Rapid estimation of battery state of health using partial electrochemical impedance spectra and interpretable machine learning
Journal of Power Sources(2024)
摘要
The longevity and efficacy of lithium-ion batteries diminish over time, making accurate estimation of their health state essential. Traditional methods, based on long-term charge and discharge data, are limited in speed and data richness. This study introduces a novel approach using partial Electrochemical Impedance Spectroscopy (EIS) and interpretable machine learning for rapid and precise battery health state estimation. Our method selects partial impedance spectra, in contrast to conventional techniques that rely on global impedance spectra, and applies interpretable machine learning. The SHAP (SHapley Additive exPlanations) framework is used to interpret the contribution of each impedance feature, enhancing model transparency and reliability. Comparative evaluations demonstrate that SHAP-enhanced partial impedance spectra provide higher prediction accuracy and are more quickly obtained than global spectra. This innovative application of interpretable machine learning in acquiring partial impedance spectra represents a significant advancement in battery health state estimation. Our findings propose a new paradigm in employing EIS for battery health analysis, promising enhanced precision and speed in practical applications.
更多查看译文
关键词
State of health,Lithium-ion battery,Electrochemical impedance spectroscopy,Interpretable machine learning,SHapley Additive exPlanations
AI 理解论文
溯源树
样例
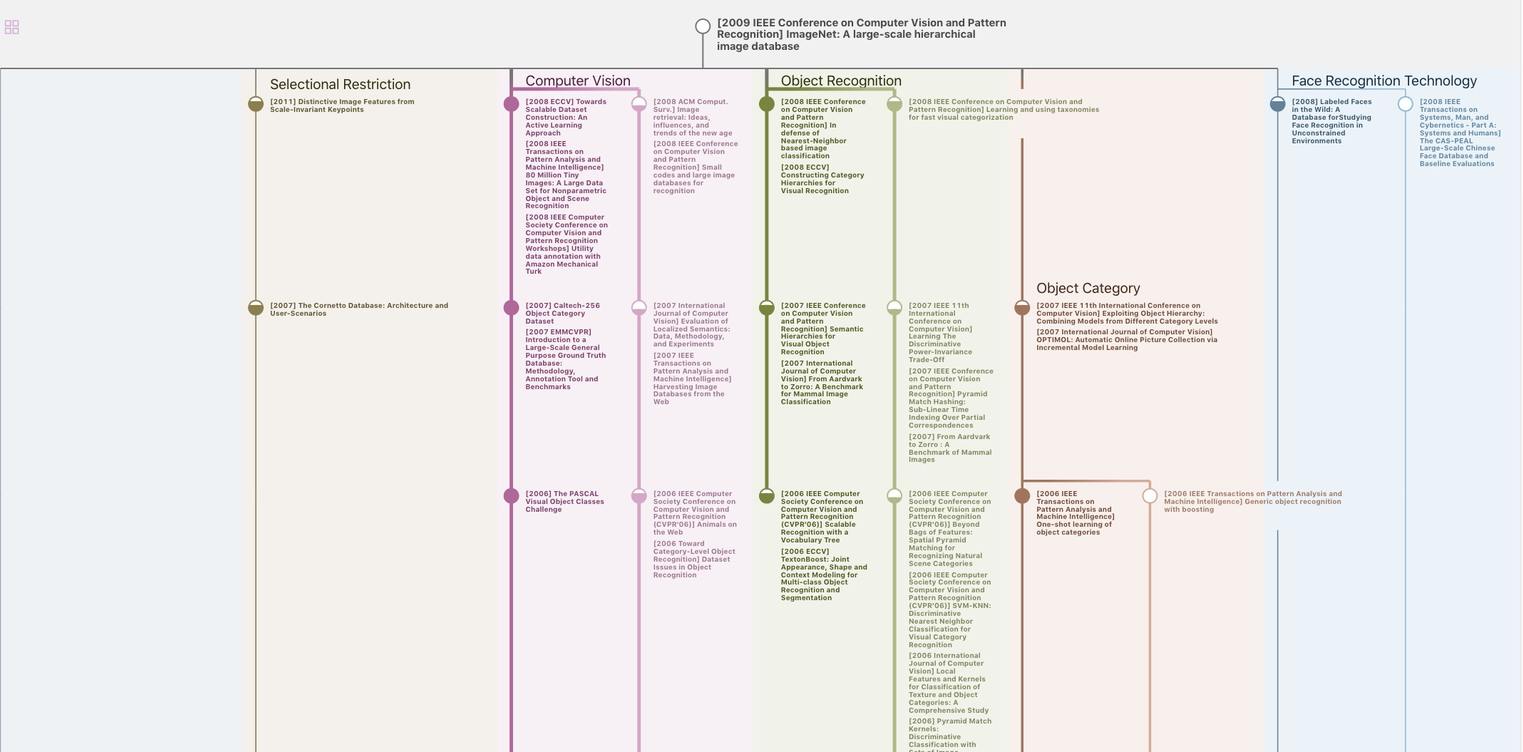
生成溯源树,研究论文发展脉络
Chat Paper
正在生成论文摘要