Accelerating preconditioned ADMM via degenerate proximal point mappings
arxiv(2024)
摘要
In this paper, we aim to accelerate a preconditioned alternating direction
method of multipliers (pADMM), whose proximal terms are convex quadratic
functions, for solving linearly constrained convex optimization problems. To
achieve this, we first reformulate the pADMM into a form of proximal point
method (PPM) with a positive semidefinite preconditioner which can be
degenerate due to the lack of strong convexity of the proximal terms in the
pADMM. Then we accelerate the pADMM by accelerating the reformulated degenerate
PPM (dPPM). Specifically, we first propose an accelerated dPPM by integrating
the Halpern iteration and the fast Krasnosel'skiĭ-Mann iteration into it,
achieving asymptotic o(1/k) and non-asymptotic O(1/k) convergence rates.
Subsequently, building upon the accelerated dPPM, we develop an accelerated
pADMM algorithm that exhibits both asymptotic o(1/k) and non-asymptotic
O(1/k) nonergodic convergence rates concerning the Karush-Kuhn-Tucker
residual and the primal objective function value gap. Preliminary numerical
experiments validate the theoretical findings, demonstrating that the
accelerated pADMM outperforms the pADMM in solving convex quadratic programming
problems.
更多查看译文
AI 理解论文
溯源树
样例
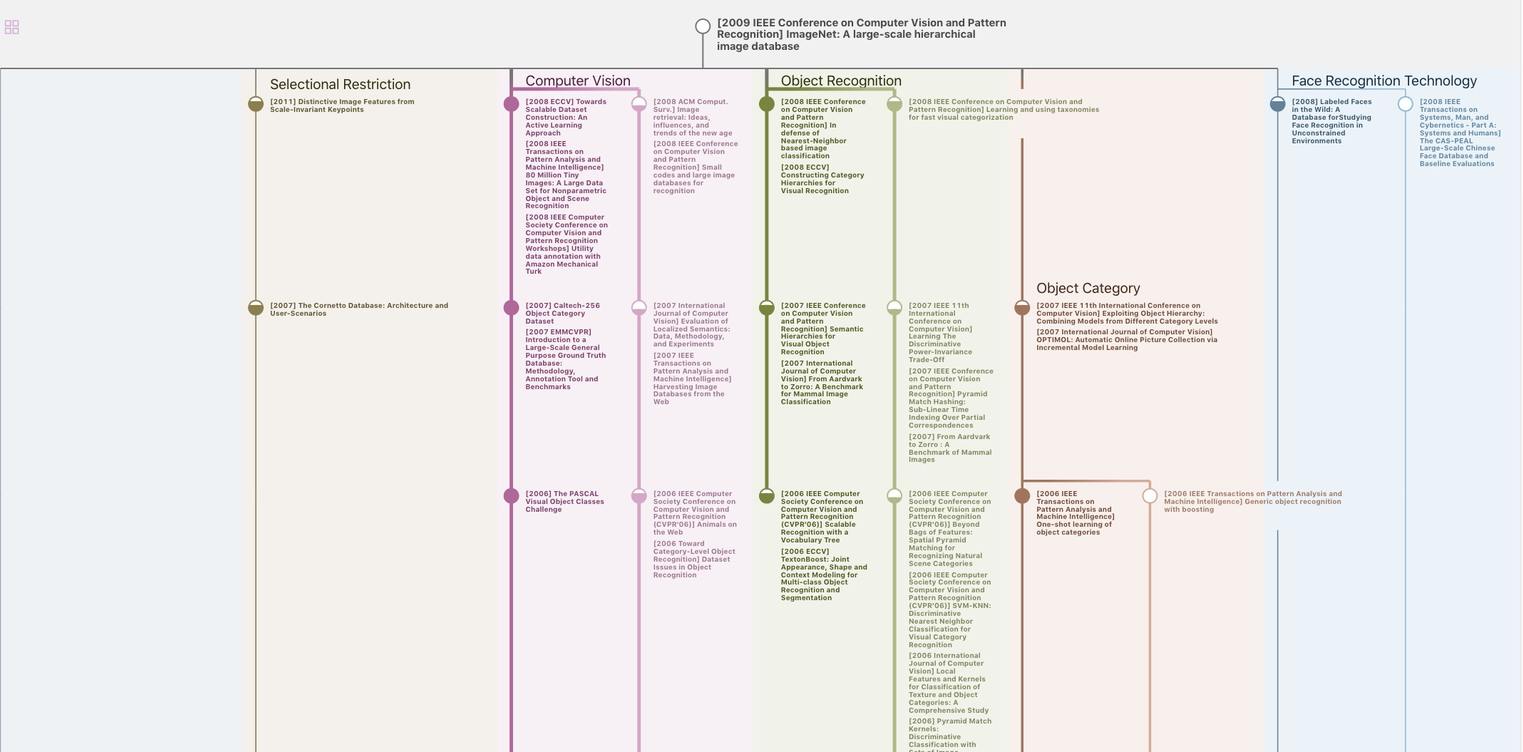
生成溯源树,研究论文发展脉络
Chat Paper
正在生成论文摘要