Environmental monitoring using orbital angular momentum mode decomposition enhanced machine learning
arxiv(2024)
摘要
Atmospheric interaction with light has been an area of fascination for many
researchers over the last century. Environmental conditions, such as
temperature and wind speed, heavily influence the complex and rapidly varying
optical distortions propagating optical fields experience. The continuous
random phase fluctuations commonly make deciphering the exact origins of
specific optical aberrations challenging. The generation of eddies is a major
contributor to atmospheric turbulence, similar in geometric structure to
optical vortices that sit at the centre of OAM beams. Decomposing the received
optical fields into OAM provides a unique spatial similarity that can be used
to analyse turbulent channels. In this work, we present a novel mode
decomposition assisted machine learning approach that reveals trainable
features in the distortions of vortex beams that allow for effective
environmental monitoring. This novel technique can be used reliably with
Support Vector Machine regression models to measure temperature variations of
0.49C and wind speed variations of 0.029 m/s over a 36m experimental turbulent
free-space channels with controllable and verifiable temperature and wind speed
with short 3s measurement. The predictable nature of these findings could
indicate the presence of an underlying physical relationship between
environmental conditions that lead to specific eddy formation and the OAM
spiral spectra.
更多查看译文
AI 理解论文
溯源树
样例
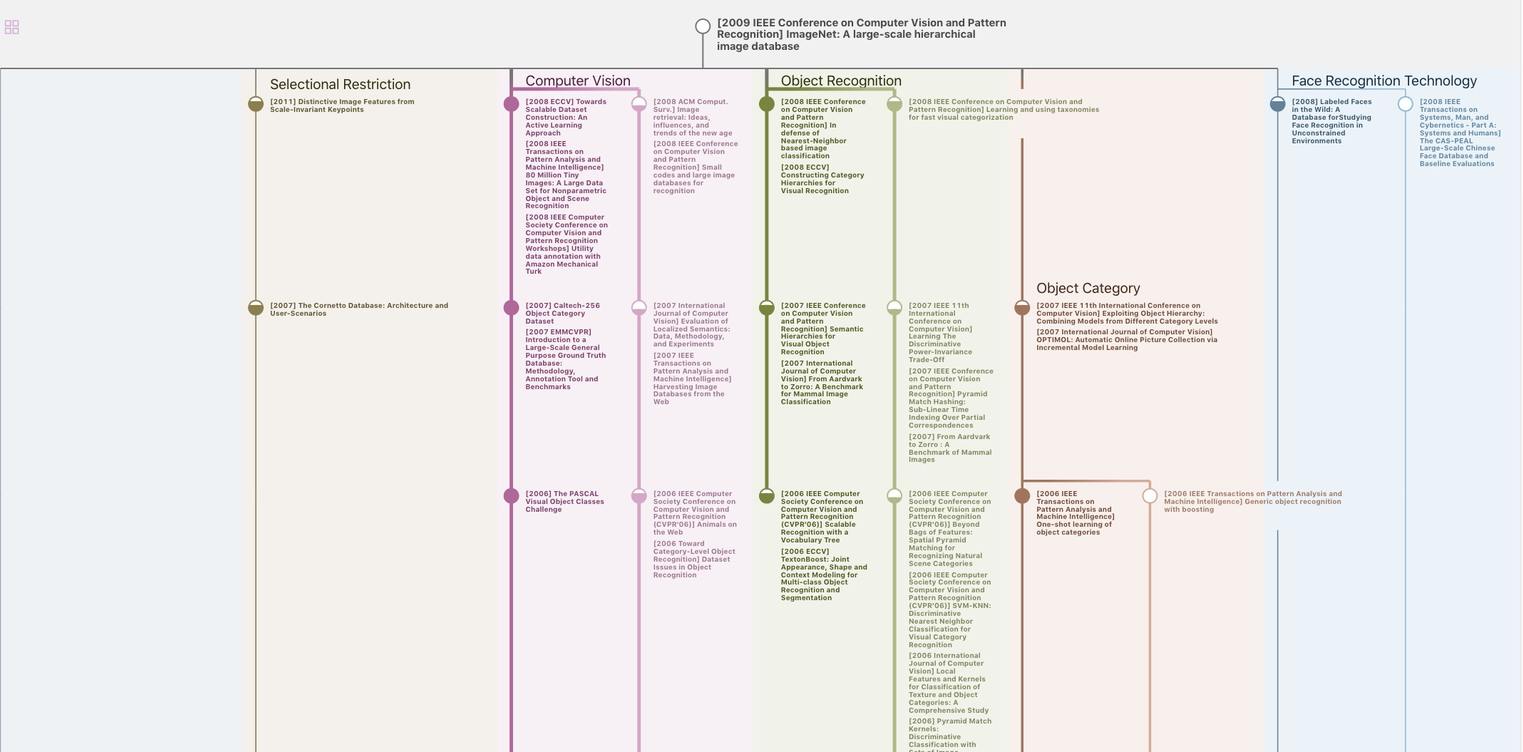
生成溯源树,研究论文发展脉络
Chat Paper
正在生成论文摘要