Quantifying corn LAI using machine learning and UAV multispectral imaging
Precision Agriculture(2024)
摘要
Predicting leaf area index (LAI) is essential for understanding crop growth status and water-fertilizer management. The aim of this study was to evaluate the effectiveness of unmanned aerial vehicle (UAV) multispectral imaging technology in estimating the LAI of corn under different water and fertilizer stress conditions. Monitoring of corn at different growth stages under three water and four fertilizer treatments was conducted using field-based multispectral imaging. Subsequently, a stacking ensemble learning model with primary learners including deep forest (DF), deep neural network, support vector regression, and linear regression was established to predict LAI. Results indicated a significant impact of water and fertilizer stress on LAI. After multi-stage data fusion, the highest Pearson correlation coefficient between vegetation index acquired by UAV and LAI measured on the ground was 0.794. The ensemble learning algorithm utilizing DF as a secondary learner outperformed individual machine learning algorithms, demonstrating prediction metrics with R2 = 0.876, MAE = 351, RMSE = 0.481. The study suggests that ensemble learning algorithms could replace individual machine learning algorithms in constructing LAI prediction models. This research provides theoretical guidance for rapid and precise water and fertilizer management in large experimental fields.
更多查看译文
关键词
Corn,LAI,Unmanned aerial vehicle,Ensemble learning,Water and fertilizer stress
AI 理解论文
溯源树
样例
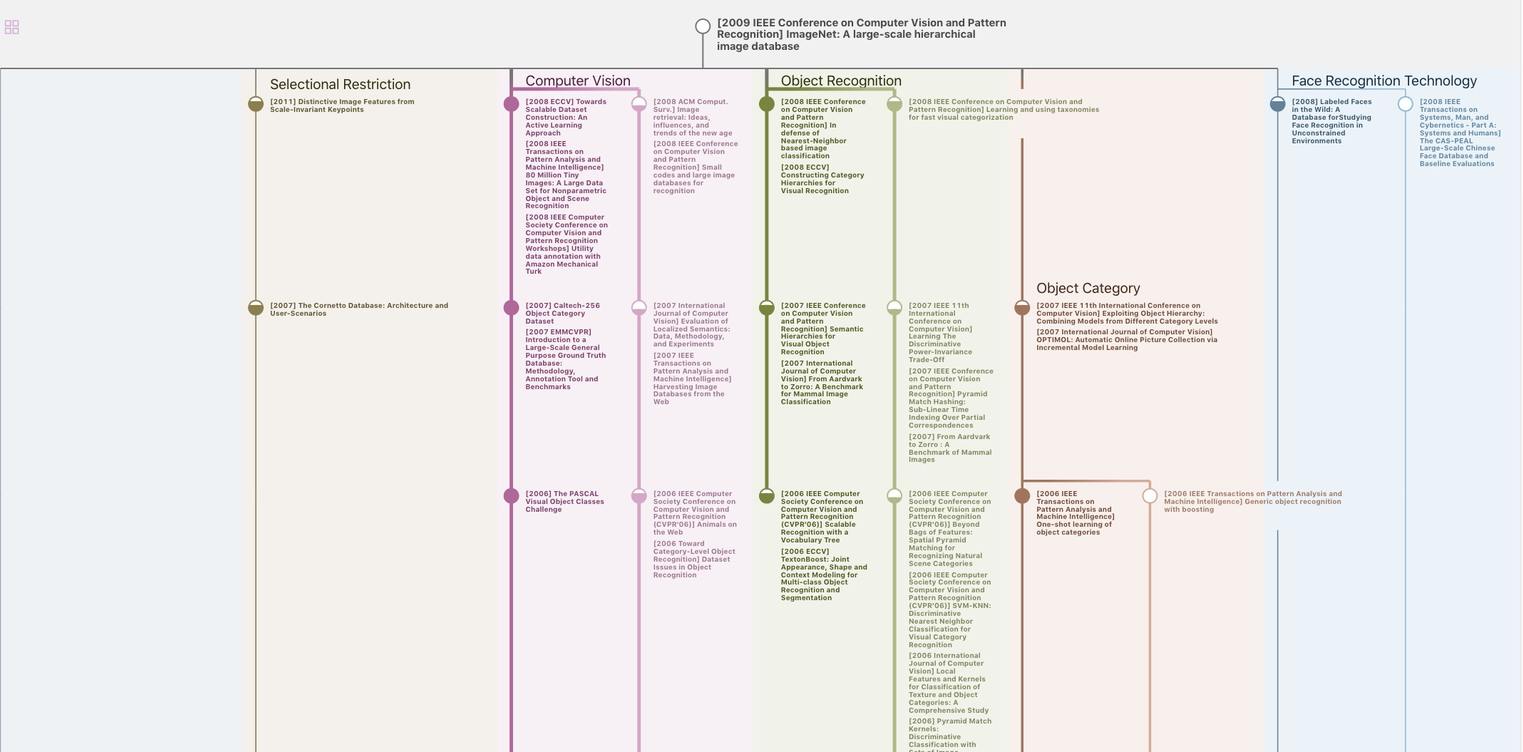
生成溯源树,研究论文发展脉络
Chat Paper
正在生成论文摘要