Classifying breast cancer subtypes on multi-omics data via sparse canonical correlation analysis and deep learning
BMC Bioinformatics(2024)
摘要
Classifying breast cancer subtypes is crucial for clinical diagnosis and treatment. However, the early symptoms of breast cancer may not be apparent. Rapid advances in high-throughput sequencing technology have led to generating large number of multi-omics biological data. Leveraging and integrating the available multi-omics data can effectively enhance the accuracy of identifying breast cancer subtypes. However, few efforts focus on identifying the associations of different omics data to predict the breast cancer subtypes. In this paper, we propose a differential sparse canonical correlation analysis network (DSCCN) for classifying the breast cancer subtypes. DSCCN performs differential analysis on multi-omics expression data to identify differentially expressed (DE) genes and adopts sparse canonical correlation analysis (SCCA) to mine highly correlated features between multi-omics DE-genes. Meanwhile, DSCCN uses multi-task deep learning neural network separately to train the correlated DE-genes to predict breast cancer subtypes, which spontaneously tackle the data heterogeneity problem in integrating multi-omics data. The experimental results show that by mining the associations among multi-omics data, DSCCN is more capable of accurately classifying breast cancer subtypes than the existing methods.
更多查看译文
关键词
Multi-omics data integration,Breast cancer subtypes,Sparse canonical correlation analysis,Deep neural network
AI 理解论文
溯源树
样例
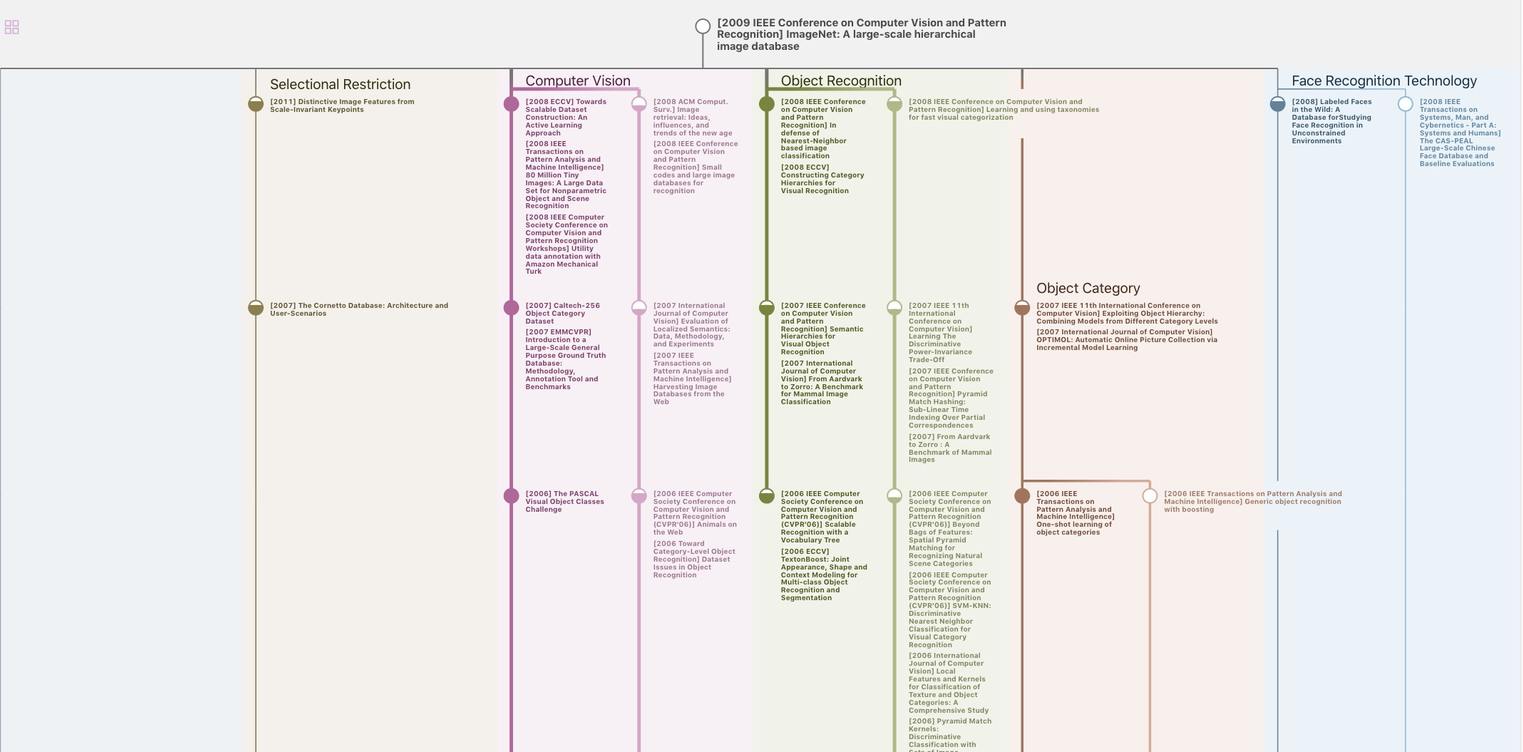
生成溯源树,研究论文发展脉络
Chat Paper
正在生成论文摘要