Spatiotemporal Enhanced Adversarial Network for Precipitation Nowcasting
IEEE Journal of Selected Topics in Applied Earth Observations and Remote Sensing(2024)
摘要
Precipitation nowcasting is a critical aspect of meteorological services, which helps people make reasonable arrangements. Nowadays the methods based on Recurrent Neural Networks (RNNs) are widely employed as the primary solution for precipitation nowcasting. However, the predictive unit of these methods has a narrow temporal receptive field that fails to provide sufficient temporal variation information for accurate prediction of the subsequent frame. Additionally, they do not adequately model the spatial deformation of visual appearance, which leads to the predicted frames lack of fine-grained spatial appearances. To address these deficiencies, we propose a Spatiotemporal Enhanced Adversarial Network (STEAN), a deep learning model for high-resolution precipitation nowcasting. STEAN incorporates a Feature Extraction Module (FEM) and an Adaptive Fusion Module (AFM) to refine the spatial appearance of prediction results. Further, it leverages a specialized Halo Attention Spatiotemporal Long Short-Term Memory (HAST-LSTM) unit to model temporal variation information. In order to improve the realism of the predicted sequences, STEAN is combined with a temporal discriminator during the training stage to reduce the blur of prediction results caused by the loss function. STEAN has been assessed on the Moving MNIST, KNMI, and CIKM datasets and the experimental results show that its prediction performance is superior to several other state-of-the-art models.
更多查看译文
关键词
Precipitation nowcasting,deep learning,spatiotemporal enhancement,generative adversarial networks,recurrent neural networks
AI 理解论文
溯源树
样例
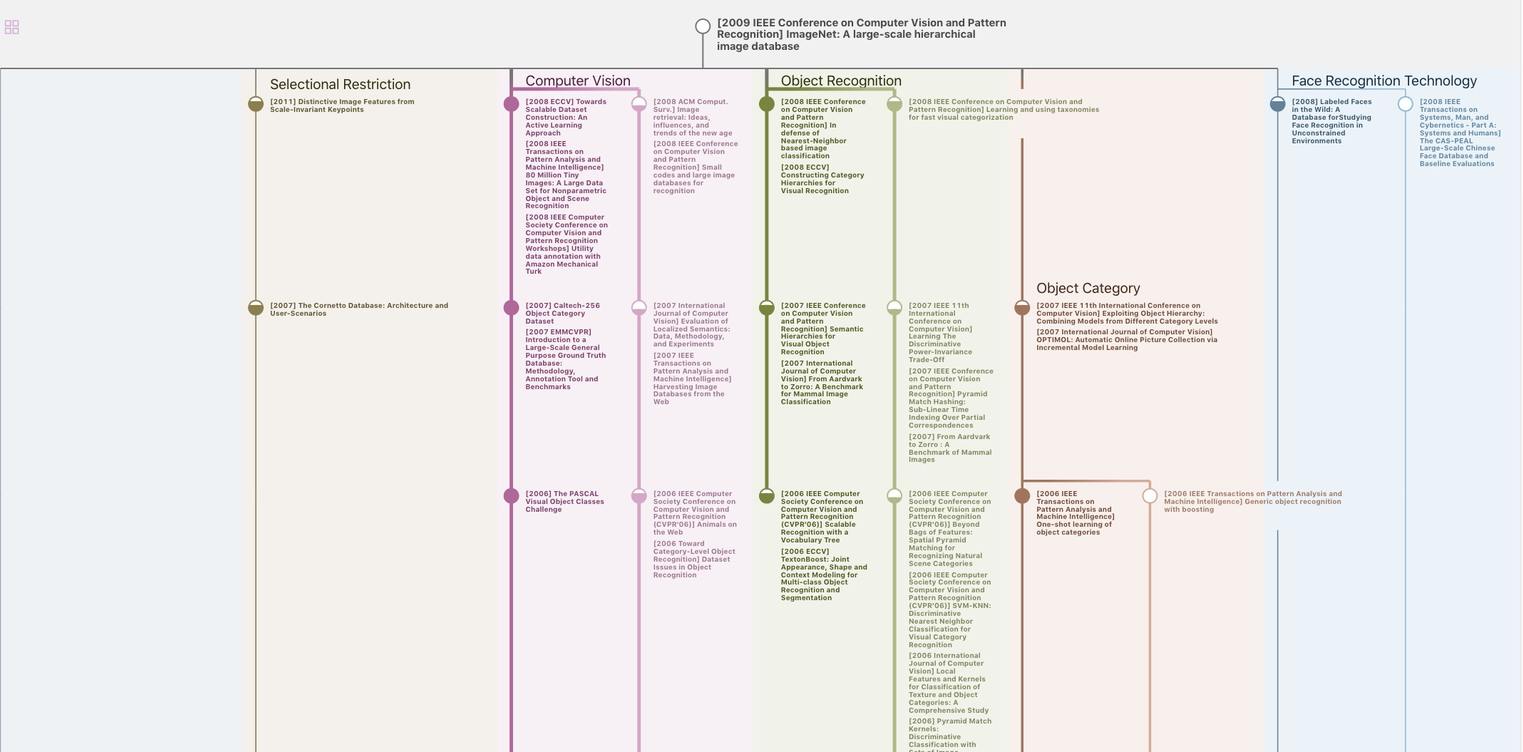
生成溯源树,研究论文发展脉络
Chat Paper
正在生成论文摘要