CompoHyDen: Hyperspectral Image Restoration via Non-Convex Component-wise Minimization
IEEE Journal of Selected Topics in Applied Earth Observations and Remote Sensing(2024)
摘要
In this paper, we propose a variational approach for estimating the clean hyperspectral images (HSI) that are corrupted by the combined effect of Gaussian noise, impulse noise, stripes, and deadlines. Successful removal of noise from the corrupted observations is essential for subsequent downstream analyses like classification, spectral unmixing, and target tracking. The main contribution of this work is as follows:
Firstly,
an objective function is designed for the joint estimation of clean data and impulse corrupted pixels. A rationale is presented for using
$\ell _{0}-$
norm to estimate the exact sparsity induced by impulse noise.
Secondly,
the problem is reformulated as a multi-convex problem which is solved using proximal projection and alternating minimization.
Thirdly,
to exploit the spatial-spectral similarity, a non-local and vectorized version of total variation (TV) regularization is proposed to estimate the clean data.
Lastly
, a study on the parameter sensitivity analysis empirically validates the convergence of the restoration results under different values of the regularization hyperparameters. The experiments conducted over synthetically corrupted and real HSI data obtained from hyperspectral sensors suggest the potential utility of the proposed methodology (
CompoHyDen
) at a scalable level.
更多查看译文
关键词
Hyperspectral imaging,Gaussian-impulse noise,Non-local total variation,Alternating minimization,Proximal projection
AI 理解论文
溯源树
样例
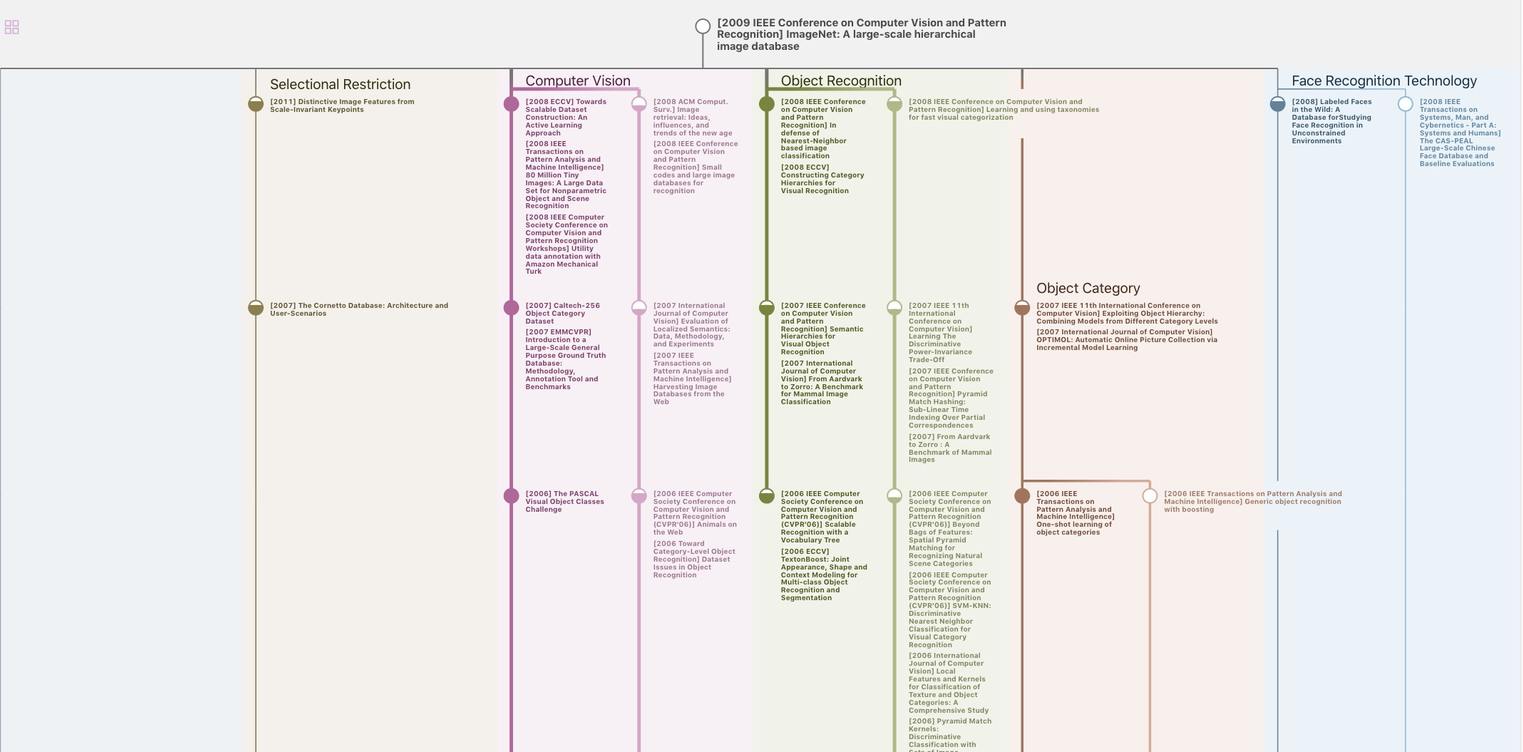
生成溯源树,研究论文发展脉络
Chat Paper
正在生成论文摘要