A Continuous Volatility Forecasting Model Based on Neural Differential Equations and Scale-Similarity
IEEE TRANSACTIONS ON NEURAL NETWORKS AND LEARNING SYSTEMS(2024)
摘要
Volatility forecasting is a problem in finance that attracts the attention of both academia and industry. While existing approaches typically utilize a discrete-time latent process that governs the volatility to forecast its future level, volatility is considered to evolve continuously, which makes discrete-time modeling inevitably lose some critical information about the evolution of volatility. In this article, a novel neural-network-based model, Continuous Volatility Forecasting Model, CVFM is proposed to tackle this problem. First, CVFM introduces a continuous-time latent process, whose evolution is modeled with neural differential equations (NDEs), to govern volatility, which effectively captures the continuous evolutionary behavior of volatility in a data-driven way. Second, a scale-similarity-based mechanism is designed to calibrate the evolution equation of the latent process with real-world observations in the absence of high-frequency data. CVFM is tested on six real-world stock index datasets. The main experimental results show that CVFM can significantly outperform existing models in terms of both forecasting accuracy and high-volatility recognition.
更多查看译文
关键词
Mathematical models,Predictive models,Differential equations,Stochastic processes,Forecasting,Finance,Time series analysis,Deep learning,neural differential equation (NDE),time-series modeling,volatility forecasting
AI 理解论文
溯源树
样例
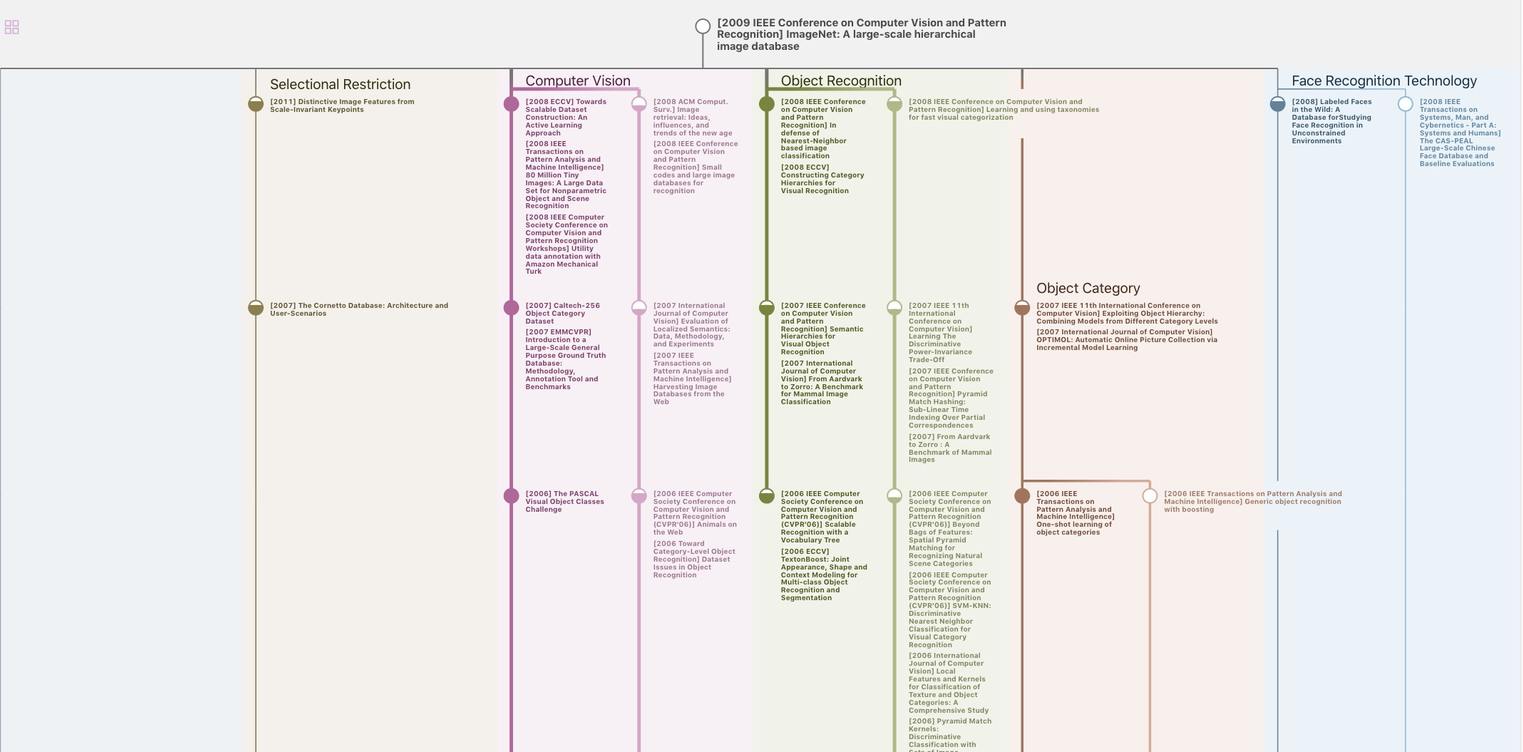
生成溯源树,研究论文发展脉络
Chat Paper
正在生成论文摘要