Detection of subclinical atherosclerosis by image-based deep learning on chest x-ray
CoRR(2024)
摘要
Aims. To develop a deep-learning based system for recognition of subclinical
atherosclerosis on a plain frontal chest x-ray. Methods and Results. A
deep-learning algorithm to predict coronary artery calcium (CAC) score (the
AI-CAC model) was developed on 460 chest x-ray (80
internal validation cohort) of primary prevention patients (58.4
age 63 [51-74] years) with available paired chest x-ray and chest computed
tomography (CT) indicated for any clinical reason and performed within 3
months. The CAC score calculated on chest CT was used as ground truth. The
model was validated on an temporally-independent cohort of 90 patients from the
same institution (external validation). The diagnostic accuracy of the AI-CAC
model assessed by the area under the curve (AUC) was the primary outcome.
Overall, median AI-CAC score was 35 (0-388) and 28.9
AUC of the AI-CAC model to identify a CAC>0 was 0.90 in the internal validation
cohort and 0.77 in the external validation cohort. Sensitivity was consistently
above 92
AI-CAC=0, a single ASCVD event occurred, after 4.3 years. Patients with
AI-CAC>0 had significantly higher Kaplan Meier estimates for ASCVD events
(13.5
accurately detect subclinical atherosclerosis on chest x-ray with elevated
sensitivity, and to predict ASCVD events with elevated negative predictive
value. Adoption of the AI-CAC model to refine CV risk stratification or as an
opportunistic screening tool requires prospective evaluation.
更多查看译文
AI 理解论文
溯源树
样例
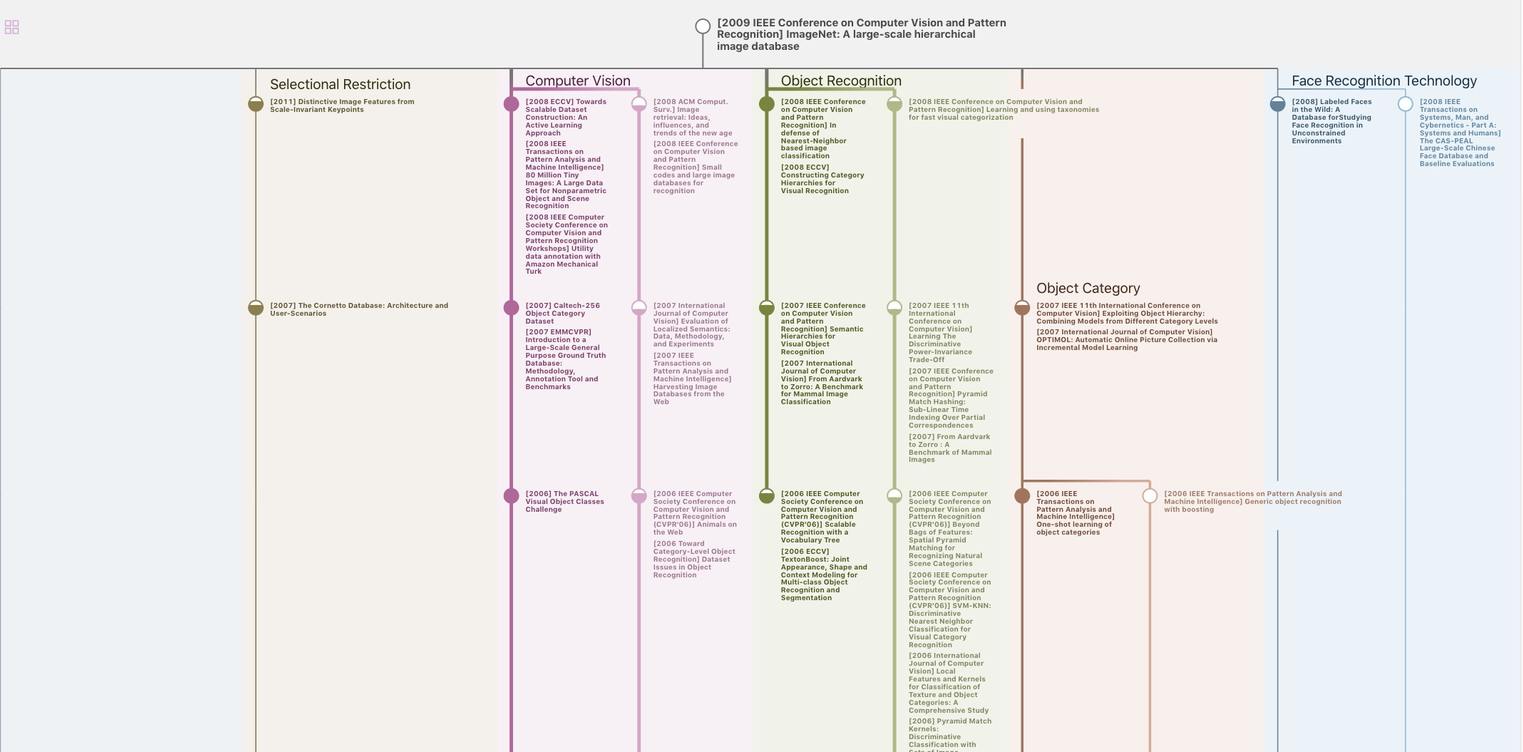
生成溯源树,研究论文发展脉络
Chat Paper
正在生成论文摘要