Development and validation of an interpretable model integrating multimodal information for improving ovarian cancer diagnosis
NATURE COMMUNICATIONS(2024)
摘要
Ovarian cancer, a group of heterogeneous diseases, presents with extensive characteristics with the highest mortality among gynecological malignancies. Accurate and early diagnosis of ovarian cancer is of great significance. Here, we present OvcaFinder, an interpretable model constructed from ultrasound images-based deep learning (DL) predictions, Ovarian-Adnexal Reporting and Data System scores from radiologists, and routine clinical variables. OvcaFinder outperforms the clinical model and the DL model with area under the curves (AUCs) of 0.978, and 0.947 in the internal and external test datasets, respectively. OvcaFinder assistance led to improved AUCs of radiologists and inter-reader agreement. The average AUCs were improved from 0.927 to 0.977 and from 0.904 to 0.941, and the false positive rates were decreased by 13.4% and 8.3% in the internal and external test datasets, respectively. This highlights the potential of OvcaFinder to improve the diagnostic accuracy, and consistency of radiologists in identifying ovarian cancer. Ovarian cancer diagnosis can be complex and can be improved through integration of multimodal data. Here, the authors develop OvcaFinder which can significantly outperform clinical models using ultrasound images and known clinical characteristics.
更多查看译文
AI 理解论文
溯源树
样例
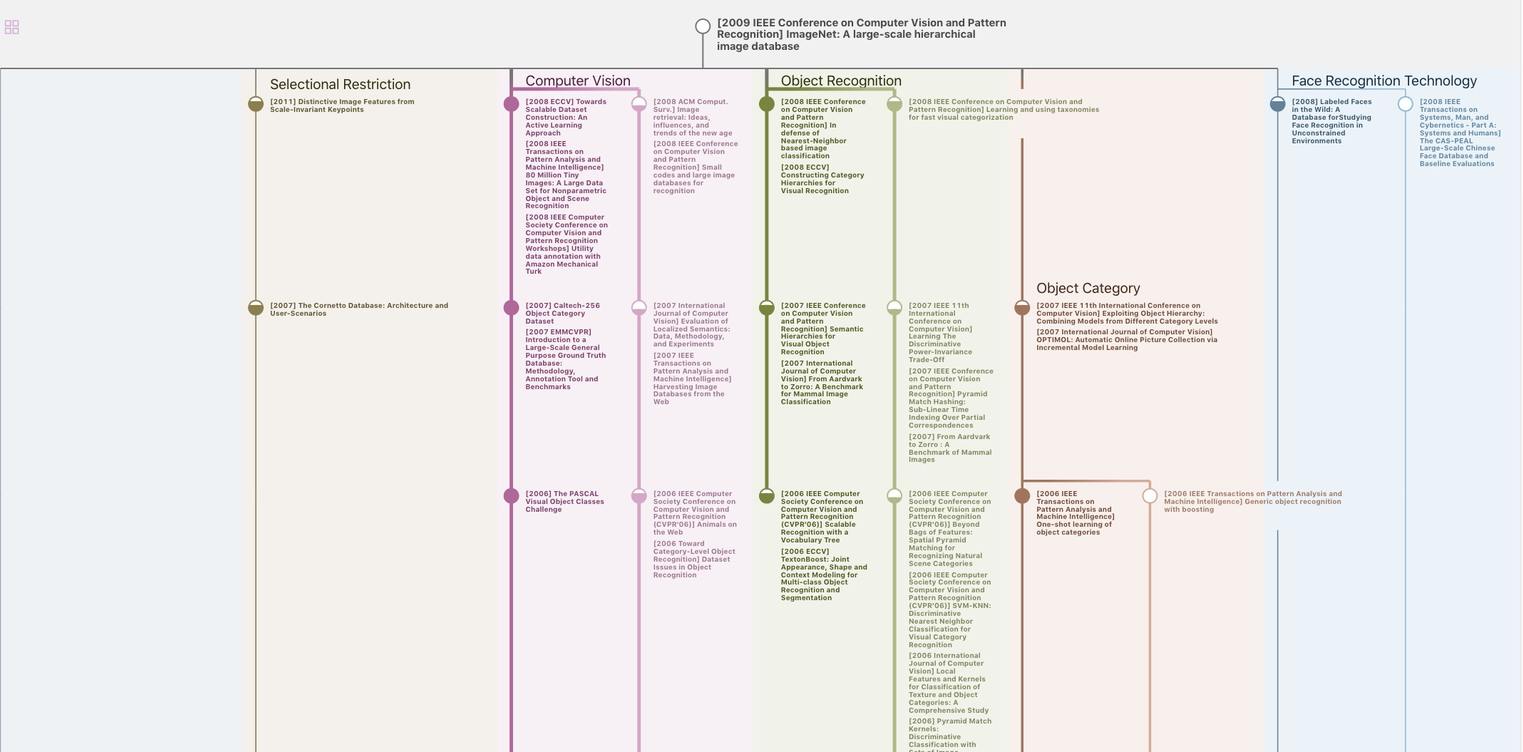
生成溯源树,研究论文发展脉络
Chat Paper
正在生成论文摘要