Information Theoretic Clustering for Coarse-grained Modeling of Non-equilibrium Gas Dynamics
Journal of Computational Physics(2024)
摘要
We present a new framework towards the objective of learning coarse-grained models based on the maximum entropy principle. We show that existing methods for assigning clusters using the maximum entropy approach are heuristic or sub-optimal. We propose a machine learning framework informed by rate-distortion theory to learn optimal cluster assignments, aiming to improve the effectiveness of the coarse-graining process. Our approach involves transforming the discrete optimization problem into a probabilistic and continuous form. Our inverse modeling approach involves the development of a fully differentiable, adjoint-driven dynamics solver for use with gradient-based optimization techniques. The entire framework is end-to-end differentiable, facilitating backward pass gradient computation to flow through the ODE solve and probabilistic coarse-graining to train a classifier. We demonstrate application in the evolution of particle quantum states in non-equilibrium conditions. The high dimensionality of these equations poses a significant challenge for efficient and accurate computations, even for seemingly simple chemical systems. Training is performed using a loss function informed by rate-distortion theory to cluster the rovibrational states of some molecule, effectively forming a reduced order model of the master equations. We also introduce variable transformations along with several ways of improving the tractability of the training process. These allow the framework to process the extremely high-dimensional discrete optimization problem successfully. Our method is general, and to demonstrate the effectiveness in a controlled setting, we apply it to a one-dimensional Gaussian source quantization problem for which an analytical solution is known, followed by the problem of isothermal relaxation of a Image 1 system with O(104) degrees of freedom.
更多查看译文
关键词
reduced order modeling,rate distortion theory,clustering,coarse graining,non-equilibrium
AI 理解论文
溯源树
样例
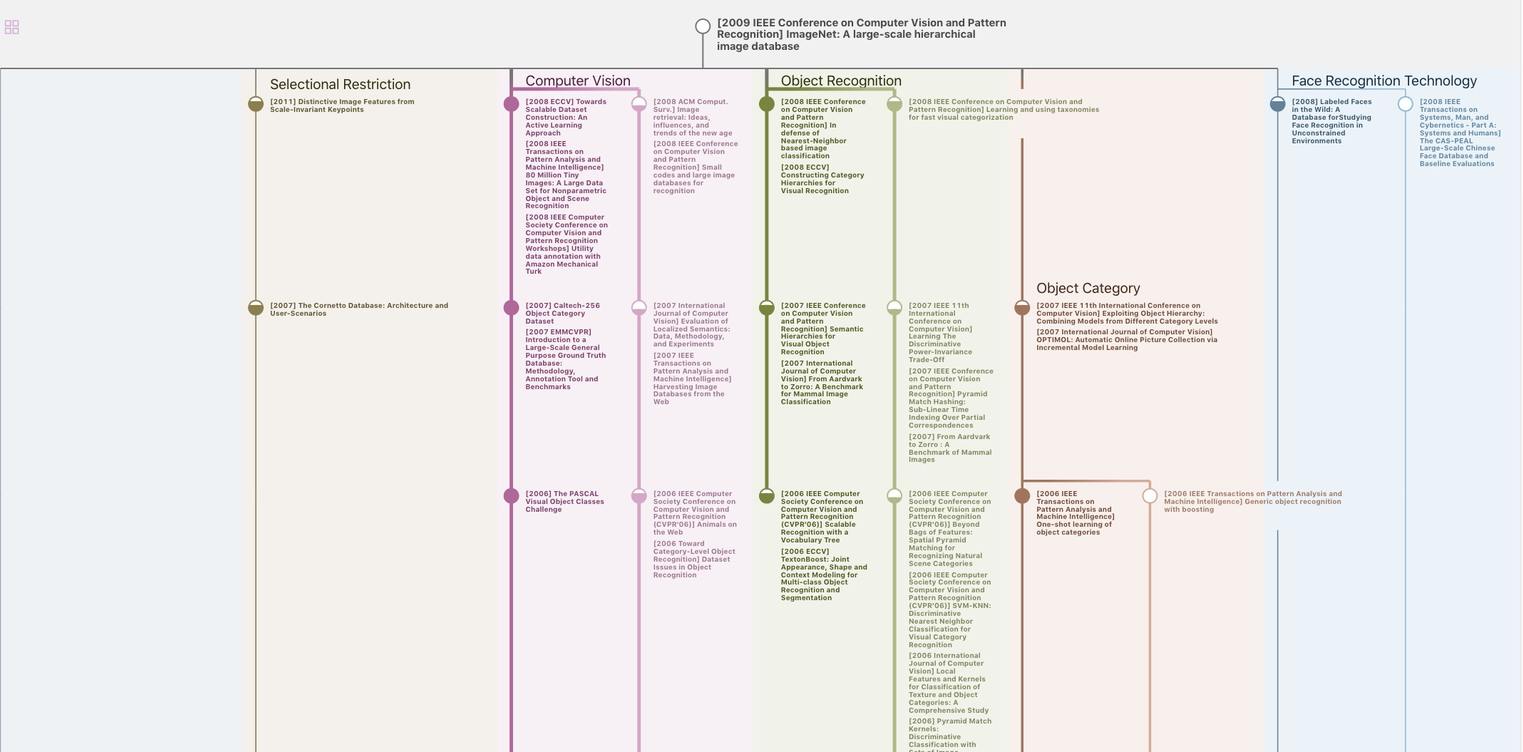
生成溯源树,研究论文发展脉络
Chat Paper
正在生成论文摘要