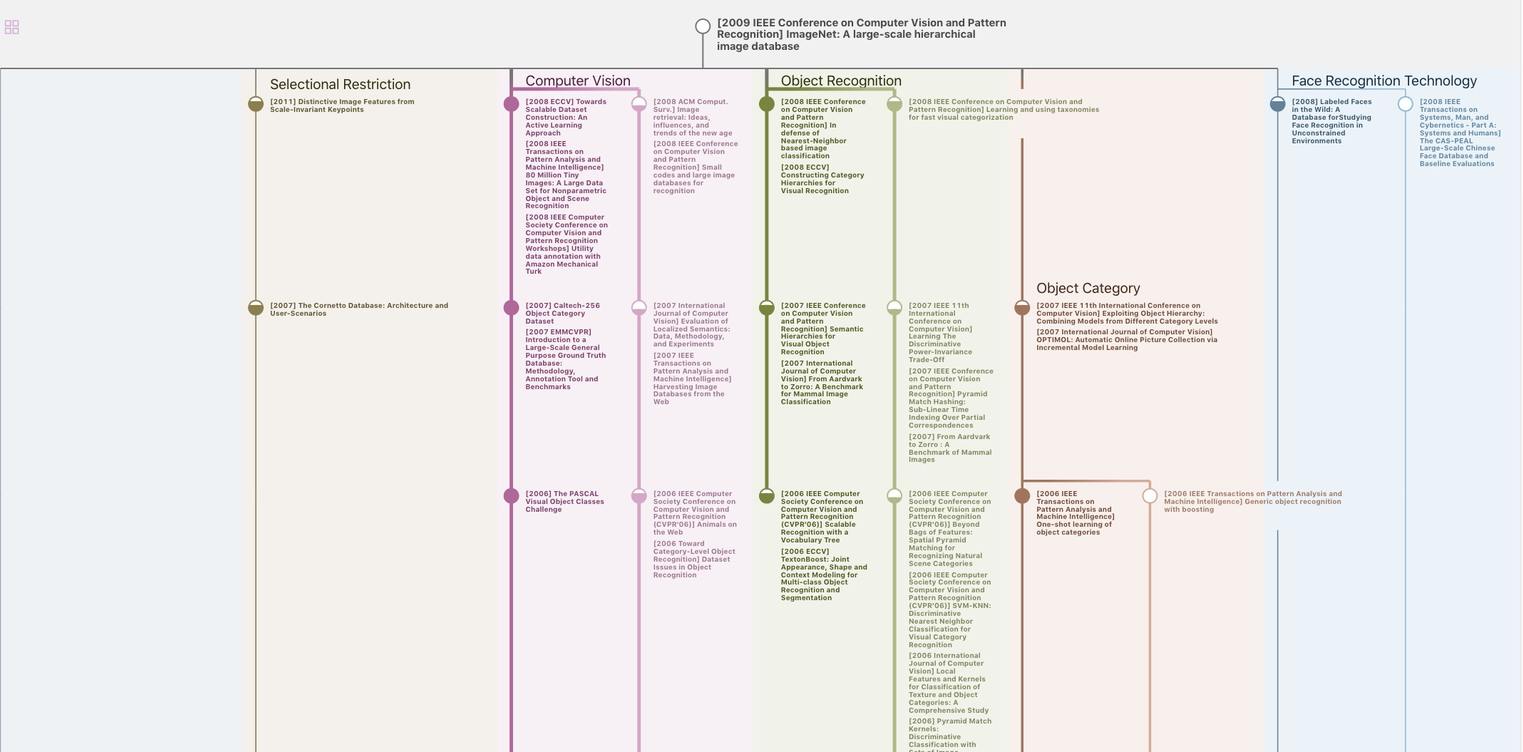
生成溯源树,研究论文发展脉络
Chat Paper
正在生成论文摘要