Integrating Confidence Calibration and Adversarial Robustness via Adversarial Calibration Entropy
Information Sciences(2024)
摘要
The vulnerability of deep neural networks to adversarial samples poses significant security concerns. Previous empirical analyses have shown that increasing adversarial robustness through adversarial training leads to models making unconfident decisions, undermining trust in model confidence scores as an accurate indication of their reliability. This raises the question: are adversarial robustness and confidence calibration mutually exclusive? In this work, we find empirically that adversarial examples mislead undefended models to make more confident mistakes during an attack and that adversarial training causes models to become more risk-averse. Further, we investigate the phenomenon of adversarial degradation from an uncertainty perspective and demonstrate that confidence and adversarial robustness can exhibit a uniform trend. To simultaneously improve the model's adversarial robustness and confidence calibration performance, we propose a novel adversarial calibration entropy to regularize the cross-entropy. Extensive experiments show that our approach increases the confidence that the model makes correct decisions and achieves adversarial robustness comparable to current state-of-the-art models.
更多查看译文
关键词
Deep neural network,Adversarial robustness,Confidence calibration,Adversarial calibration entropy
AI 理解论文
溯源树
样例
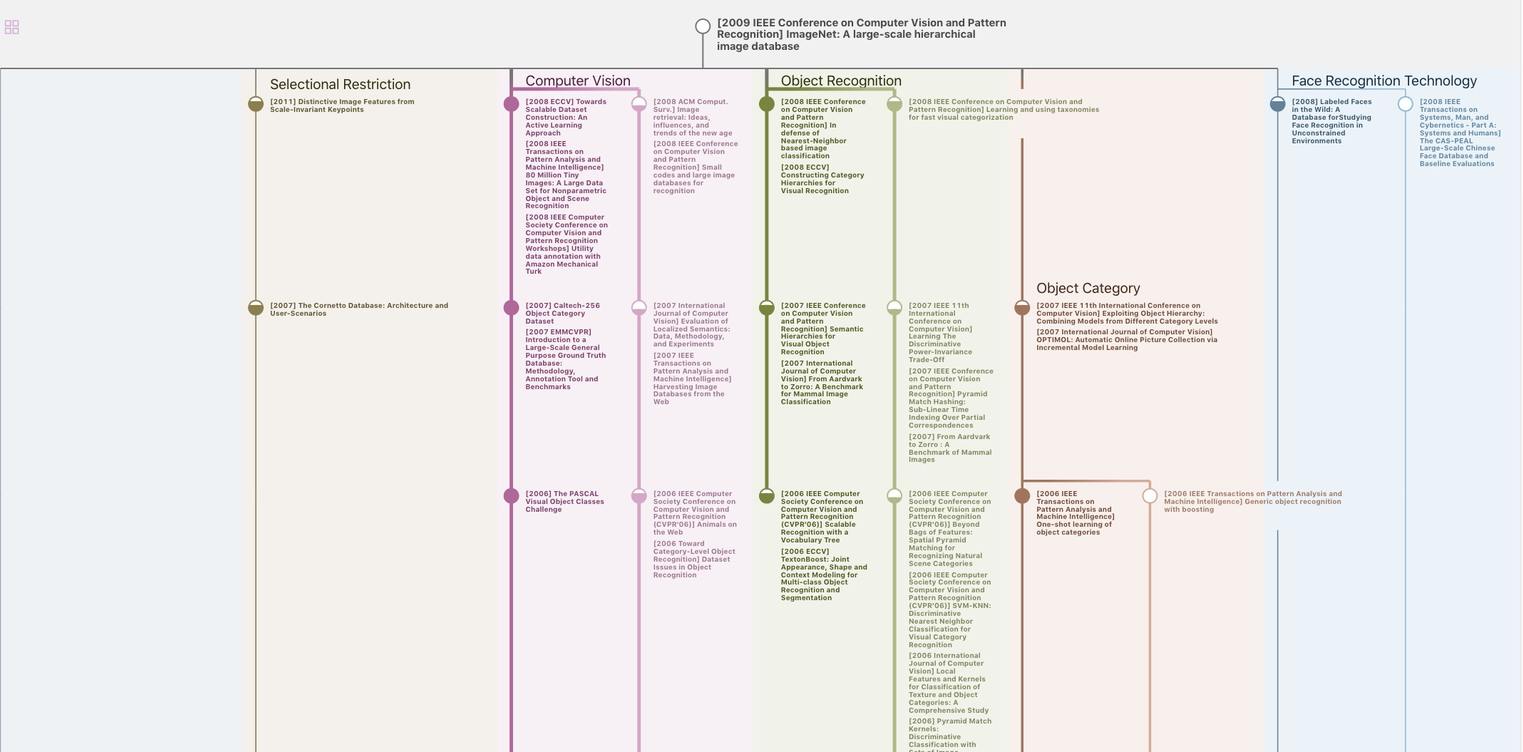
生成溯源树,研究论文发展脉络
Chat Paper
正在生成论文摘要