An effective targeted label adversarial attack on graph neural networks by strategically allocating the attack budget
Knowledge-Based Systems(2024)
摘要
Although graph neural networks (GNNs) have been extensively employed in various research fields, they are susceptible to adversarial attacks, as revealed in previous studies. Targeted label attacks, in which the victim node is anticipated to fall into a desired category, are more consistent with practical circumstances than untargeted label attacks. However, there has been relatively little research on them, and existing attackers require access to the target model and do not consider the budget allocation; the budget refers to the maximum number of perturbations permitted. Furthermore, the performance of untargeted label attacks is weakened when they are converted into targeted label attacks. From the perspective of budget allocation, we devise an effective targeted label attack (ETLA). The prior knowledge includes training labels, node features, and the graph structure. The main concept is based on the strategical allocation of a given budget from the search space and optimisation objective. For the search space, a stratified node selection strategy and an adaptive edge choice strategy are developed to capture insertable and removable candidates. The former exploits unlabelled node information through massive pseudo-labels, whereas the latter capitalises on the similarity of node features. Regarding the optimisation objective, a temperature factor is introduced into the attack loss to better direct the flipping of the candidates. After a finite number of perturbations, the final disturbed graph is fed into various target models to evaluate attack performance. Generous trials indicate that our ETLA has excellent attack capability compared with other attack techniques with the same budget.
更多查看译文
关键词
Graph neural network,Node classification,Graph structure attack,Targeted label attack
AI 理解论文
溯源树
样例
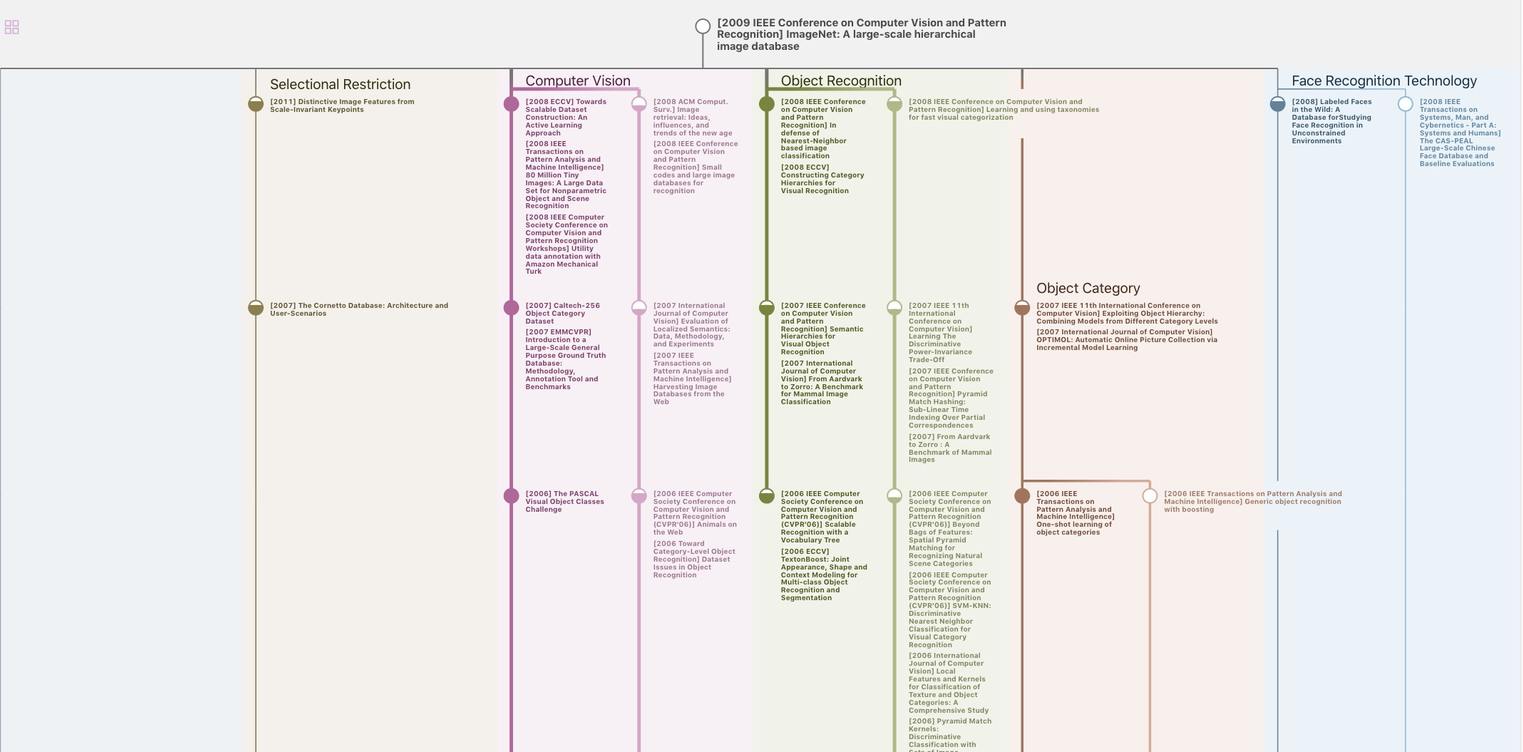
生成溯源树,研究论文发展脉络
Chat Paper
正在生成论文摘要