DS-MSFF-Net: Dual-path self-attention multi-scale feature fusion network for CT image segmentation
Applied Intelligence(2024)
摘要
Computed tomography (CT) is an important technique that is widely used in disease screening and diagnosis. In order to assist doctors in diagnosis and treatment plans, an efficient and accurate automatic image segmentation technology is urgently needed. CT images of different lesions always have problems such as different resolutions, different numbers of lesions, and inconspicuous contrast between lesions and background areas, which brings considerable challenges to the automated segmentation process. To this end, we propose a dual-path self-attention multi-scale feature fusion network (DS-MSFF-Net) that fuses self-attention mechanism and dilated convolution. It is worth noting that this network includes two parallel branch paths, which enables it to extract long-range semantic feature information effectively while extracting detailed feature information of CT images. Additionally, a novel feature extraction module is designed to focus limited learning resources on low-resolution high-order semantic feature maps, which can improve the segmentation accuracy without significant additional computational overhead. We extensively evaluate our method on the LIDC-IDRI lung nodule segmentation dataset and the LiTS2017 liver segmentation dataset, which outperforms other recent state-of-the-art methods on various CT image segmentation tasks.
更多查看译文
关键词
Dual-path,CT,Self-attention,MSFF,Image segmentation
AI 理解论文
溯源树
样例
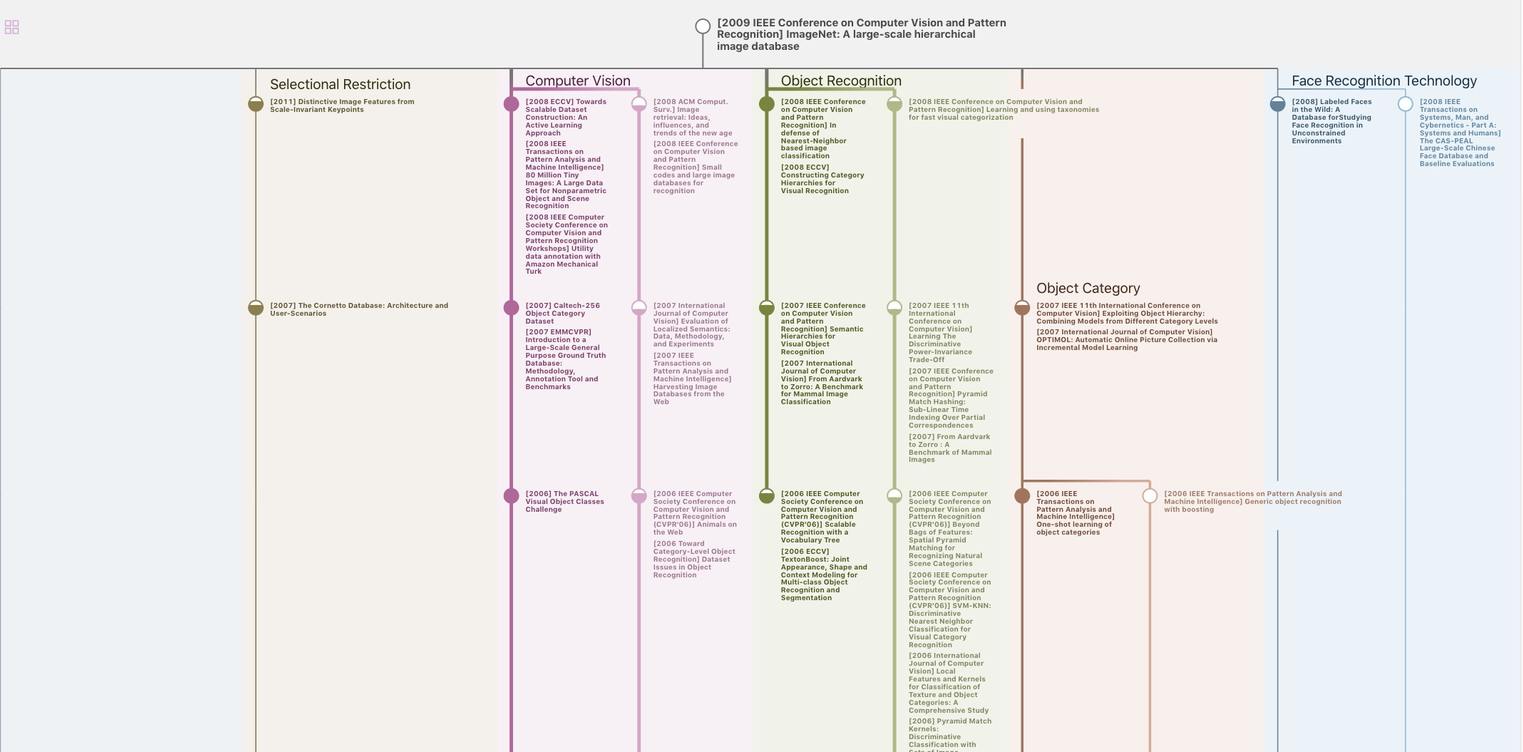
生成溯源树,研究论文发展脉络
Chat Paper
正在生成论文摘要