A Defect Data Compensation Model for Infrared Thermal Imaging Based on Bi-LSTM with Attention Mechanism
JOM(2024)
摘要
The infrared thermal imager presents unique advantages in plate fault detection and prediction. However, during the data collection process, three abnormal conditions can result in anomalies and defects in the temporal data. To address this, a defect data compensation model based on bidirectional LSTM is proposed in this paper. The average gray value (AGV) of the plate in the infrared image is employed to represent the working state of the plate, serialized into time series data. To tackle the abnormality and defect issues in the sequential data, four methods are employed to complete the sequential data. Leveraging the strong correlation among time series data, this paper integrates a bidirectional LSTM with attention mechanism (Bi-AM-LSTM) to establish a defect data compensation model. This model compensates for abnormal and defective data, effectively enhancing the reliability and integrity of time series data. Experimental results demonstrate that the model achieves better training results when using the method of replacing the original value with the B-channel value in the RGB three channel. Moreover, when compared with other models, the proposed model exhibits the most effective data compensation.
更多查看译文
AI 理解论文
溯源树
样例
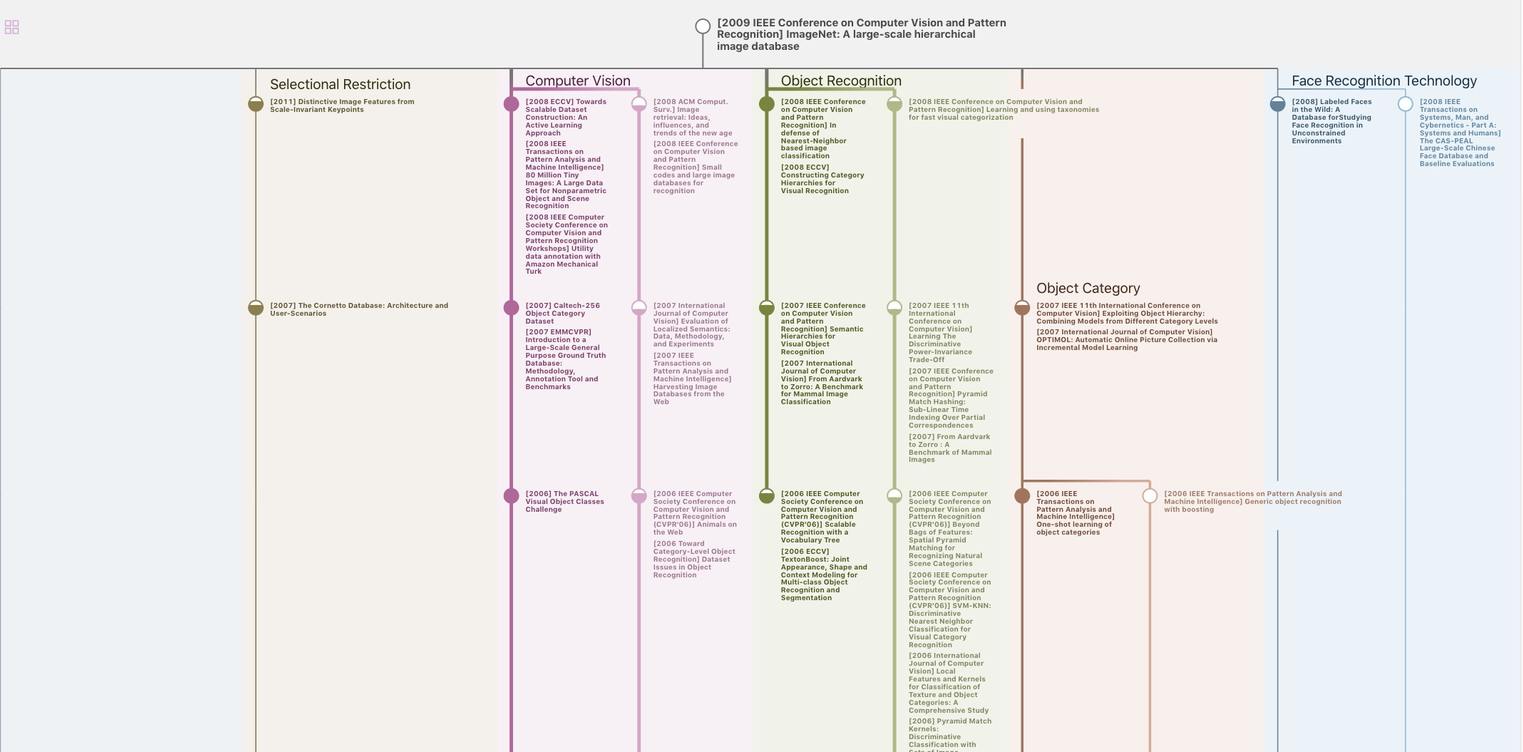
生成溯源树,研究论文发展脉络
Chat Paper
正在生成论文摘要