Application of nonparametric quantifiers for online handwritten signature verification: A statistical learning approach
STATISTICAL ANALYSIS AND DATA MINING(2024)
摘要
This work explores the use of nonparametric quantifiers in the signature verification problem of handwritten signatures. We used the MCYT-100 (MCYT Fingerprint subcorpus) database, widely used in signature verification problems. The discrete-time sequence positions in the x -axis and y-axis provided in the database are preprocessed, and time causal information based on nonparametric quantifiers such as entropy, complexity, Fisher information, and trend are employed. The study also proposes to evaluate these quantifiers with the time series obtained, applying the first and second derivatives of each sequence position to evaluate the dynamic behavior by looking at their velocity and acceleration regimes, respectively. The signatures in the MCYT-100 database are classified via Logistic Regression, Support Vector Machines (SVM), Random Forest, and Extreme Gradient Boosting (XGBoost). The quantifiers were used as input features to train the classifiers. To assess the ability and impact of nonparametric quantifiers to distinguish forgery and genuine signatures, we used variable selection criteria, such as: information gain, analysis of variance, and variance inflation factor. The performance of classifiers was evaluated by measures of classification error such as specificity and area under the curve. The results show that the SVM and XGBoost classifiers present the best performance.
更多查看译文
关键词
classification,feature selection,nonparametric quantifier,signature verification,statistical learning
AI 理解论文
溯源树
样例
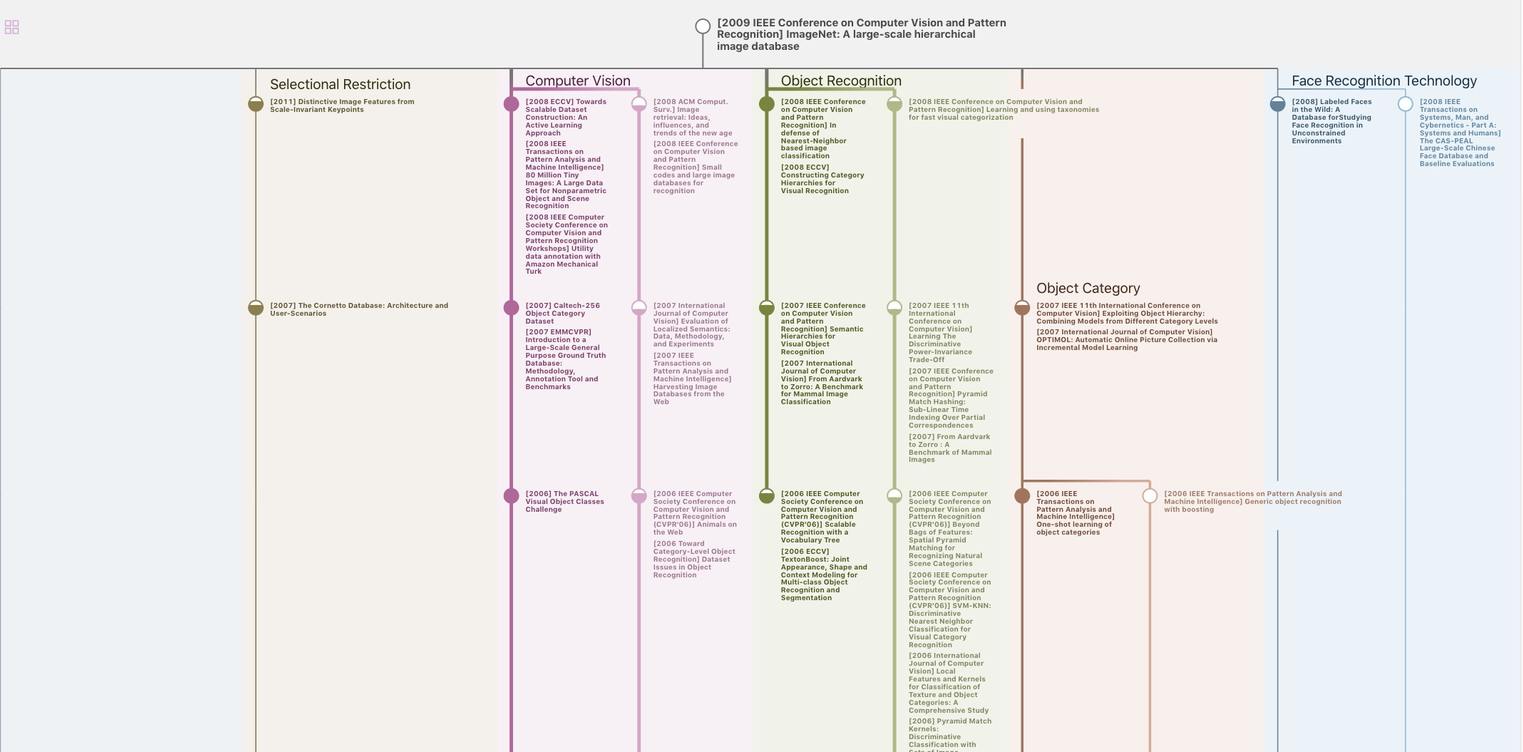
生成溯源树,研究论文发展脉络
Chat Paper
正在生成论文摘要