Nativism and empiricism in artificial intelligence
Philosophical Studies(2024)
摘要
Historically, the dispute between empiricists and nativists in philosophy and cognitive science has concerned human and animal minds (Margolis and Laurence in Philos Stud: An Int J Philos Anal Tradit 165(2): 693-718, 2013, Ritchie in Synthese 199(Suppl 1): 159–176, 2021, Colombo in Synthese 195: 4817–4838, 2018). But recent progress has highlighted how empiricist and nativist concerns arise in the construction of artificial systems (Buckner in From deep learning to rational machines: What the history of philosophy can teach us about the future of artificial intelligence. Oxford University Press.). This paper uses nativism and empiricism to address questions about the nature of artificial intelligence and its trajectory. It begins by defining the nativism/empiricism debate in terms of the generality of a system. Nativist systems have initial states with domain-specific features; empiricist systems have initial states with only domain-general features. With the debate framed in this way, it then explores a variety of arguments for nativism and empiricism in AI. These arguments revolve around two different questions which must be distinguished: whether nativism the only possible approach to developing human-level AI (HLAI); and whether nativism is the most practical approach to developing HLAI. On the first question, it argues that nativism is quite clearly not the only possible approach to developing HLAI, as is sometimes suggested. It argues that existing arguments for the necessity of nativism are unconvincing, because they analogize from poverty of the stimulus arguments about humans, while AIs often have access to much more data than humans. Then it argues that the case of evolution gives us a compelling argument against nativism. On the second, practical question, the paper argues that there is a tradeoff between the advantages of encoding innate machinery directly, and the advantages of evolving or learning it. However, as the past decade has shown, empiricism is a much more viable path to greater capability levels, given the ‘bitter lesson’ (Sutton in Reinforcement Learning: An Introduction. MIT press.) that encoding the ‘correct’ knowledge in AI systems is perennially outperformed by more empiricist methods that leverage large-scale data and computation.
更多查看译文
关键词
Artificial intelligence,Evolution,Nativism,Empiricism,Cognitive science
AI 理解论文
溯源树
样例
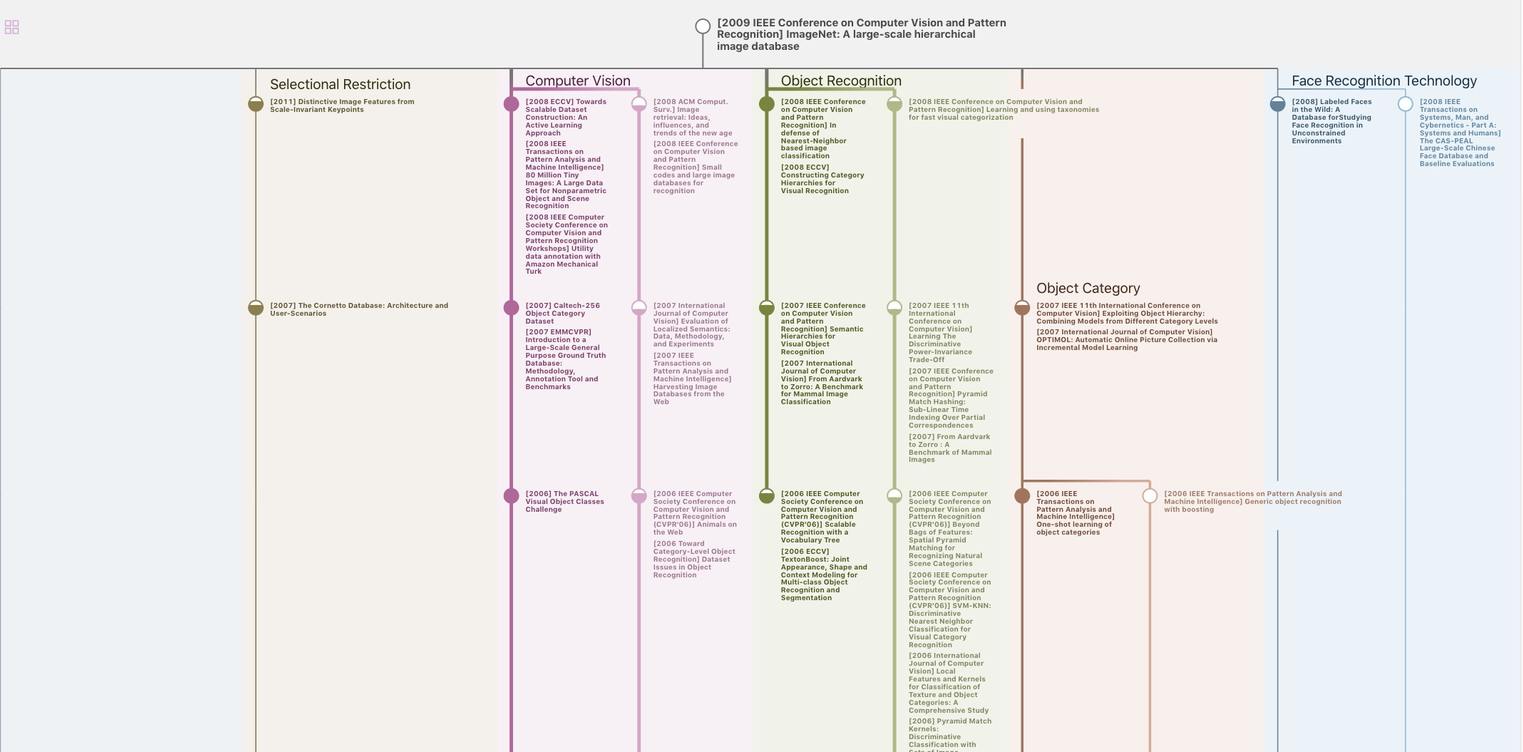
生成溯源树,研究论文发展脉络
Chat Paper
正在生成论文摘要