RAMMM: A Rapid Attention-Based Multimodal Modification Model for Massive Image Generation.
International Conference on Parallel and Distributed Systems(2023)
摘要
Nowadays, deep learning (DL) techniques have found extensive applications in the Internet of Things (IoT) community, such as autonomous driving and medical diagnosis. Despite these successful implementations, elevated data collection expenses, data confidentiality concerns, and privacy issues all contribute to the complexity and costliness of data acquisition in DL-based image processing. Image modification methods based on diffusion models can subtly modify images to generate new images with similar content to the original image but with unique differences, providing a powerful tool for data augmentation. Nonetheless, diffusion models exhibit constraints in cross-modal image modification, such as sensitivity to prompt, model complexity, and user-friendliness. To address these issues, this paper proposes a rapid attention-based multimodal modification model (RAMMM) to facilitate straightforward and efficient text-guided image modification. Our proposed RAMMM primarily enhances the text processing and image generation procedures via an attention mechanism, thus improving the ability in capturing semantic and contextual information within the text. Consequently, RAMMM excels in generating high-quality sample images aligned with the description provided for the modified text. In addition, by utilizing text-guided image modification, RAMMM is capable of generating batches of image samples systematically to augment the dataset size. Evaluation results demonstrate that RAMMM can improve the performance and generalization capabilities of diffusion models by enhancing both the quality and quantity of the dataset. When employed for data augmentation on the CIFAR10 dataset, RAMMM achieves a 1.5% increase in target recognition accuracy.
更多查看译文
关键词
deep learning,data augmentation,image modification,attention mechanism
AI 理解论文
溯源树
样例
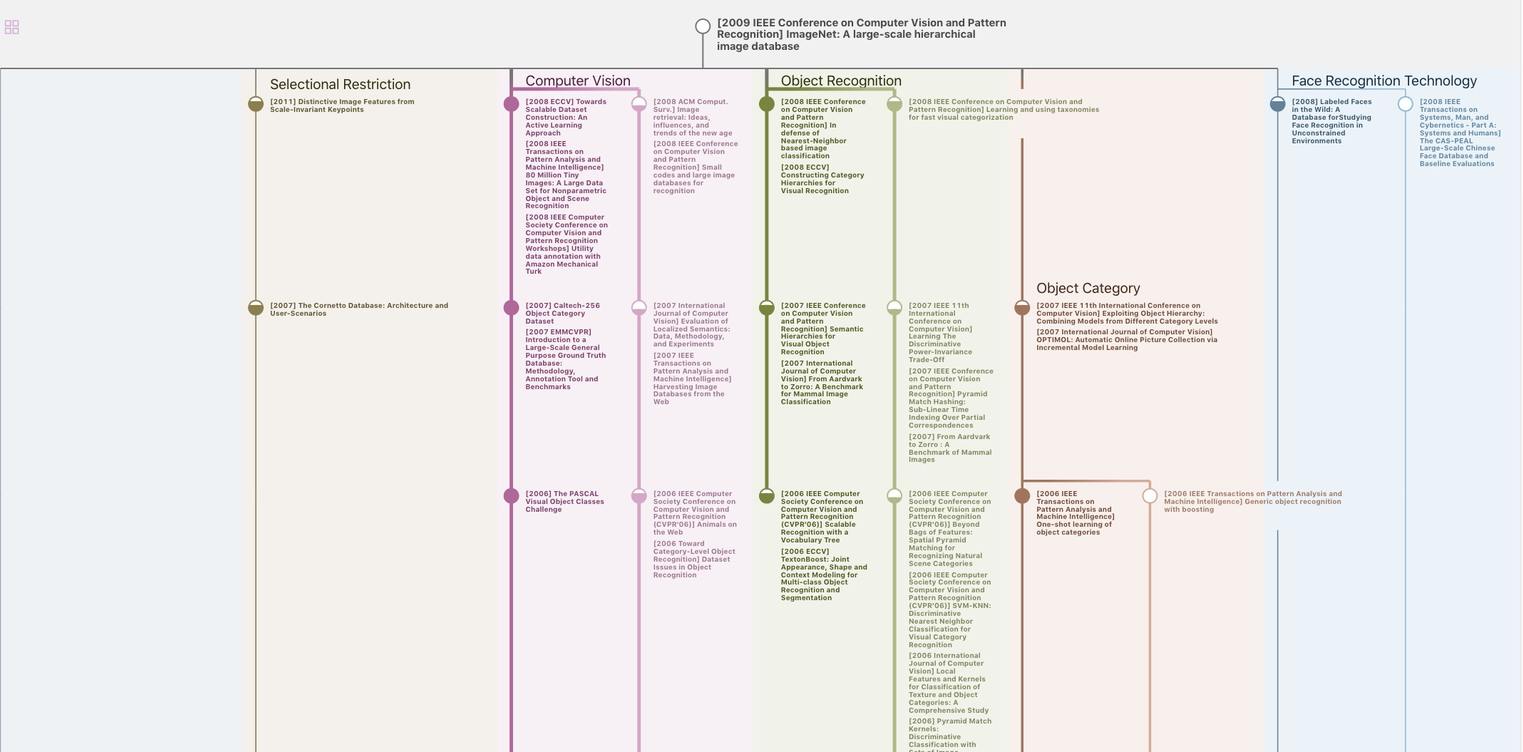
生成溯源树,研究论文发展脉络
Chat Paper
正在生成论文摘要