SeqOAE: Deep sequence-to-sequence orthogonal auto-encoder for time-series forecasting under variable population sizes
Reliability Engineering & System Safety(2024)
摘要
This paper presents a novel approach to modeling non-linear time-series data in which the population size changes over observation time. This is a common phenomenon when forecasting the time-series of an ongoing study/operation. The goal is to make predictions about the mature time-series at the end of the study/operation given the immature time-series at an early stage of the study/operation. Existing time-series models often ignore this phenomenon by focusing on a fixed population size or a single unit of interest. This paper proposes a sequence-to-sequence deep learning model (SeqOAE) that captures the change in the time-series as a function of observation time. It learns an orthogonal latent feature space for robust long-term temporal forecasting. SeqOAE is non-parametric in time, which allows it to capture non-trivial and non-linear temporal patterns. SeqOAE enables forecasting performance behavior, which is paramount for reliability engineering applications. The model performance is validated on a reliability engineering case study using an extensive automotive warranty dataset with over 12,500 components from millions of vehicles.
更多查看译文
关键词
Auto-encoder,Deep learning,Forecasting,Time-series,Orthogonality,Machine learning,Reliability,Warranty
AI 理解论文
溯源树
样例
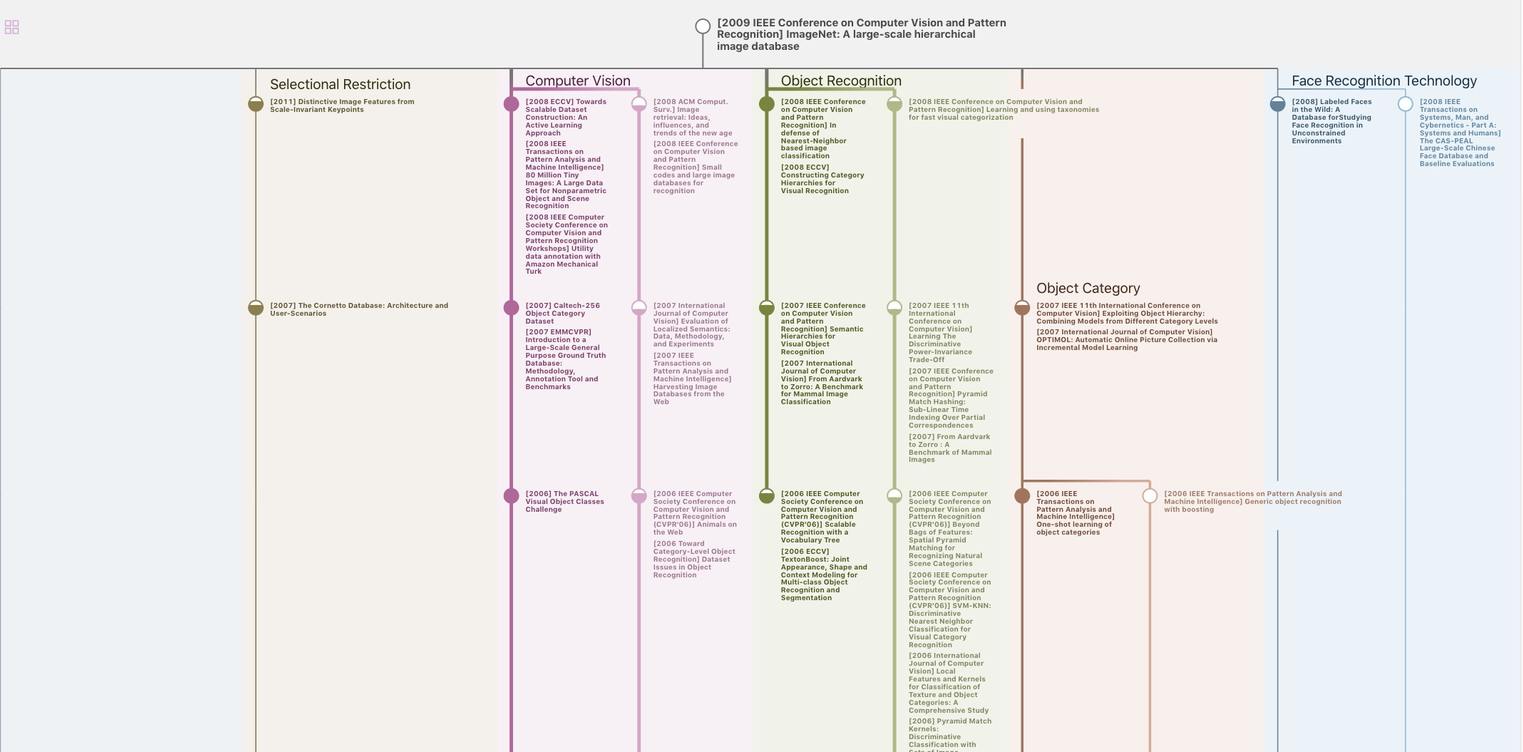
生成溯源树,研究论文发展脉络
Chat Paper
正在生成论文摘要