Evolutionary Search Via Channel Attention Based Parameter Inheritance and Stochastic Uniform Sampled Training
Computer Vision and Image Understanding(2024)
摘要
Convolutional neural networks’ performance is significantly affected by their architecture. Different algorithms have been developed to aid in automating the network structure selection. Recently, evolutionary neural architecture search (ENAS) methods have gained increasing number of attentions due to their excellent global search capability. Nevertheless, evolutionary neural architecture can be computationally expensive since improving evolution typically involves many performance evaluations. This study introduces a framework utilizing evolutionary search for a lightweight channel attention convolutional network. It leverages stochastic uniform sampled and parameter inheritance to address these challenges. The lightweight channel attention method is applied to the model to make it more capable of extracting features while using fewer parameters. In this framework, parent individuals undergo stochastic sampling and receive training repeatedly on each mini-batch of the training dataset. The strategy of parameter inheritance enables the assessment of offspring individuals’ fitness without fully training the offspring candidate network. Compared to existing methods on standard-bench (CIFAR-10, CIFAR-100, ImageNet) and real-world datasets(NEU-CLS, Chest Xray2017), our model demonstrated superior search efficiency by several orders of magnitude. Our experiments showed that Stochastic uniform sampled evolutionary Network (SUSE-Net) achieved the lowest error rate among state-of-the-art NAS models, with 2.55% for CIFAR-10, 14.8% for CIFAR-100, and 23.2% for ImageNet dataset. This results indicate that the proposed algorithm is not only more computationally efficient, but also competitive with existing methods in terms of learning performance.
更多查看译文
关键词
Deep learning,Neural architecture search,Image classification
AI 理解论文
溯源树
样例
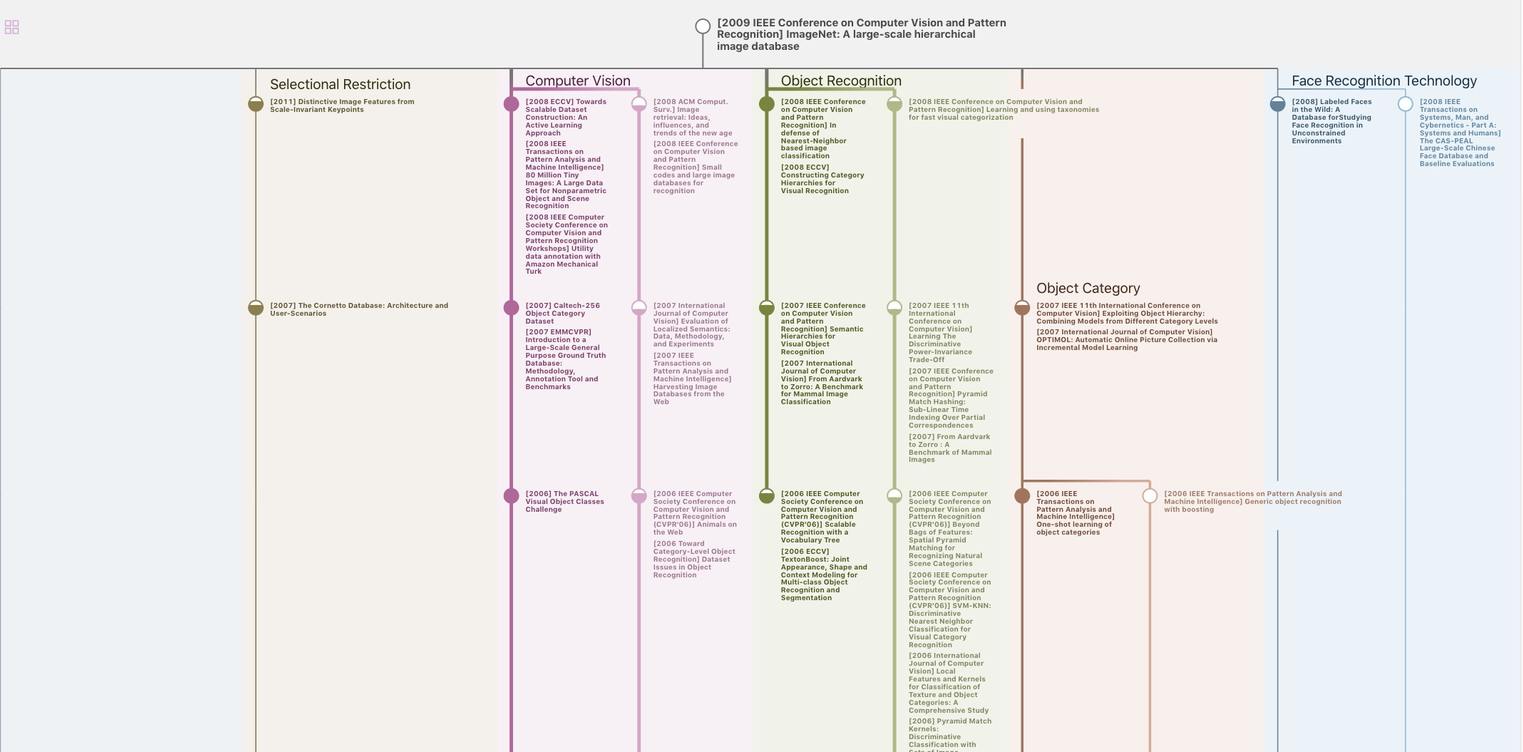
生成溯源树,研究论文发展脉络
Chat Paper
正在生成论文摘要