Integrating Misidentification and OOD Detection for Reliable Fault Diagnosis of High-Speed Train Bogie
IEEE TRANSACTIONS ON INTELLIGENT TRANSPORTATION SYSTEMS(2024)
摘要
Developing a trustworthy framework for intelligent fault diagnosis (IFD) of machines has two major challenges: confidently recognizing known faults and precisely detecting novel faults. However, current IFD frameworks are typically based on the closed-world assumption and tackle the two issues independently, making it hard to meet the expectations of reliable diagnosis in complicated working situations. In this paper, a Probabilistic framework for Mechanical fault diagnosis (ProMo) is proposed to integrate misidentification and out-of-distribution (OOD) detection for high-speed train bogie. ProMo uses variational parameters to capture uncertainties at both the feature and prediction levels, in this way the misclassified and OOD samples with high predictive uncertainty are differentiated. To begin, a Bayesian deep neural network with a hierarchical classifier is built, offering diverse predictions and enabling ensemble uncertainty estimate. Then, a probabilistic null space analysis technique for post hoc OOD detection is presented, in which the magnitude of feature projections indicates the OOD-ness. Additionally, a novel metric assessing classification correctness and prediction reliability simultaneously is proposed. Extensive experiments revealed that ProMo outperforms state-of-the-art methods and achieves reliable fault diagnosis, even in the presence of covariate shift in monitoring data.
更多查看译文
关键词
Uncertainty,Reliability,Fault diagnosis,Bayes methods,Probabilistic logic,Predictive models,Data models,high-speed train bogie,Bayesian learning,out-of-distribution detection,uncertainty estimation
AI 理解论文
溯源树
样例
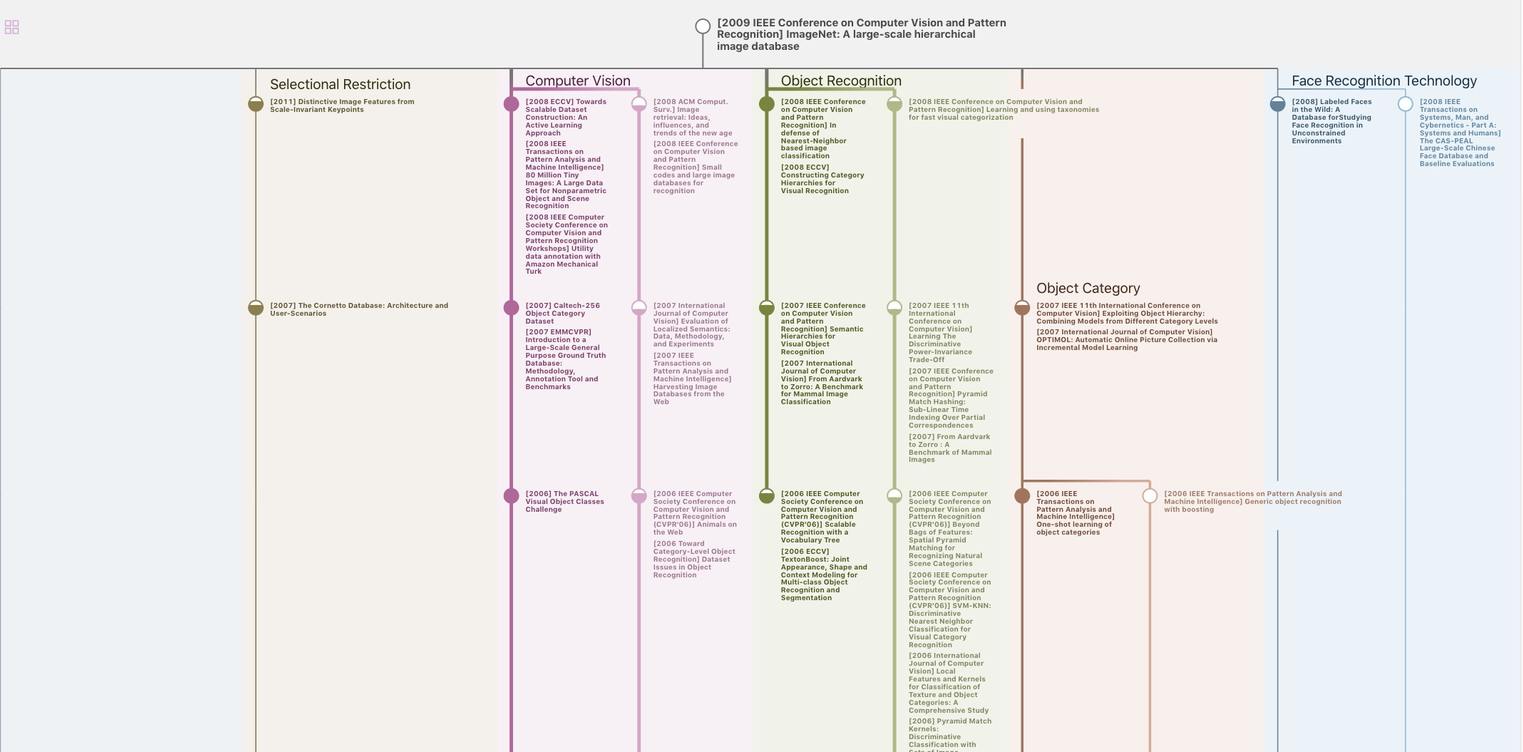
生成溯源树,研究论文发展脉络
Chat Paper
正在生成论文摘要