Study on the Detection Method of COD in Surface Water Based on UV-Vis Spectroscopy
SPECTROSCOPY AND SPECTRAL ANALYSIS(2024)
摘要
Chemical Oxygen Demand (COD) is one of the important indicators of water quality detection, reflecting the organic content of water. Traditional chemical detection methods for COD have disadvantages, such as complicated operation, long waiting times and secondary pollution. UV-Vis spectrometer has been one of the most widely acceptable methods for detecting COD because of its rapidity detection and no pollution. In order to satisfy the requirement of detecting COD of surface water rapidity, real-time and on-line, a model of kernel principal component analysis (KPCA) combined with particle swarm optimization extreme learning machine (PSO-ELM) was developed for COD prediction of surface water based on UV-Vis spectrometer. Savitzky-Golay filtering was employed to smooth the spectrum. The integral spectrum was substituted for the processed spectrum to decrease the impact of fluctuations. In the meantime, spectrum normalisation was used to eliminate the impact caused by different dimensions of spectrum data. The KPAC algorithm was used to compress the whole spectrum into 5 features, effectively solving the spectral information redundancy problem. PSO algorithm was used to optimize the weight and bias of ELM, which improved the model's accuracy. 217 surface water samples, such as rivers, Yangtze River, lakes and reservoirs, were randomly divided into training sets and test sets according to 7:3, and modeling tests were conducted. The R-squared (R-2) of the training set was 0.930 2, the root mean square error (RMSE) of the training set was 0.363 0 mg.L-1, the R-squared (R-2) of the test set was 0.931 9, and the root mean square error (RMSE) of test set was 0.400 7 mg.L-1. In order to verify the improvement of the KPCA based on the full spectrum compression method, data Compression algorithms such as principal component analysis (PCA), successive projection algorithm (SPA) and Lassoregression (LASSO) were compared. The RMSE of PCA-PSO-ELM model, SPA-PSO-ELM model and LASSO-PSO-ELM model was 0.715 1, 0.473 7 and 0.412 6 mg.L-1, respectively. It was shown that the results of the KPCA-PSO-ELM model were better than the above three models, and RMSE decreased by 78.46%, 18.22% and 2.97%, showing that KPCA is an efficient spectral dimension reduction algorithm, which can effectively eliminate spectral redundant information and improve the prediction accuracy of the model. The KPCA-PSO-ELM proposed can realize fast and real-time monitoring of COD in surface water, which can provide algorithm reference for the scene of online water quality monitoring of rapid pollution. As a basic chemical oxygen demand detection research, it provides method reference for online monitoring scenarios for chemical oxygen demand.
更多查看译文
关键词
Chemical oxygen demand,UV-Vis spectroscopy,Kernel principal component analysis,Extreme learning machine
AI 理解论文
溯源树
样例
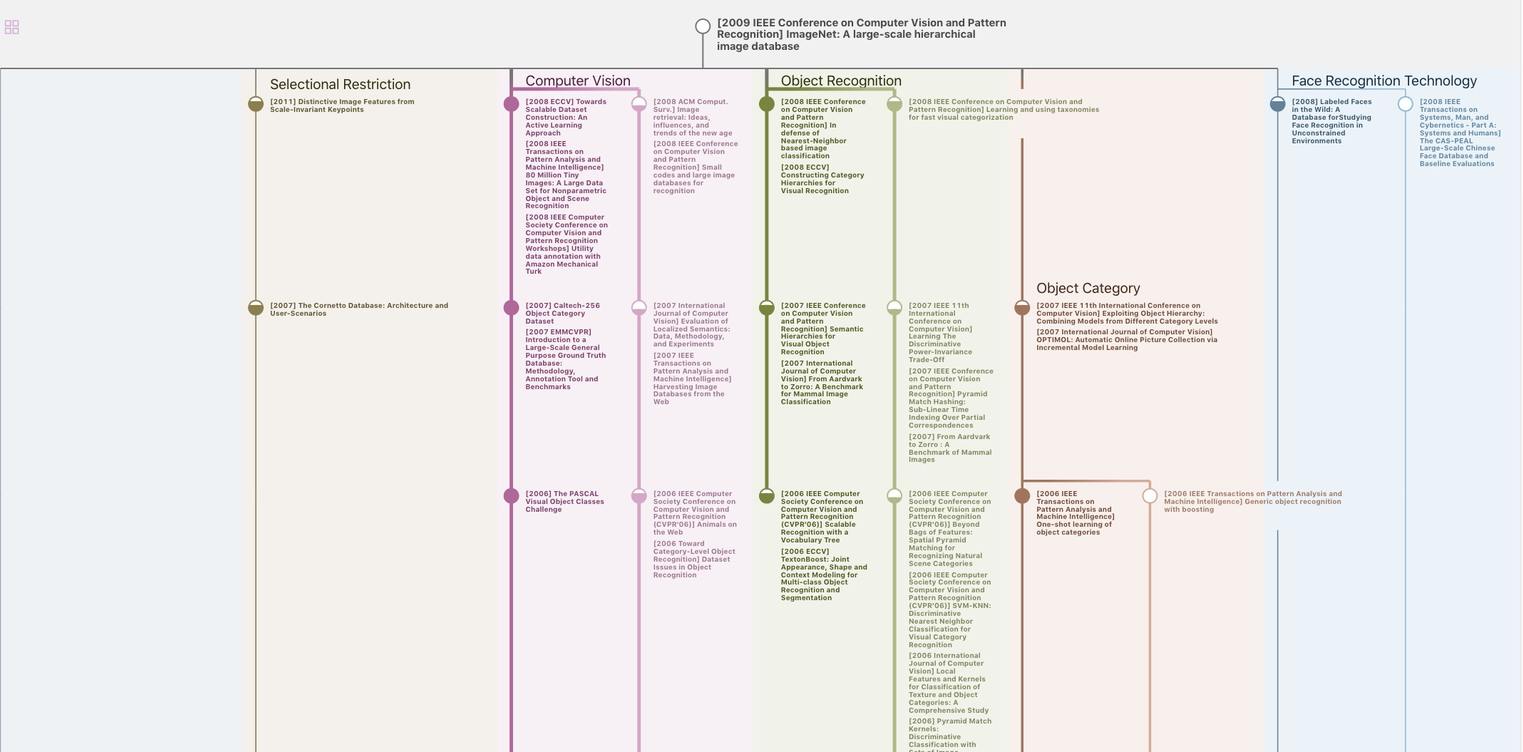
生成溯源树,研究论文发展脉络
Chat Paper
正在生成论文摘要