Explaining Answers Generated by Knowledge Graph Embeddings
International Journal of Approximate Reasoning(2024)
摘要
Completion of large-scale knowledge bases, such as DBPedia or Freebase, often relies on embedding models that turn symbolic relations into vector-based representations. Such embedding models are rather opaque to the human user. Research in interpretability has emphasized non-relational classifiers, such as deep neural networks, and has devoted less effort to opaque models extracted from relational structures, such as knowledge graphs. We introduce techniques that produce explanations, expressed as logical rules, for predictions based on the embeddings of knowledge graphs. Algorithms build explanations out of paths in an input knowledge graph, searched through contextual and heuristic cues.
更多查看译文
关键词
Knowledge graphs,Embeddings,Link prediction,Interpretability,Explainable Artificial Intelligence
AI 理解论文
溯源树
样例
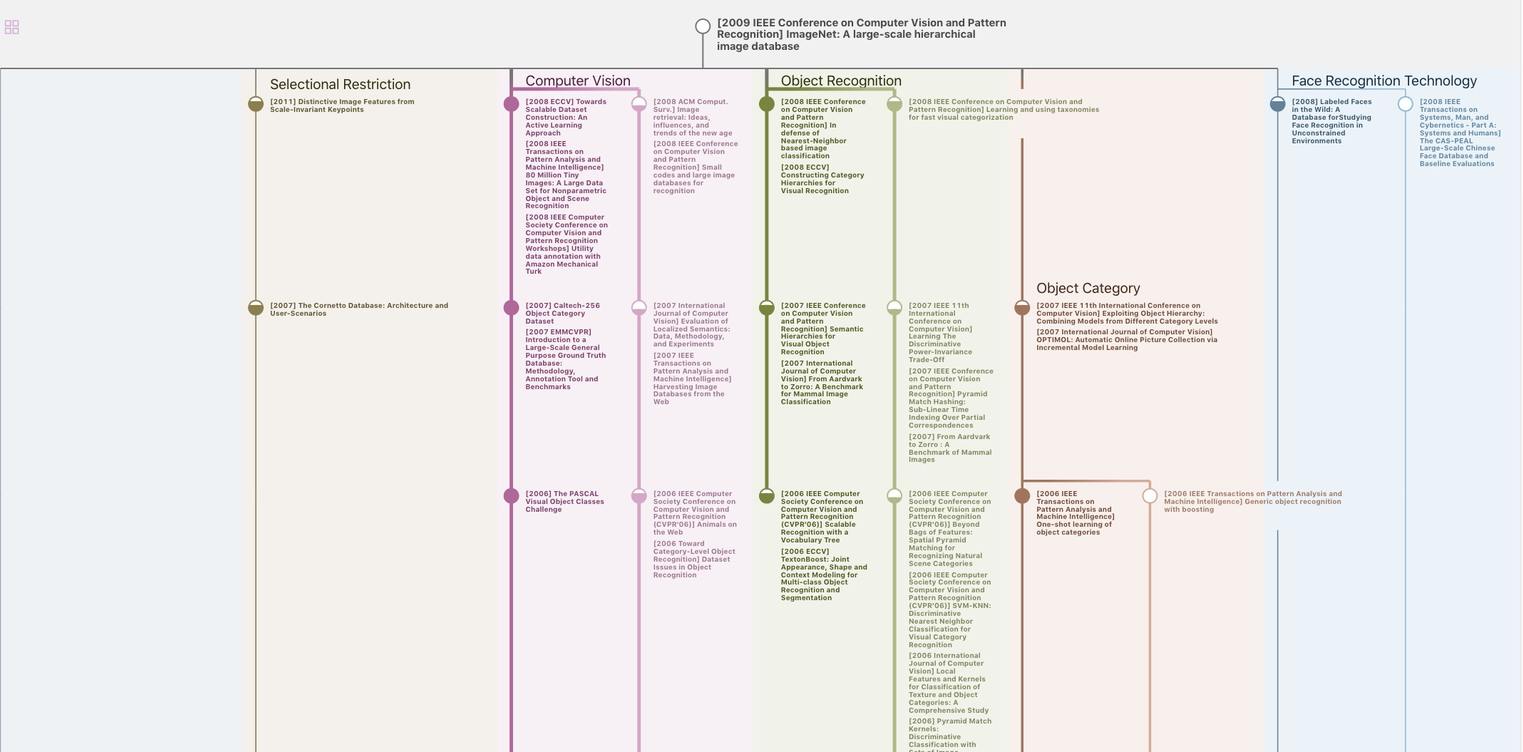
生成溯源树,研究论文发展脉络
Chat Paper
正在生成论文摘要